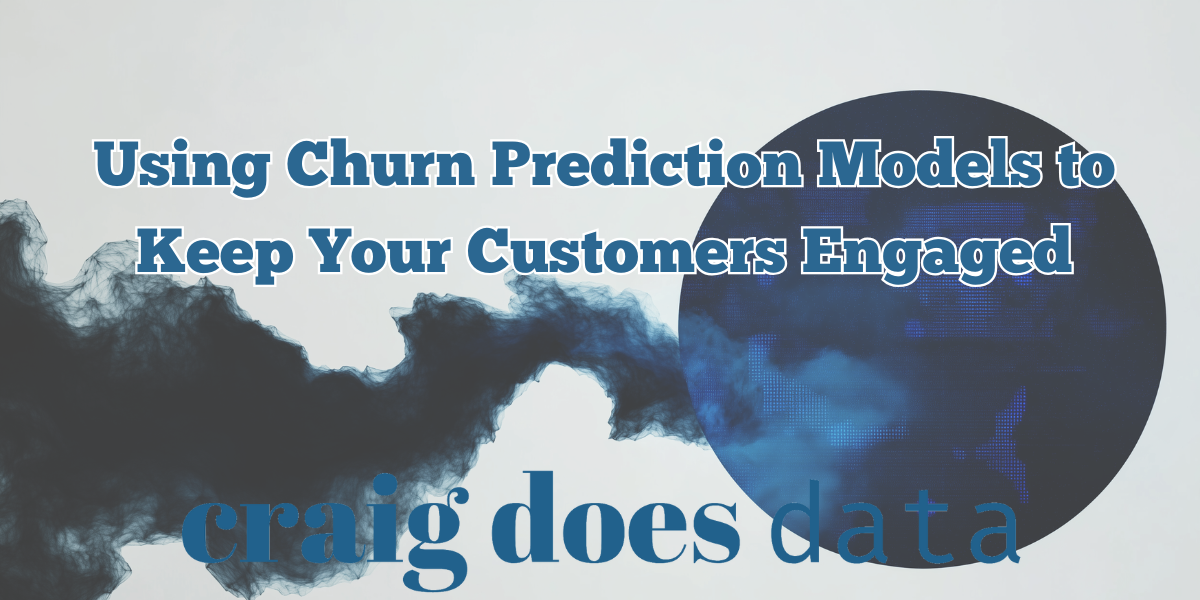
Using Churn Prediction Models to Keep Your Customers Engaged
Introduction
Customer churn is one of the most critical challenges that modern businesses face across all sectors. Whether you operate a subscription-based service, an e-commerce platform, or a traditional brick-and-mortar store, the cost of losing a customer and having to replace them with a new one can be significant. Churn does not only signify a drop in revenue, but also a signal that your product or service failed to meet ongoing customer needs. When clients stop engaging with your brand—ceasing to make purchases, unsubscribing from your platform, or simply becoming inactive—it is a direct reflection of diminished value or dissatisfaction in some aspect of the customer journey.
In today’s competitive environment, customer engagement stands out as one of the most powerful tools for retention. Businesses that proactively keep their customers interested—whether through meaningful interactions, personalized offers, or timely support—often see improved brand loyalty and repeat purchases. Engagement is no longer just an abstract concept; it’s a quantifiable metric that correlates strongly with retention, revenue growth, and long-term sustainability.
This article serves as a comprehensive guide to tackling churn by leveraging churn prediction models. We will explore how advanced analytics and machine learning algorithms can be utilized to forecast which customers might leave and, most importantly, how you can proactively intervene before it’s too late. From understanding the fundamentals of churn to implementing advanced predictive models and engagement strategies, you will learn the ins and outs of keeping your customers satisfied and loyal.
Below is the roadmap for what we will discuss:
- Understanding Customer Churn: Why churn occurs and why it matters.
- The Importance of Customer Engagement: Connecting engagement metrics to retention.
- Introduction to Churn Prediction Models: The role of data and machine learning.
- How Churn Prediction Models Work: Building, training, and evaluating churn models.
- Implementing Churn Prediction in Business: Practical steps and cross-functional teamwork.
- Strategies for Engagement: Leveraging model outputs to tailor customer interactions.
- Overcoming Common Challenges: Dealing with data issues, complexity, and stakeholder alignment.
- Future Outlook: Emerging trends and technologies shaping churn prediction and engagement.
By the end of this article, you will have both a theoretical and practical understanding of how to utilize churn prediction models as a cornerstone of a robust customer engagement strategy. Let’s dive into the details and learn how to keep your customers invested in your brand for the long run.
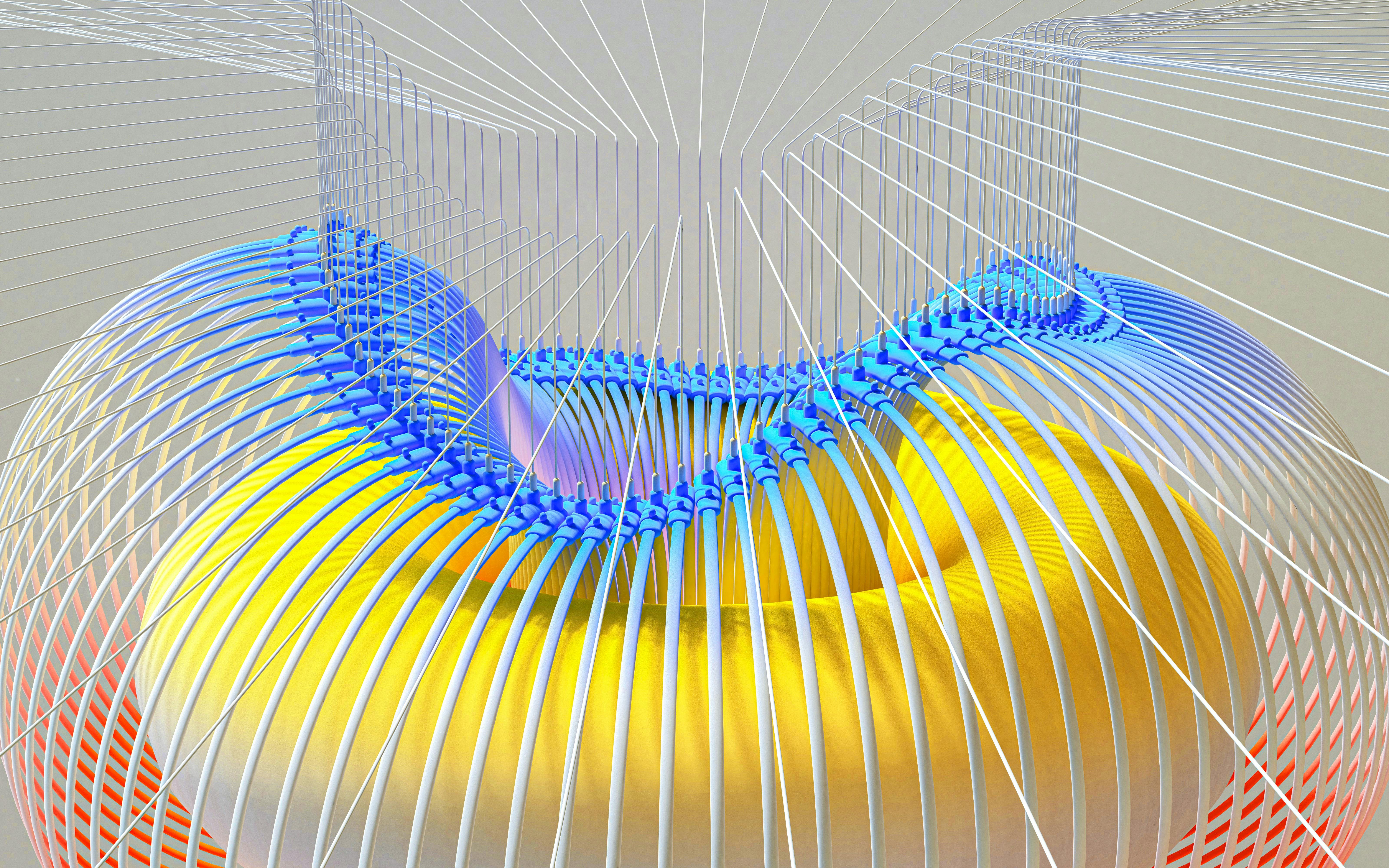
1. Understanding Customer Churn
Customer churn, simply put, is the rate at which customers stop doing business with a company. This could manifest as unsubscribing from a service, ceasing to purchase products, or not renewing a membership. In subscription models, churn is often measured monthly or annually, depending on billing cycles. In retail or traditional business contexts, churn can show up as declining purchase frequency or a failure to return over a specified period. Regardless of the specific manifestation, churn serves as a key performance indicator that highlights potential issues in product market fit, customer satisfaction, or overall value delivery.
The significance of customer churn is profound across multiple industries. In the telecommunication sector, for example, where competition is fierce and customers have myriad options, high churn rates can drastically impact revenue streams. In subscription-based online services like video streaming platforms, music providers, or software-as-a-service (SaaS) products, churn directly translates to lost monthly recurring revenue. Meanwhile, retail and e-commerce businesses experience churn when customers no longer choose to shop with them, often swayed by more competitive pricing, better customer service, or more appealing brand experiences elsewhere.
Common indicators of churn include significant drops in usage or engagement metrics. For instance, a user who consistently visited a website daily but has not logged in for two weeks may be at risk of churning. Other telltale signs can be negative customer feedback, unresolved support tickets, or decreased spending behavior over time. Understanding these indicators is crucial; the earlier you spot them, the more time you have to intervene and potentially salvage the relationship.
Why does churn matter so much? First, acquiring new customers is usually more expensive than retaining existing ones. Marketing campaigns, advertising spend, and sales efforts needed to attract new patrons often outweigh the investment required to keep current clients happy. Secondly, high churn rates can indicate systemic problems within your product or service—perhaps your pricing is not competitive, or your user experience is underwhelming. Third, investors and stakeholders closely watch churn rates as an indicator of a company’s long-term health and potential. A rapidly churning customer base can cast doubt on the sustainability and profitability of the entire business model.
From a strategic perspective, understanding churn allows businesses to take proactive measures. If you can diagnose why customers are leaving, you can tailor strategies to address those pain points—whether that involves improving user experience, adjusting pricing, or enhancing customer support. Moreover, identifying which customers are most likely to churn enables you to optimize resource allocation, focusing retention efforts where they are most needed.
In the sections that follow, we’ll delve deeper into how effective customer engagement and predictive analytics can serve as powerful tools in reducing churn. By embracing the idea that churn is both a symptom and an opportunity, businesses can transform their approach to customer retention from a reactive cost center to a proactive, strategic growth function.
2. The Importance of Customer Engagement
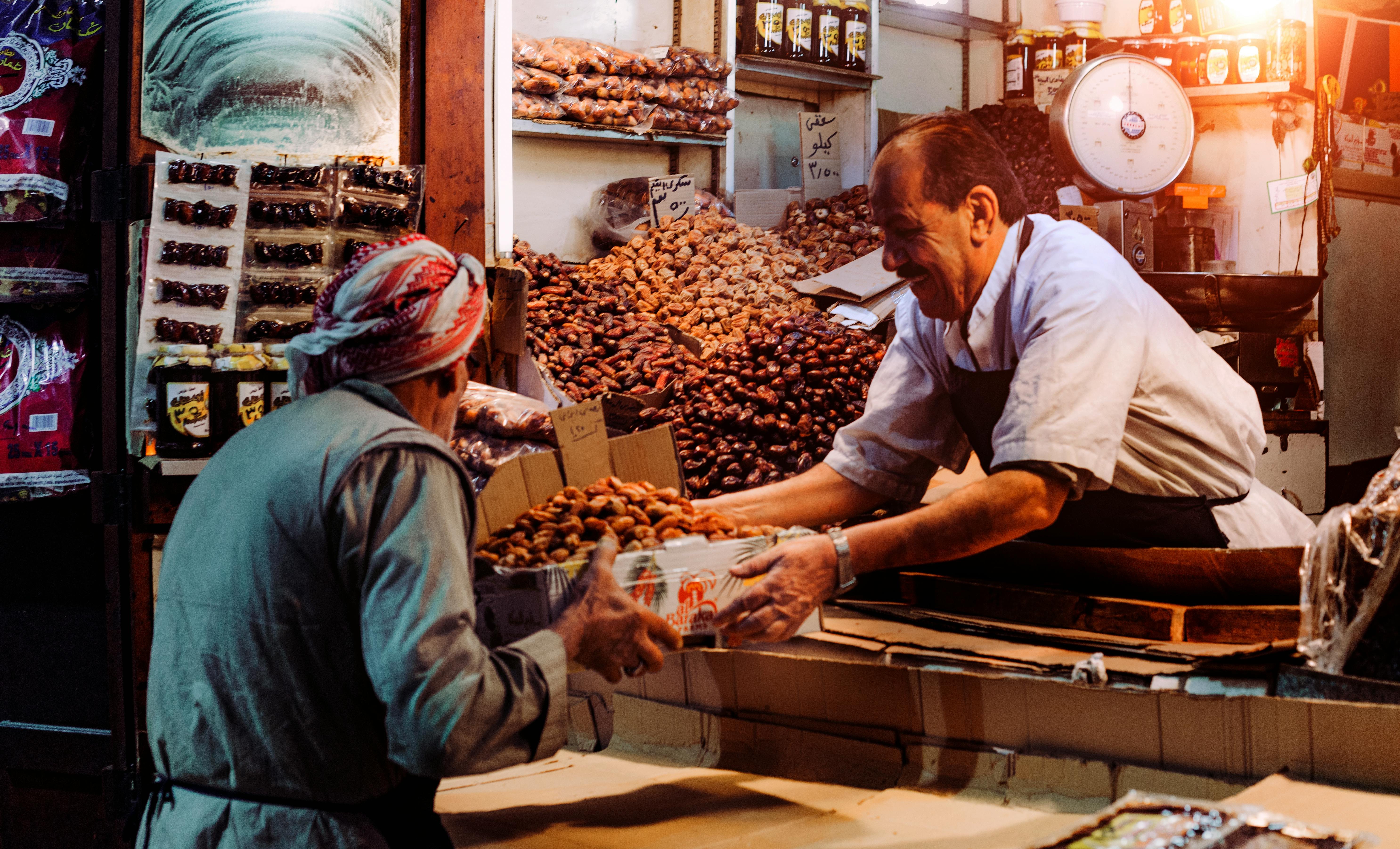
Customer engagement refers to the depth of the relationship between a business and its customers, characterized by meaningful interactions at various touchpoints. These touchpoints could be as diverse as a mobile app interface, a social media post, an email campaign, or a real-life store visit. Highly engaged customers are more likely to continue purchasing products and services, provide valuable feedback, and even advocate for the brand within their social circles. In a marketplace saturated with competing offerings, effective engagement strategies can set a brand apart and foster loyalty.
The link between customer engagement and churn has been well documented across industries. Multiple studies point to a strong correlation between high engagement and reduced churn rates. For instance, a SaaS company might find that its daily active users are significantly less likely to cancel their subscriptions than users who log in infrequently. In e-commerce, engaged customers who interact with promotional campaigns, read product reviews, and have personalized shopping experiences often exhibit higher repurchase rates. Even in business-to-business settings, clients who consistently engage with a software platform or a service representative tend to renew their contracts more frequently.
One reason engagement is so powerful is because it fosters an emotional connection with the brand. When customers feel recognized, appreciated, and understood, they are less inclined to explore competitors. Engagement also encourages the accumulation of small “wins”—positive interactions that build up a sense of satisfaction over time. Whether it's a well-timed discount, an exclusive offer, or consistent communication from a dedicated account manager, these touchpoints reinforce the idea that staying with the brand is beneficial and worthwhile.
Another key benefit of robust engagement is that it enhances the customer’s lifetime value (CLV). A buyer who remains loyal for years, continually making purchases or renewing subscriptions, will generate far more revenue than a new customer who only makes a single purchase and then churns. This increase in lifetime value can justify strategic investments in personalization, rewards programs, customer support infrastructure, and community-building efforts.
Beyond financial metrics, an engaged customer base can serve as a vital source of organic marketing. These satisfied customers often leave positive reviews, share their experiences on social media, and become vocal advocates for your brand. Word-of-mouth referrals are among the most effective forms of marketing, as people tend to trust recommendations from friends, family, or colleagues more than traditional advertising. Consequently, engagement fuels a virtuous cycle: engaged customers help attract more like-minded customers, which in turn grows revenue and brand awareness.
As we move into the practical aspects of using churn prediction models, keep in mind that all these efforts ultimately hinge on this fundamental principle: engagement is the cornerstone of retention. Predictive analytics will reveal who is at risk of leaving, but well-crafted engagement strategies—tailored to individual customer segments—will determine whether at-risk customers choose to stay.
3. Introduction to Churn Prediction Models
Churn prediction models are analytical frameworks designed to identify which customers are most likely to discontinue their relationship with a business. They function by analyzing a wide array of data points—from transactional behavior to demographic profiles—to unearth patterns that correlate with churn. The underlying logic is straightforward: customers who exhibit certain behaviors, or who fall within specific demographic segments, are statistically more likely to stop using your services, unless an intervention takes place.
Typically, these models rely on three major types of data:
- Transactional Data: This includes past purchase history, subscription renewals, payment methods, and average order value. By examining how customers buy, businesses can detect early warning signs such as a decrease in purchase frequency.
- Behavioral Data: This encompasses website visits, app usage patterns, clicks, time spent on the platform, and customer support interactions. For instance, a SaaS provider might track login frequency, feature usage, and help-desk tickets to gauge satisfaction levels.
- Demographic Data: Age, location, income level, and other demographic variables can also influence churn risk. While not always deterministic, these factors often reveal segments that behave differently and may require tailored engagement strategies.
Over the years, the rise of machine learning and big data analytics has dramatically improved the accuracy of churn prediction models. In earlier phases, businesses relied on relatively basic statistical techniques—like logistic regression—using a handful of variables to predict churn. While these methods are still valuable, modern analytics often employ more sophisticated algorithms such as random forests, gradient boosting, neural networks, and ensemble methods. These techniques can handle massive datasets and detect complex, non-linear relationships between variables and churn outcomes.
Machine learning models excel at recognizing hidden patterns that might be missed by conventional analytics. For example, a neural network might detect that a certain niche segment of customers, who log in once a week and make sporadic low-value purchases, are on the verge of cancelling. Yet, these same users might not be flagged by a simpler model that looks solely at overall purchase frequency or total spending.
Another advantage of churn prediction models is their capability for continuous learning. As fresh data flows in—new purchases, updated customer feedback, the introduction of new products, etc.—the model can be retrained or updated to reflect the latest trends and behaviors. This agility allows businesses to keep a real-time pulse on which customers are on the fence, enabling swift interventions that might include personalized email campaigns, targeted promotions, or proactive customer service outreach.
In subsequent sections, we’ll explore how these models work in detail and the steps required to integrate them into your existing operations. Regardless of the specific machine learning algorithm you choose, the unifying theme remains the same: by accurately forecasting who is likely to leave, you gain a pivotal opportunity to shift at-risk customers into long-term advocates for your brand.
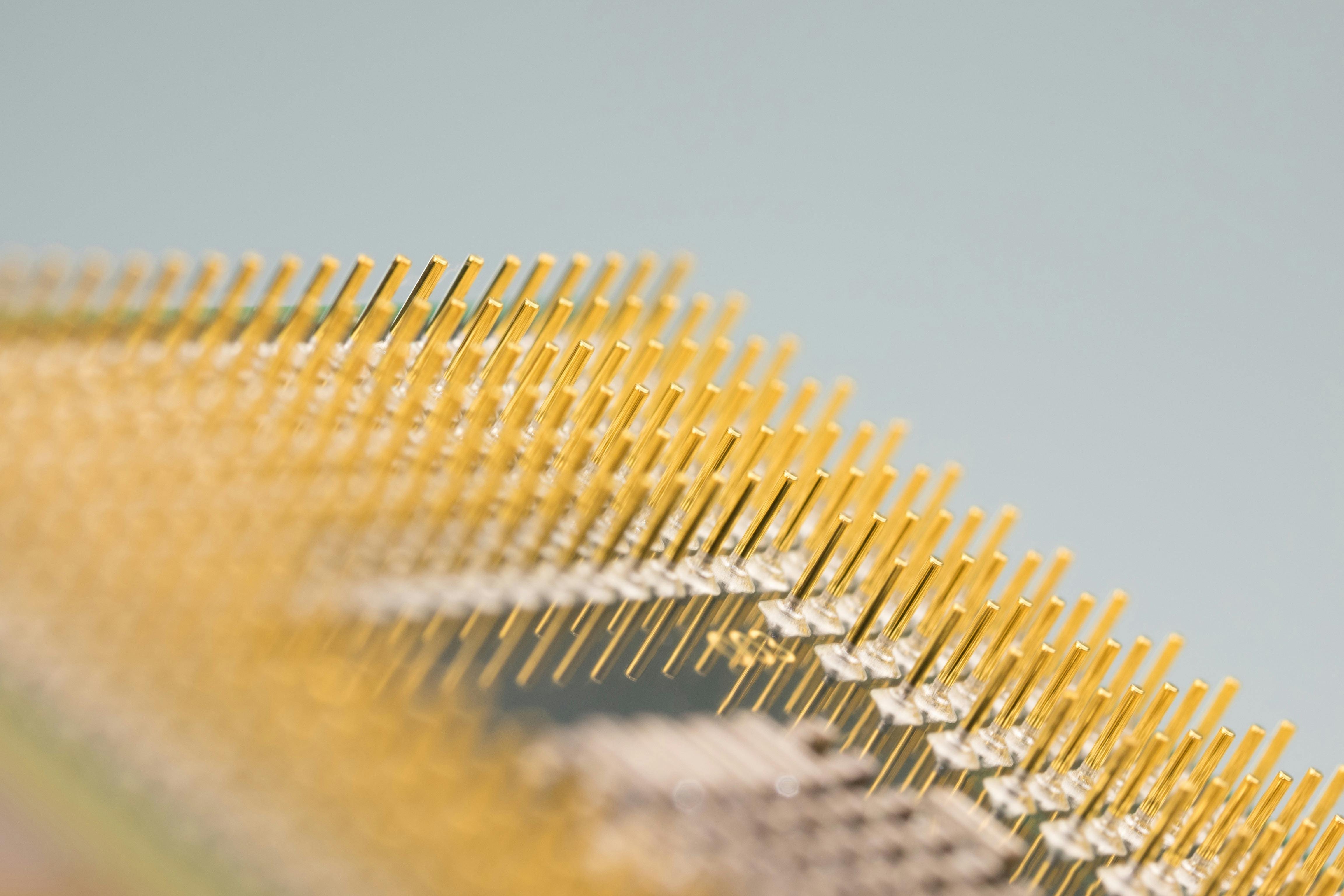
4. How Churn Prediction Models Work
Building an effective churn prediction model is a multi-step process that typically follows a logical sequence. While there are various methodologies—such as CRISP-DM (Cross-Industry Standard Process for Data Mining)—the core steps remain relatively consistent across different frameworks. Below is a high-level breakdown of how these models are created and refined.
Data Collection and Preprocessing
The first step is to gather comprehensive data relevant to customer activity. This may include transactional records (purchases, subscriptions), behavioral metrics (logins, page views), and demographic information. Because churn can manifest differently depending on the type of customer, it’s crucial to include as many relevant data points as possible. Once collected, the data needs to be cleansed of inaccuracies, duplicates, and missing values. Preprocessing might also involve normalizing or standardizing numerical features to ensure they are on a similar scale.
Feature Selection and Engineering
Feature selection involves identifying which variables are most predictive of churn. This can be guided by domain expertise (e.g., marketing teams might highlight that email open rates are very indicative of customer interest) or by algorithmic methods (like feature importance scores in random forests). Feature engineering takes this a step further by creating new variables from existing data. For instance, you could calculate “average spend per visit” or “time since last purchase” to capture more nuanced aspects of customer behavior. Well-engineered features often have a significant impact on model accuracy.
Model Training and Evaluation
After selecting and engineering features, the next phase involves training a machine learning model to distinguish between customers who churn and those who do not. Common algorithms include:
- Logistic Regression: A traditional statistical method that estimates churn probability based on input features. It’s easy to interpret but may not capture complex interactions.
- Decision Trees: Tree-based models that split data into subsets based on specific feature thresholds. These are intuitive to interpret and can handle non-linear patterns.
- Random Forests and Gradient Boosting: Ensemble methods that build multiple trees to improve predictive performance. They’re highly accurate but can be somewhat opaque in interpretation.
- Neural Networks: These models are particularly good at capturing complex relationships in large datasets. However, they may require more computational resources and can be harder to interpret.
Once trained, the model’s performance is evaluated using metrics such as accuracy, precision, recall, and the Area Under the Receiver Operating Characteristic (ROC) curve (AUC). The choice of metric depends on the business context. For instance, if the cost of falsely labeling a customer as a non-churner is very high, recall or AUC might be more important than raw accuracy.
Deployment and Monitoring
The final step involves deploying the model in a production environment, making its predictions accessible to marketing, sales, and customer success teams. Modern platforms enable real-time or near-real-time scoring, allowing teams to promptly identify at-risk customers. Ongoing monitoring is essential to ensure the model remains accurate as market conditions or customer behaviors evolve. Regular retraining and recalibration of the model are typical, especially when new features are introduced or substantial changes occur in the product lineup.
By meticulously following these steps, companies can develop churn prediction models that serve as reliable “warning systems.” These systems help your organization detect early signs of customer dissatisfaction or disengagement, laying the groundwork for targeted interventions that can turn would-be defectors into loyal patrons.
5. Implementing Churn Prediction Models in Your Business
While the technical aspects of building a churn prediction model are critical, successful implementation also requires organizational alignment and practical integration into day-to-day workflows. A well-built model that sits unused on a data scientist’s laptop provides no real value. Instead, the insights must be disseminated across teams, from marketing and sales to customer support and product development.
Below is a step-by-step guide to seamlessly integrating churn prediction models into your business operations:
Step 1: Define Clear Objectives and KPIs
Before rolling out a predictive model, specify what success looks like. Are you aiming to reduce churn by a certain percentage within a set timeframe? Are you looking to target specific customer segments (e.g., high-value subscribers)? Aligning on KPIs ensures everyone from the executive team to frontline employees understands the goals and the intended impact.
Step 2: Collaborate Across Functional Teams
Effective churn prediction often requires input and buy-in from multiple departments. Data engineers or IT personnel handle the extraction and processing of data. Marketing teams contribute domain expertise on campaign targeting and customer touchpoints. Sales and customer success teams manage direct interactions with clients based on risk scores. Facilitate regular meetings to share insights, address challenges, and ensure everyone is aligned on strategy.
Step 3: Choose Tools and Platforms Wisely
The choice of technology stack can significantly influence the ease and speed of implementation. Many businesses opt for open-source libraries like scikit-learn in Python or Spark MLlib for large-scale processing. Alternatively, numerous commercial platforms and cloud services (such as AWS Sagemaker, Google Cloud AI, or Azure Machine Learning) offer end-to-end solutions. Consider factors like scalability, cost, existing in-house expertise, and integration capabilities with your CRM or marketing automation tools.
Step 4: Integrate with CRM and Automation Systems
For churn prediction to be truly effective, the outputs should feed directly into the systems your teams already use. For instance, if the model flags a cohort of customers as high-risk, that information should automatically populate your CRM system (Salesforce, HubSpot, etc.), prompting account managers to take immediate action. Marketing automation platforms can then deliver targeted emails or in-app messages based on these risk scores.
Step 5: Pilot Programs and Iterative Improvement
Start with a pilot program targeting a subset of customers or a specific product line. Track the outcomes of interventions, gather feedback, and refine your model parameters and engagement strategies accordingly. Iterative improvement is key; even the most sophisticated models require fine-tuning to account for shifting market dynamics and evolving customer behaviors.
By following these steps, businesses can turn the theoretical promise of churn prediction into tangible gains in retention and revenue. The next sections will delve into how to use these insights in practical engagement strategies and what challenges you might face along the way.
6. Strategies to Keep Customers Engaged Using Churn Predictions
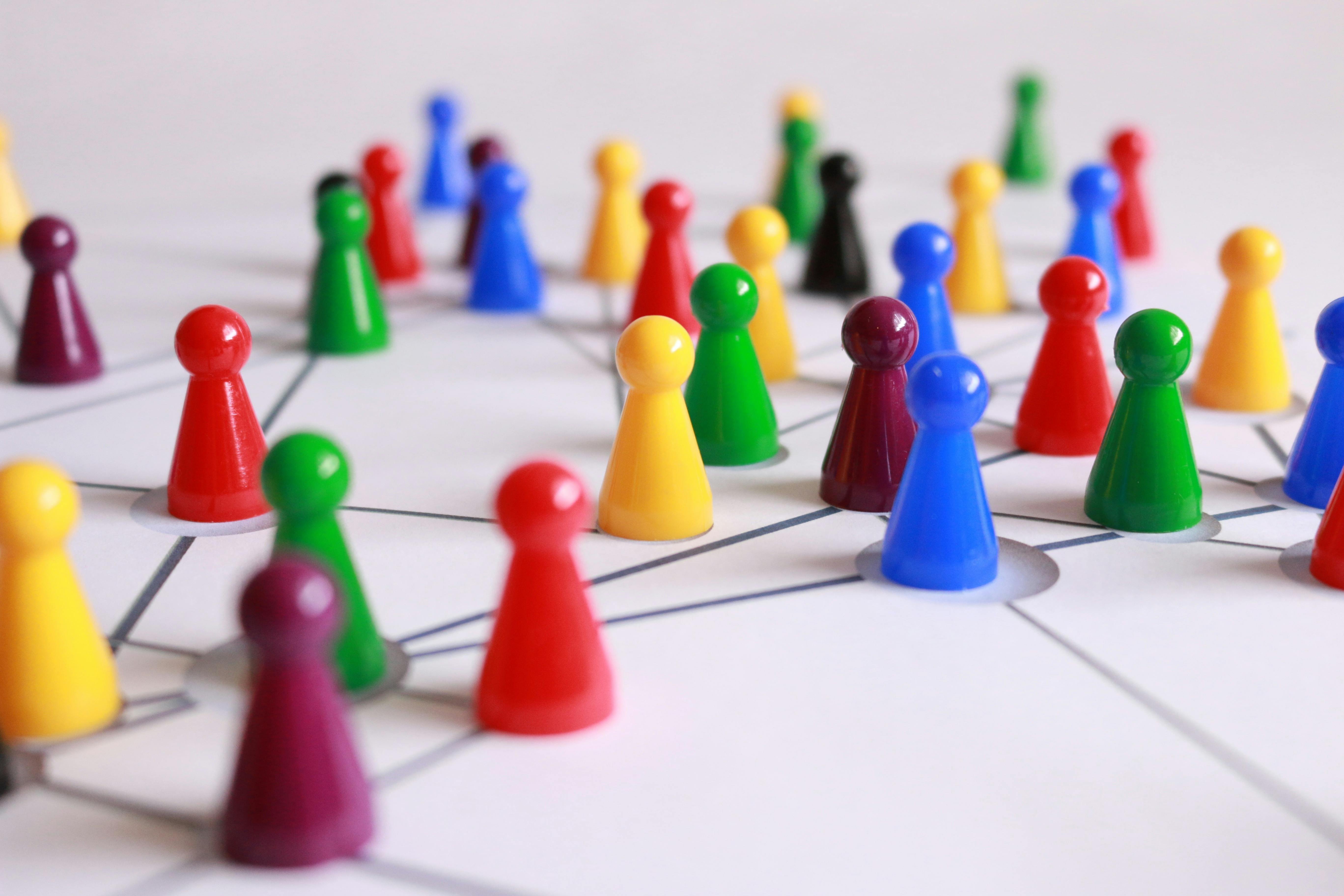
Once a churn prediction model identifies customers at risk of leaving, the question becomes: what next? The ultimate goal is to re-engage these customers and remind them of the value your business provides. Different segments of at-risk customers often require different approaches, making personalization a central component of effective retention strategies.
Below are some actionable strategies that leverage churn predictions to keep customers engaged:
1. Personalized Marketing Campaigns
Use churn risk scores to tailor your marketing messages. Customers flagged as high-risk might receive a well-timed discount or a personalized note highlighting relevant features or products. By contrast, a medium-risk segment might be best served by educational content that reinforces the value of your offerings. The key is relevance: a promotion or message should resonate with the specific concerns or usage patterns of that customer group.
2. Enhanced Customer Support
A common driver of churn is poor customer service or unresolved issues. When your model predicts that certain customers may be on the brink of leaving, proactively reach out with personalized support. This could include dedicated account managers, priority phone or chat assistance, or even on-site visits in a B2B context. Demonstrating that you are willing to invest resources into resolving their concerns can significantly boost loyalty and satisfaction.
3. Loyalty and Reward Programs
Rewarding continued engagement is a powerful retention tool. Consider implementing point-based systems, tiered memberships, or exclusive access to new products for loyal customers. If the churn model suggests certain high-value customers are at risk, offering a loyalty incentive, such as bonus rewards or unique experiences, can serve as a strong motivator to stay connected with your brand.
4. Tailored Product Recommendations
Many customers churn simply because they no longer see how a product or service fits their evolving needs. Personalized recommendations—based on browsing data, past purchases, or known preferences—can rekindle interest. For instance, an e-commerce retailer can suggest items that complement a previous purchase. A SaaS provider might highlight new software modules that better align with a user’s changing workflow.
5. Targeted Education and Onboarding
In some cases, especially with complex products or services, churn can be attributed to a lack of understanding or underutilization of features. Providing targeted onboarding sessions, video tutorials, or educational webinars can help customers discover value they might have missed. These resources not only boost user competence but also reaffirm the company’s commitment to customer success.
Many of these strategies have been successfully employed by industry leaders. For instance, streaming services like Netflix and music platforms like Spotify use predictive analytics to suggest content and pricing plans that align with user preferences, thus keeping churn rates relatively low. E-commerce giants like Amazon rely heavily on personalized recommendations and loyalty initiatives (e.g., Prime membership) to maintain engagement. By studying these real-world examples, businesses of all sizes can glean insights into how best to integrate churn predictions into their engagement tactics.
7. Overcoming Challenges in Churn Prediction and Engagement
Despite its proven benefits, implementing churn prediction models and associated engagement strategies is not without hurdles. From data availability to organizational roadblocks, businesses must be prepared to tackle a variety of challenges head-on. Below are some of the most common issues and how to address them.
Data Quality and Integration
One of the biggest obstacles is obtaining high-quality, unified data. Many organizations store customer information in disparate systems—CRM platforms, billing databases, marketing tools—making it difficult to build a comprehensive view of each customer. Inconsistent data formats, missing values, and duplicates can lead to inaccurate model predictions. To address this, investing in data warehousing or data lake solutions that centralize information is essential. Regular data cleaning, de-duplication efforts, and standardization protocols ensure that your model is trained on accurate inputs.
Model Complexity and Interpretability
As models become more sophisticated—think deep learning or complex ensemble methods—they also become more challenging to interpret. Stakeholders may be reluctant to trust a system they don’t understand. Tools like SHAP (SHapley Additive exPlanations) and LIME (Local Interpretable Model-Agnostic Explanations) can help by shedding light on how each feature contributes to a prediction. Providing interpretable insights, rather than black-box outputs, builds trust among decision-makers and encourages adoption.
Organizational Silos and Resistance
Deploying churn prediction often requires collaboration across departments—data science, IT, marketing, sales, and customer success. Silos can slow down implementation and result in misaligned objectives. Overcoming this challenge requires clear leadership support and cross-functional teams with shared accountability. Facilitating regular communication, setting common KPIs, and celebrating quick wins can help break down resistance and foster a culture of collective ownership.
Privacy and Compliance Concerns
Collecting and analyzing customer data raises valid privacy considerations. Regulations like GDPR (General Data Protection Regulation) in the EU and CCPA (California Consumer Privacy Act) in the U.S. specify how personal data should be stored and used. Ensuring that your churn prediction initiatives adhere to these guidelines is non-negotiable. Incorporate “privacy by design” principles into your data collection processes and maintain transparent communication with customers about how their data is employed.
Maintaining Model Relevance Over Time
Customer behaviors and market conditions are not static; they evolve. A model that accurately predicts churn today might become obsolete months down the line if you fail to update it with current data. Ongoing monitoring, retraining, and validation are crucial. Allocate resources for periodic model refreshes and consider establishing a pipeline that automatically retrains models as new data becomes available.
By proactively addressing these challenges, businesses can avoid common pitfalls and fully capitalize on the potential of churn prediction. No approach is perfect from day one, but a commitment to iterative improvement and cross-functional collaboration will help ensure that your churn initiatives yield tangible, long-lasting results.
8. The Future of Churn Prediction and Customer Engagement
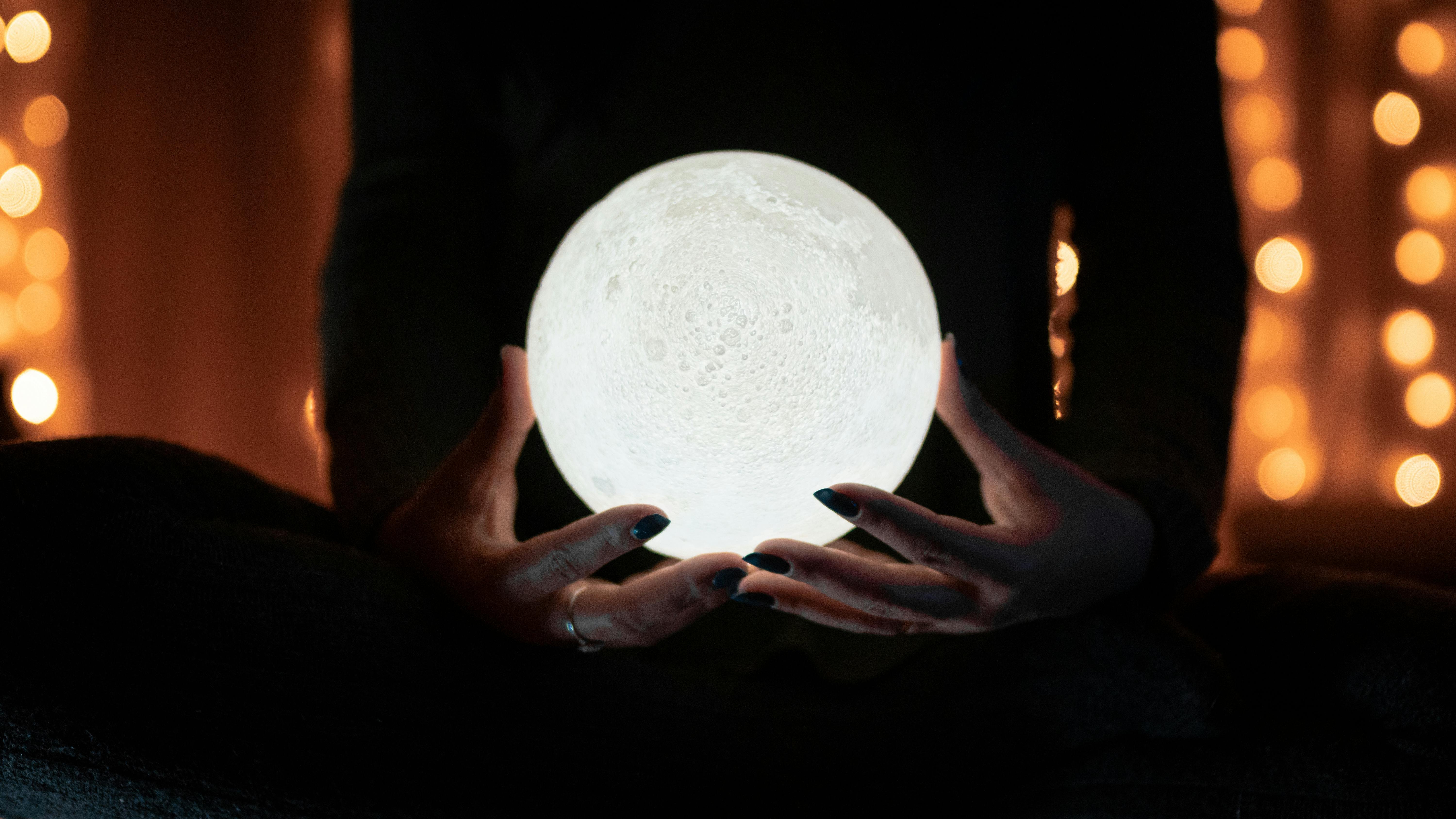
As technology continues to evolve at a rapid pace, churn prediction models and customer engagement strategies are poised to become even more sophisticated. Innovations in artificial intelligence and data processing mean that real-time, highly personalized interactions will increasingly become the norm. Below are a few emerging trends that are set to shape the future of churn prediction.
Real-Time Analytics and Streaming Data
Instead of waiting to process data on a weekly or monthly basis, businesses can now leverage streaming data platforms like Apache Kafka and real-time analytics engines to make on-the-fly predictions. This is particularly relevant for customer-facing applications, where immediate interventions—such as a discount offer when a user appears to be abandoning a shopping cart—can stop churn before it happens. Real-time analytics enable companies to shift from reactive to proactive customer engagement.
Hyper-Personalization
With the influx of data from wearable devices, smart home technologies, and advanced user tracking, the opportunities for personalization are expanding. Future churn prediction models will likely incorporate data from multiple facets of a customer’s lifestyle to build a more holistic profile. Personalized engagement will go beyond simple product recommendations, evolving into experiences uniquely tailored to individual preferences, contexts, and real-world conditions.
Integration of Voice and Chat Interfaces
Voice assistants (like Amazon Alexa or Google Assistant) and chatbots are becoming more integrated into daily life. This opens new avenues for engagement, including personalized reminders, real-time troubleshooting, or proactive notifications. As these interfaces become more conversational, they can gather nuanced data on user sentiment, concerns, and preferences—further refining churn prediction models.
Advanced AI and Deep Learning
Deep learning architectures, such as Transformers and advanced sequence models, already excel in fields like natural language processing and computer vision. As they become more common in customer analytics, these models can decode increasingly complex patterns in user behavior, potentially offering early warnings of churn that simpler algorithms miss. Continual learning models also promise to adapt automatically to changing customer preferences without frequent retraining cycles.
Ethical and Responsible AI
As predictive models grow in power, ethical considerations become more pressing. There is a rising demand for transparency and fairness in algorithms, particularly those that make decisions about customers. Companies may need to demonstrate that their churn prediction practices do not discriminate based on sensitive attributes like race, gender, or age—and that they maintain user data privacy and consent. Regulatory bodies may enforce stricter guidelines, making it crucial for businesses to align their churn prediction strategies with ethical best practices.
In this evolving landscape, businesses that remain agile, data-driven, and customer-centric will find themselves at a competitive advantage. The opportunities to reduce churn, optimize engagement, and create personalized experiences are vast and only set to increase. By anticipating these trends and adapting accordingly, you can not only keep pace with the future but help shape it.
Conclusion
From understanding the fundamental reasons behind churn to leveraging machine learning models that forecast customer attrition, we’ve explored the critical components of a successful churn prevention strategy. Churn prediction models serve as advanced warning systems, enabling businesses to identify at-risk customers early and intervene with targeted engagement tactics. These tactics—ranging from personalized marketing campaigns to loyalty rewards—demonstrate how data-driven insights can be translated into tangible customer retention efforts.
At the core of these initiatives is a commitment to genuine customer engagement. Predictive analytics might highlight who is likely to leave, but it’s the subsequent, personalized strategies that compel customers to stay. Engagement, in turn, drives loyalty, higher lifetime value, and long-term sustainable growth. As technology evolves, businesses that embrace real-time analytics, hyper-personalization, and ethically responsible AI will find themselves at the forefront of churn reduction and customer satisfaction.
In essence, using churn prediction models to keep your customers engaged is not just a tactical move—it’s a strategic imperative. The ability to foresee churn and proactively address it can spell the difference between stagnation and robust growth in today’s highly competitive markets. By continuously refining your models, optimizing engagement strategies, and staying aligned with emerging trends, you can cultivate a loyal customer base that propels your organization forward.
What next?
Ready to put these insights into action? We invite you to explore our additional resources, where you’ll find step-by-step guides, case studies, and expert tips for implementing churn prediction models and advanced engagement strategies in your own organization. Whether you’re new to predictive analytics or looking to refine existing processes, these tools and services can help you get started on the path to stronger customer loyalty and improved bottom-line results.
If you found this article useful, please share it with your colleagues and professional network. Together, we can push the boundaries of what’s possible in customer retention and build sustainable, customer-centric businesses.