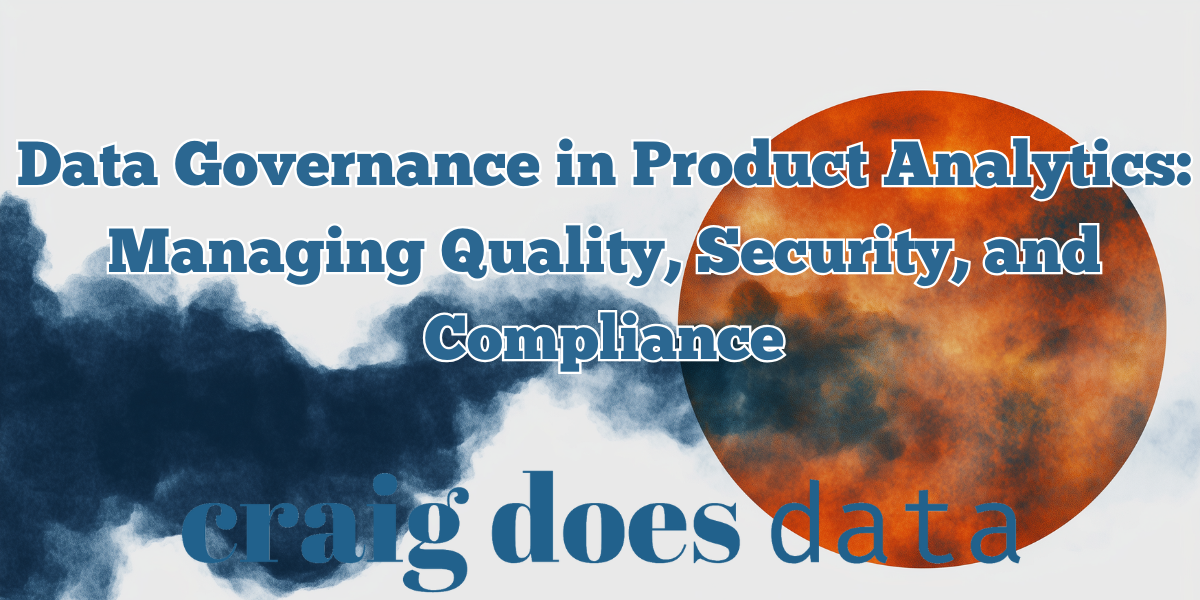
Data Governance in Product Analytics: Managing Quality, Security, and Compliance
In an era where data drives almost every aspect of modern business, the governance of that data stands out as a critical discipline. Organizations around the world rely on product analytics data to guide development decisions, refine user experiences, and maintain a competitive edge. This data can be incredibly valuable—if it is accurate, secure, and compliant with relevant regulations. That is precisely where data governance comes into play. Data governance encompasses the policies, procedures, and frameworks that ensure data is handled responsibly, effectively, and in alignment with business objectives.
Without robust data governance, even the most sophisticated product analytics initiatives risk producing misleading insights, exposing sensitive information, or violating regulatory requirements. Good governance transforms raw data into a strategic asset. It shapes how data is collected, stored, analyzed, and shared, while clearly defining roles and responsibilities throughout the organization. When done well, data governance ensures that product teams can trust the data they use, fosters a culture of accountability, and preserves the integrity of customer information.
This article provides an in-depth exploration of data governance in product analytics, focusing on three key aspects: data quality, data security, and compliance. We will discuss why these elements are essential for any organization seeking to maximize the value of its data while respecting legal and ethical obligations. We will also examine best practices, real-world examples, and forward-looking trends. By the end, you will have a comprehensive understanding of why data governance matters, how to implement it effectively, and what the future may hold for this rapidly evolving field.
1. Understanding Data Governance in Product Analytics
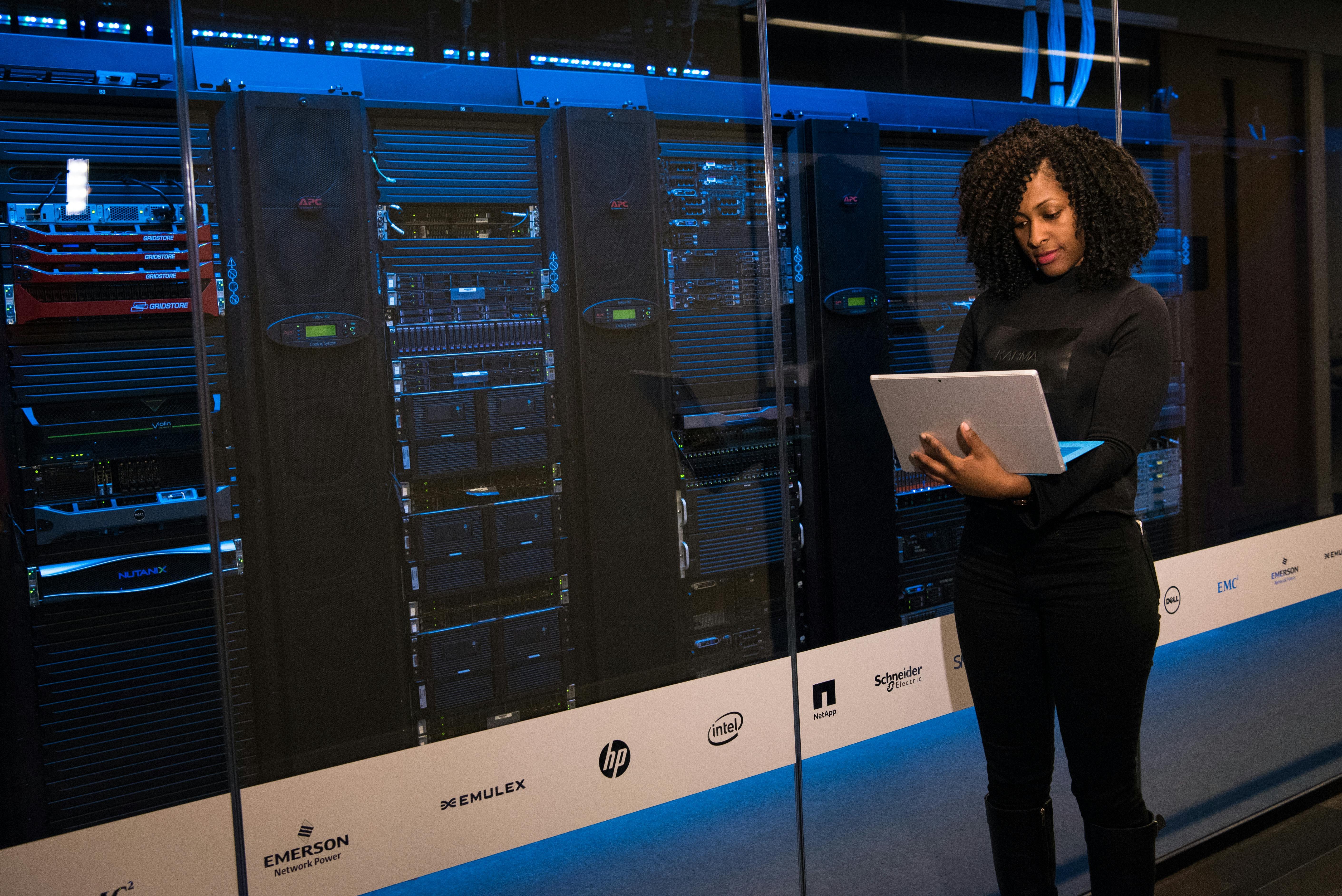
Data governance is often described as the framework of rules, processes, and responsibilities that ensure high data quality and consistent data management practices across an organization. In the context of product analytics, this framework becomes the backbone of how data is gathered, analyzed, and utilized to improve product features and user experience. Data governance establishes the guardrails that prevent misinterpretation, misuse, or mismanagement of data, serving as a guiding principle from collection to reporting.
Beyond definitions, data governance is a crucial strategic function. By clarifying how data can be used in product analytics, governance helps to align data management processes with overarching business objectives. For example, a company aiming to optimize user retention may employ data governance rules to clarify which metrics must be tracked, how they are defined, and who is responsible for ensuring the consistency of these metrics across various departments. When such alignment is lacking, product teams might measure user retention differently, leading to confusion and inconsistent reporting.
There are three key components that typically fall under the umbrella of data governance in product analytics:
- Data Quality: Ensuring that the data you rely on for product decisions is accurate, complete, consistent, and timely.
- Data Security: Safeguarding sensitive and proprietary information from unauthorized access, breaches, and other threats.
- Compliance: Adhering to legal and regulatory requirements that govern how data is collected, stored, and processed.
Each component is interrelated. Good data quality efforts reduce the risk of misinformed decisions. Effective data security measures protect both the organization and its customers. Compliance ensures that business practices are lawful and ethical. Taken together, these components form a cohesive strategy that not only enhances the reliability of product analytics but also builds consumer trust and mitigates organizational risk.
2. Ensuring Data Quality in Product Analytics
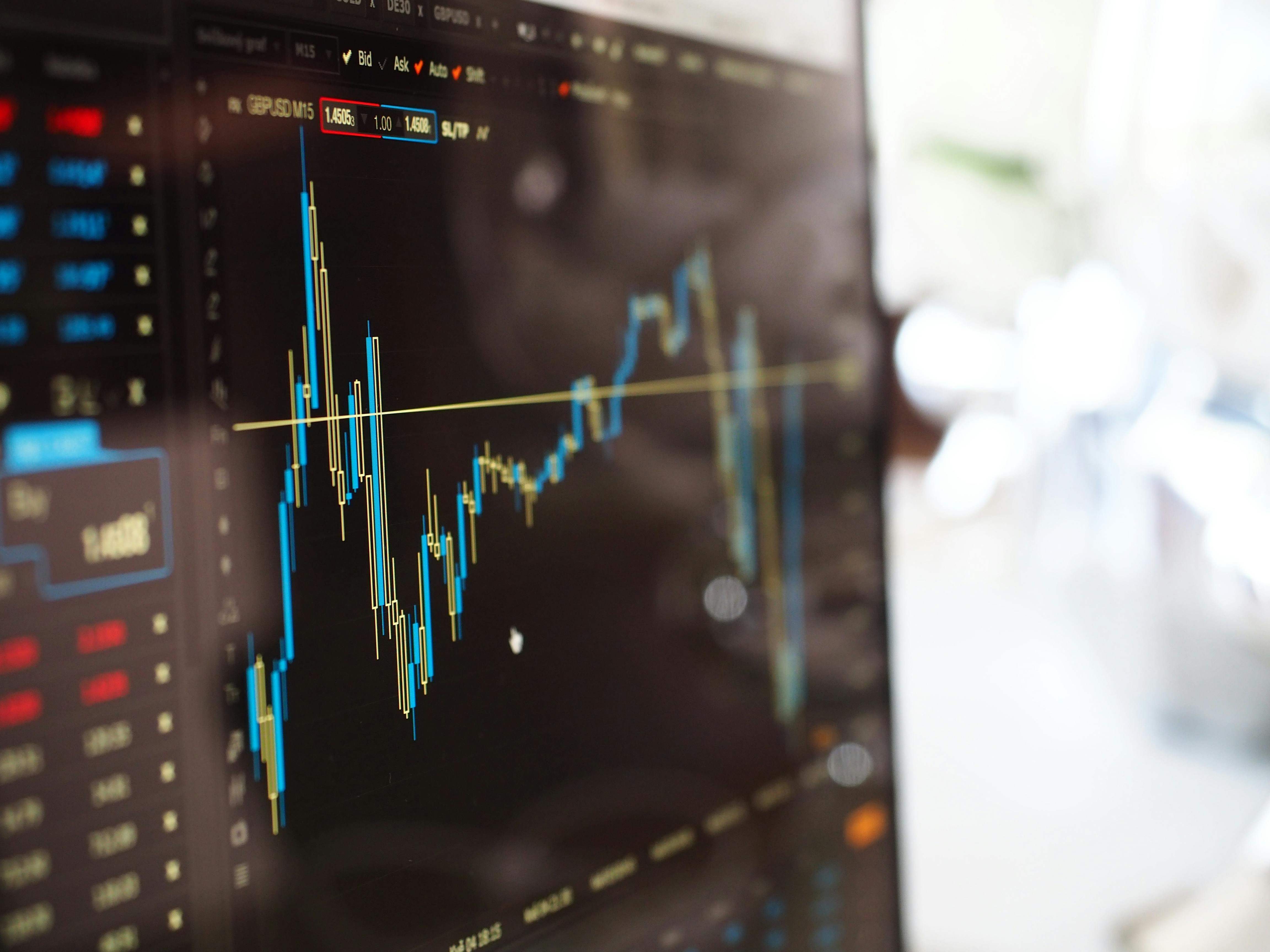
Data quality is the lifeblood of product analytics. Even the most powerful analytical models and visualization tools will fail to deliver meaningful insights if the underlying data is inaccurate or incomplete. High data quality means having data that is correct, consistent, comprehensive, and timely—allowing analysts and stakeholders to make decisions based on trustworthy information.
One common example of poor data quality in product analytics is inconsistent user event tracking. Suppose a company’s mobile app team logs “AppOpened” events while the web team uses “AppOpen” for a similar event. This discrepancy may seem minor, but it can lead to confusion, misalignment in analysis, and erroneous conclusions about how users are engaging with the product. Another frequent issue involves missing data fields—like failing to record user IDs or timestamps properly—which can derail entire analytics pipelines.
The consequences of poor data quality are far-reaching. Decision-makers might invest in product features that users do not actually want, or risk miscalculating the return on investment for new product developments. Additionally, flawed data can erode trust among stakeholders, creating an environment where insights are perpetually doubted and second-guessed.
To mitigate these risks, organizations often employ several strategies for enhancing data quality:
- Data Cleaning: Systematically identifying and correcting inaccuracies or inconsistencies. This can involve removing duplicate records, fixing misspelled entries, and standardizing formats.
- Validation Rules: Implementing checks and constraints that ensure data follows specific criteria. For instance, a validation rule could enforce that all timestamps are in a particular time zone or that all event names adhere to a naming convention.
- Standardization: Defining consistent data definitions and metrics. If all teams agree on what “Daily Active Users” means, the metric is more likely to be reported accurately across different product lines.
- Automated ETL Pipelines: Using Extract, Transform, Load (ETL) tools to automatically format and load data into storage systems. Well-designed pipelines include error checks and automated notifications for anomalies.
In addition to these strategies, there are several tools designed to improve data quality. Data quality platforms and data observability solutions can monitor data pipelines in real time, flagging anomalies or data drift. Master Data Management (MDM) systems help maintain a “single source of truth” for key data entities, such as product information or customer details. By integrating these tools and techniques into everyday product analytics workflows, organizations can ensure that the data feeding critical decisions is both reliable and actionable.
Ultimately, prioritizing data quality is an ongoing process rather than a one-time fix. As products evolve and user behaviors change, data schemas and definitions will also need to be revisited and updated. A collaborative approach—where data engineers, product managers, and analysts communicate closely—helps organizations maintain and enhance data quality over time.
3. Securing Data in Product Analytics
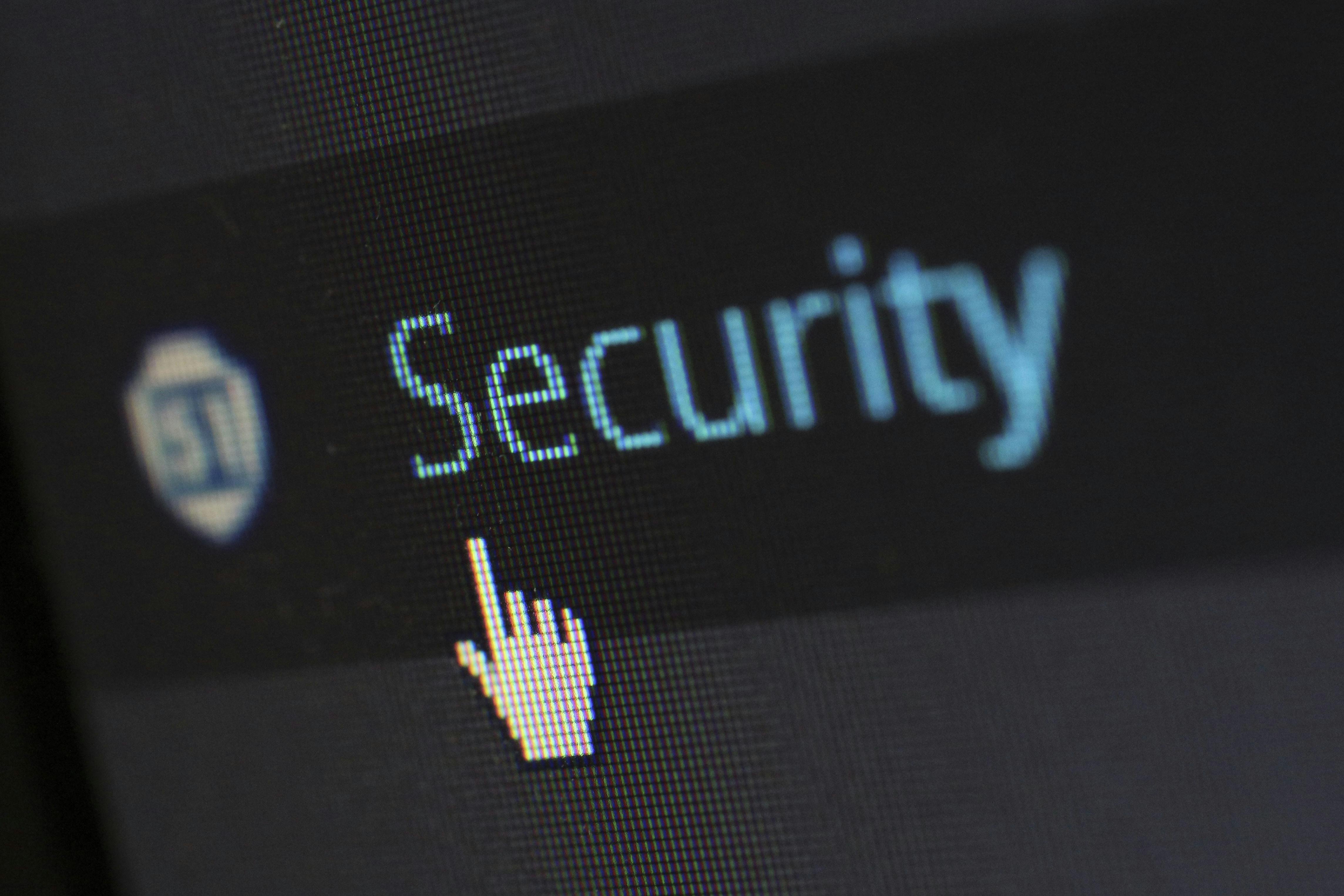
With massive amounts of customer and product data flowing through organizations, ensuring data security has become paramount. Security breaches can expose sensitive information, harm corporate reputations, and lead to severe financial and legal consequences. In the realm of product analytics, where data often contains detailed user interactions and behavioral patterns, the stakes are especially high.
Common threats to data security include external attacks (such as hacking attempts or phishing scams) and internal vulnerabilities (like unauthorized access by employees or contractors). Attackers often target analytics systems because these systems can hold comprehensive data sets that may include customer details, product usage statistics, and proprietary business insights. Even seemingly benign metadata can reveal patterns about product development timelines or partnerships that competitors could exploit.
To counter these threats, organizations should adopt a layered security approach. This involves combining multiple safeguards to protect data at rest and in transit, monitor for unusual activity, and control user access. Key best practices include:
- Encryption: Encrypt data both when it is stored in databases or data lakes (encryption at rest) and when it is transmitted between systems (encryption in transit). This ensures that even if an attacker gains access to raw data files, the information remains unreadable.
- Access Controls: Implement role-based access control (RBAC) to grant permissions based on job responsibilities. For instance, a product manager may only need to see aggregate user statistics rather than individual user records. Requiring multi-factor authentication (MFA) further reduces unauthorized entry points.
- Network Segmentation: Isolate analytics environments from other parts of the corporate network. This limits the blast radius if a breach does occur, preventing attackers from easily moving from one system to another.
- Monitoring and Incident Response: Use intrusion detection systems, log management solutions, and security information and event management (SIEM) tools to monitor data-related activities. An incident response plan outlines how the organization will react in the event of a breach, enabling rapid containment and recovery.
Cybersecurity frameworks such as the National Institute of Standards and Technology (NIST) Cybersecurity Framework provide structured guidelines that organizations can follow. These frameworks cover risk assessment, threat monitoring, and recommended security controls, which can be mapped directly to an organization’s data governance policies. Adhering to established frameworks not only improves your security posture but also facilitates alignment with industry best practices and regulatory requirements.
Overall, data security is not merely an IT or cybersecurity department’s responsibility—everyone from executives to product analysts plays a role. Product managers must consider user privacy and safety when designing features that collect data. Analysts should practice responsible data handling and storage, and engineers must implement security measures in the software architecture. By fostering a culture of security awareness, organizations make significant strides in keeping their product analytics data safe.
4. Compliance in Product Analytics
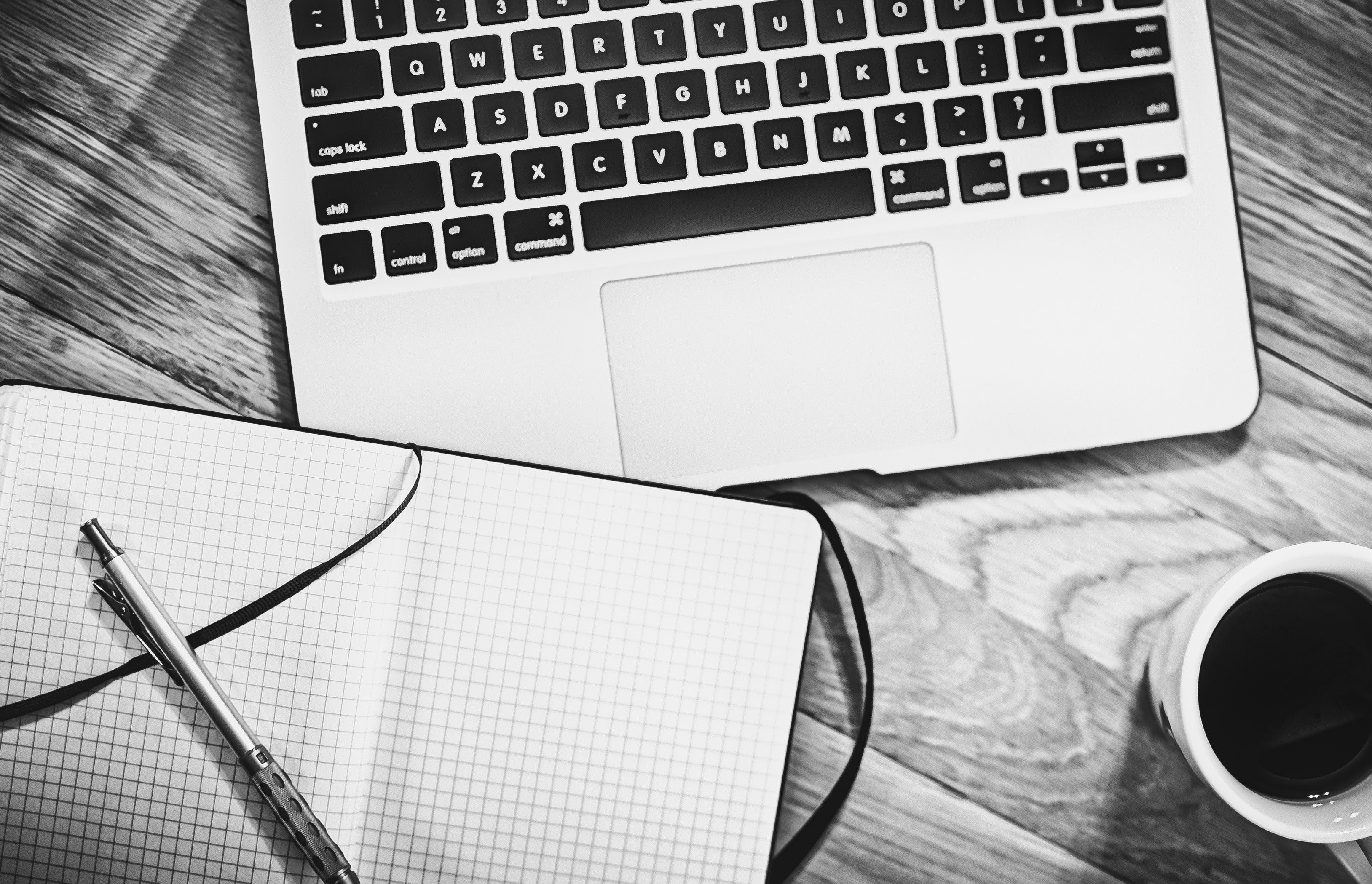
Alongside quality and security, compliance forms the third pillar of a well-rounded data governance program. Compliance refers to the adherence to legal and regulatory requirements that govern how data is collected, stored, processed, and shared. In product analytics, this might encompass everything from handling personally identifiable information (PII) responsibly, to adhering to international data transfer restrictions.
Among the most well-known regulations in data governance are the European Union’s General Data Protection Regulation (GDPR) and the California Consumer Privacy Act (CCPA) in the United States. Both laws focus on protecting consumer privacy, giving individuals rights over how their data is used, stored, and shared. If your product analytics stack collects any data from EU residents, GDPR compliance becomes a non-negotiable requirement, even if your company is based elsewhere.
Non-compliance can lead to hefty fines, legal sanctions, and irreparable damage to a company’s reputation. Besides GDPR and CCPA, other sector-specific regulations—like HIPAA for healthcare in the U.S.—may also be relevant. The complexity of this regulatory landscape underscores the importance of a robust compliance strategy.
To ensure compliance in product analytics, organizations should:
- Conduct Regular Audits: Periodic reviews of your data collection practices, storage mechanisms, and access logs can help identify potential compliance gaps. Audits also serve as evidence of proactive compliance efforts in case of regulatory inquiries.
- Document Data Flows: Maintain clear records of where data is coming from, how it is used, and where it is sent. Data lineage tools can help track transformations throughout your analytics pipeline, crucial for demonstrating compliance and responding to user data requests.
- Implement Consent Management: Ensure that you have clear, user-friendly methods for obtaining and storing user consents. Under regulations like GDPR, you must be able to prove that users have opted in to data collection where applicable.
- Staff Training: Train employees on privacy and compliance requirements. Product managers, analysts, and engineers should understand the legal constraints that apply to their day-to-day handling of user data.
When managing compliance across different jurisdictions, organizations may need to tailor their approach based on local laws. This includes storing data in specific geographic regions to meet data residency requirements, implementing localized consent forms, or appointing data protection officers in certain regions. Successful compliance strategies often rely on a blend of legal expertise, technological tools, and clear internal guidelines that empower employees to handle data responsibly.
5. Building a Robust Data Governance Framework
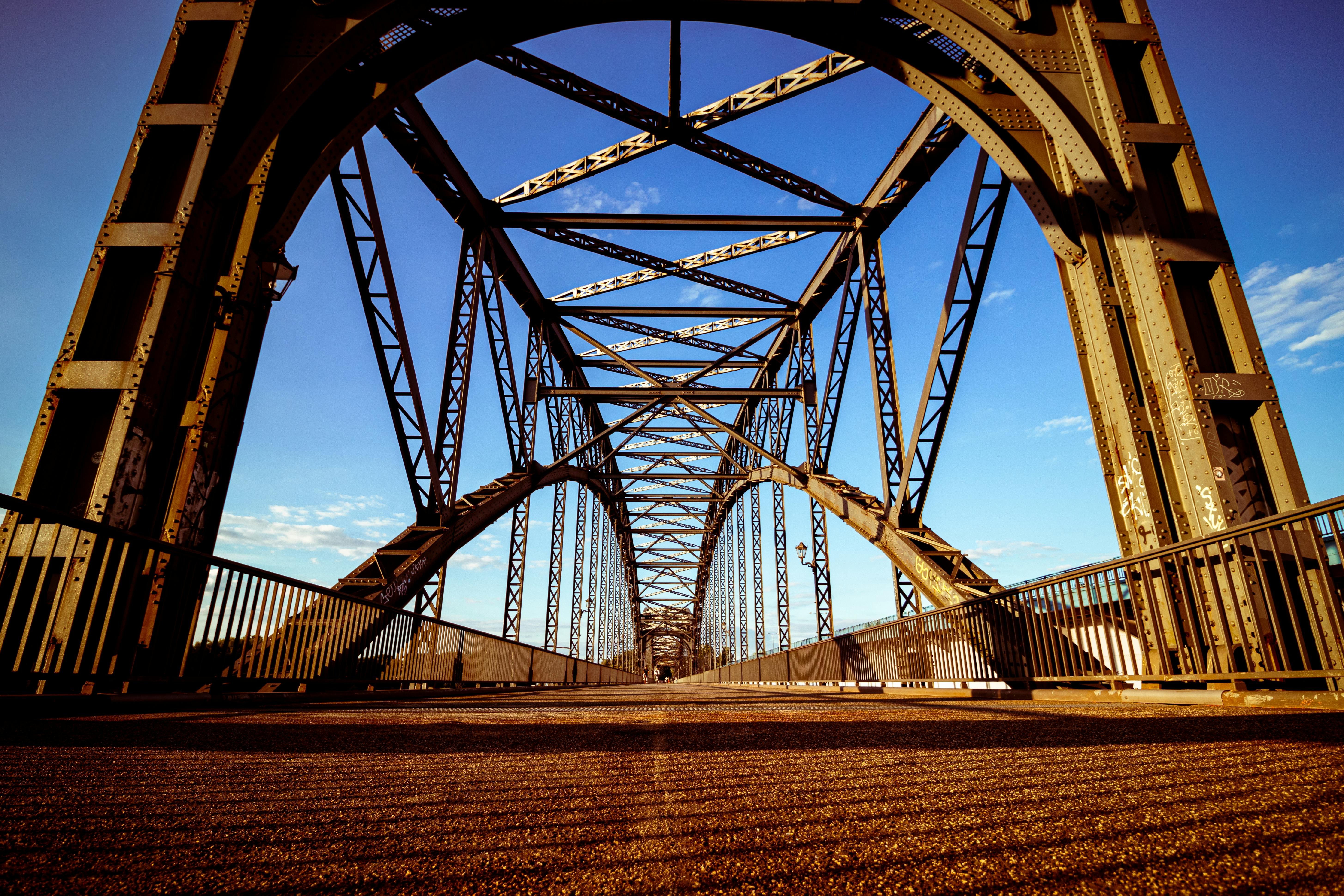
A robust data governance framework is the scaffolding that supports data quality, security, and compliance efforts. It formalizes processes, assigns clear roles, and provides a roadmap for how data is treated throughout its lifecycle. Organizations lacking such a framework often struggle with siloed data, inconsistent metrics, and reactive crisis management when breaches or compliance issues arise.
Establishing this framework typically involves several steps:
- Assess Current State: Start by examining your existing data infrastructure, cataloguing data sources, and identifying pain points in quality, security, or compliance.
- Define Objectives: Clarify what you want to achieve with your data governance program. For product analytics, objectives might include improving the accuracy of usage metrics, securing customer data, or meeting new compliance standards.
- Develop Policies and Procedures: Write down how data should be collected, stored, accessed, and retained. Outline requirements for metric definitions, user permissions, and incident response protocols.
- Assign Roles and Responsibilities: Designate data owners, who are accountable for specific data sets, and data stewards, who handle day-to-day management tasks. Establish a governance council to oversee strategic decisions.
- Implement Technology Solutions: Use tools like data catalogs, data quality platforms, and security solutions to enforce policies automatically where possible.
- Measure and Iterate: Track key performance indicators (KPIs) related to data governance, such as the number of data quality issues resolved or time to detect security incidents. Use these metrics to refine the governance framework continuously.
In terms of team structure, many organizations create a Data Governance Council composed of stakeholders from various departments—IT, Security, Legal, Product, and Data Analytics. This council oversees governance policies and resolves cross-functional conflicts. Data owners, who may be product managers or heads of certain business units, carry responsibility for the content and accuracy of the data relevant to their domains. Data stewards are often more hands-on, managing day-to-day data validation, classification, and lifecycle management.
A case study illustrating a robust data governance framework in action is a global e-commerce company that faced repeated issues with inconsistent product taxonomy across different marketplaces. By launching a governance council and defining global standards for product attributes, the company successfully reduced data discrepancies, streamlined analytics reporting, and improved the customer shopping experience. Regular audits and real-time data quality checks allowed them to catch errors early, reinforcing trust in their analytics.
Developing a comprehensive data governance policy can be a significant undertaking, but the benefits are worth the effort. When employees have a clear reference for how to handle data, they can align their actions with overall organizational goals, reducing the potential for errors, security lapses, and compliance violations. This alignment is especially impactful in product analytics, where multiple teams must collaborate to collect and interpret user data effectively.
6. Challenges and Solutions in Data Governance
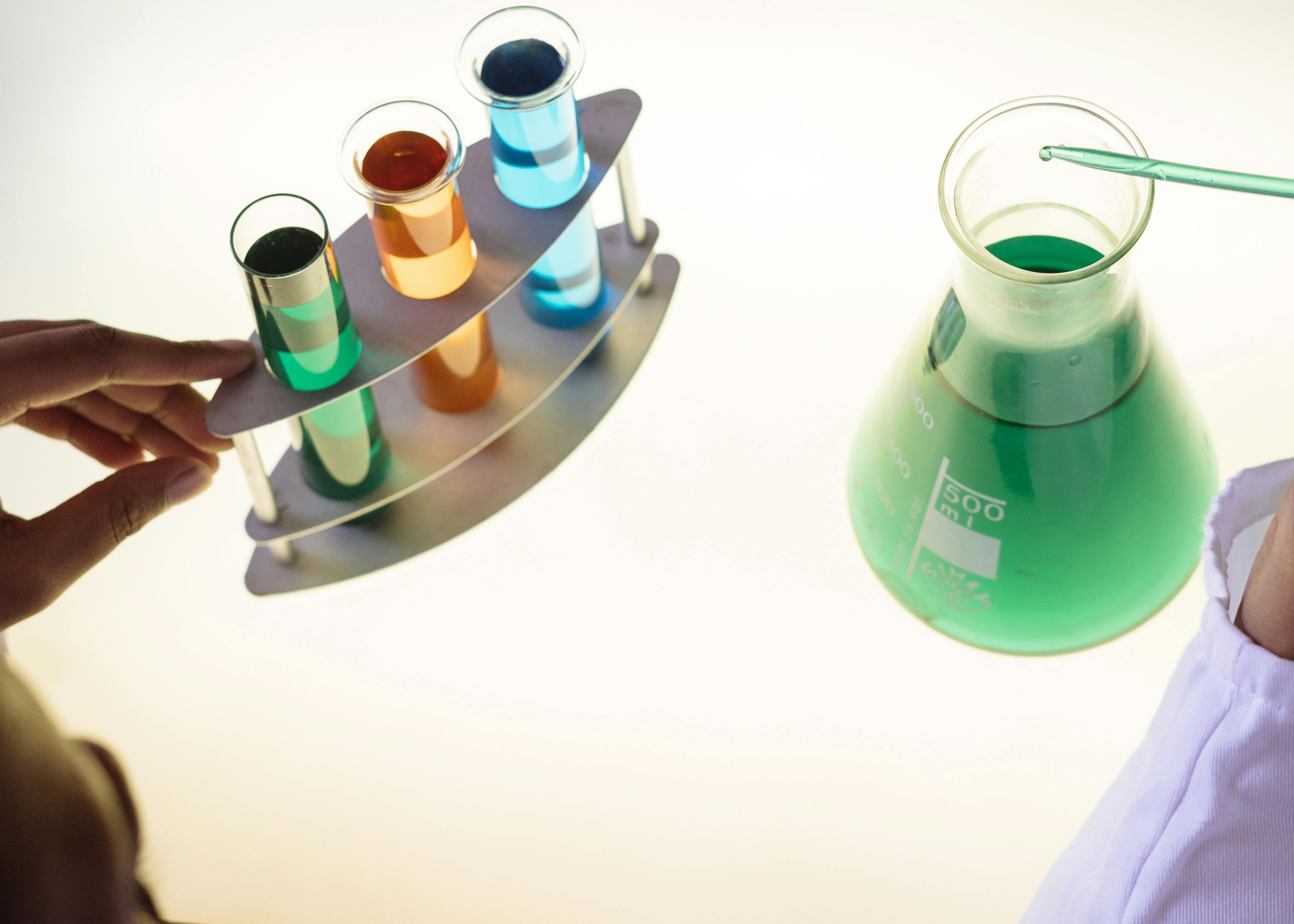
Implementing data governance is no small task, and organizations often encounter a range of challenges along the way. Understanding these obstacles in advance—and having a strategy to address them—can significantly increase the likelihood of a successful program.
One frequent challenge is organizational resistance. Data governance policies can be perceived as bureaucratic or restrictive, especially if employees are accustomed to easily accessing or modifying data without oversight. Overcoming this resistance often requires clear communication about the benefits of governance—how it can improve data usability, protect sensitive information, and align departmental goals.
Another challenge is data silos. In many companies, data is spread across multiple systems managed by different departments. Each team may have unique ways of naming, storing, or interpreting data. Bringing these silos together under a unified governance framework can be labor-intensive, requiring significant data engineering work, cross-team negotiation, and sometimes cultural change.
Lack of leadership support can also derail governance initiatives. Without executive buy-in, it is difficult to secure the resources, time, and policy changes necessary to enforce standards. Demonstrating ROI—for instance, by showing how data governance can accelerate product development or reduce risk—can help build the case for leadership involvement.
The following are some practical solutions to these challenges:
- Change Management: Incorporate change management practices, such as stakeholder analysis and transparent communication plans. Educate employees on how governance improves their workflow rather than hinders it.
- Gradual Implementation: Pilot governance policies in specific teams or for particular data sets before rolling out across the organization. This allows for iterative improvements and demonstration of success stories.
- Technology Integration: Use data integration platforms or data virtualization tools to break down silos without needing to physically move all data into one repository. A distributed approach can be more scalable and less disruptive.
- Quantify the Benefits: Track metrics like reduced time to find accurate data or improved data quality scores. Present these metrics to leadership to secure ongoing support and funding.
Technological solutions are increasingly addressing data governance complexities. Modern data catalogs can automatically discover new data sets, populate metadata, and apply data quality checks. AI-driven classification tools can help identify sensitive data or detect anomalies. These tools, combined with a well-defined governance process, significantly reduce manual workloads and enhance the efficiency of data governance initiatives.
Ultimately, data governance challenges often boil down to aligning people, processes, and technology. By recognizing these friction points early and implementing targeted solutions, organizations can develop a governance program that is not only effective but also embraced by stakeholders across the enterprise.
7. The Future of Data Governance in Product Analytics
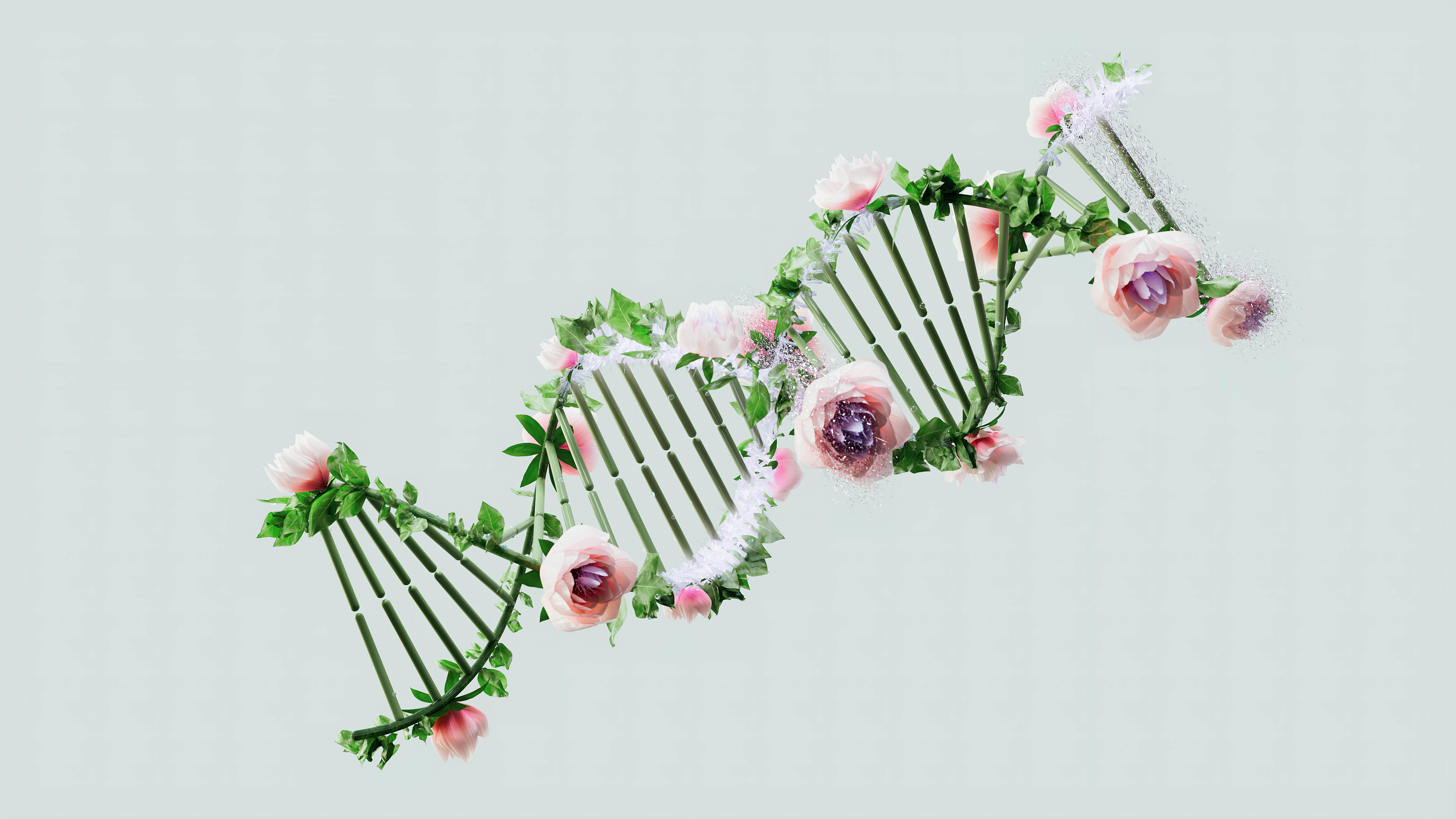
As products become more data-driven and user-centric, data governance will continue to evolve. Emerging technologies, new business models, and ever-changing regulatory landscapes will shape how organizations manage and safeguard their data in the years ahead. By staying proactive, companies can leverage these developments to strengthen their data governance strategies and generate even more valuable insights from product analytics.
One major trend is the increasing use of artificial intelligence (AI) and machine learning (ML) for data governance tasks. AI-powered tools can automate data classification, detect unusual access patterns that could indicate a security breach, and even forecast data quality issues before they become serious. For example, a system might recognize that certain fields are frequently prone to input errors, prompting proactive validation or training for data-entry teams.
Another emerging concept is the data mesh, which promotes a decentralized approach to data management. Rather than funneling data into one centralized warehouse, teams maintain ownership of their data “domains” while adhering to shared governance standards. In this model, each product team might be responsible for the data it generates, ensuring quality and access controls at the source. A well-implemented data mesh can reduce bottlenecks and encourage innovation, but it also raises new governance challenges related to cross-domain collaboration and standardization.
On the regulatory front, we can expect stricter rules around data privacy and responsible AI. Legislation similar to GDPR is cropping up across different jurisdictions, and global data transfers are becoming increasingly scrutinized. As ML and advanced analytics begin to influence product decisions in real-time, regulators are paying closer attention to the fairness, transparency, and accountability of these systems. Future governance frameworks will likely include guidelines on “explainable AI,” requiring companies to document how algorithms process personal data and make decisions that affect users.
Overall, the future of data governance in product analytics will be characterized by increasing automation, decentralization, and scrutiny. Organizations that invest in forward-thinking governance strategies—balancing the drive for innovation with compliance and ethical considerations—will be well-positioned to harness the full potential of their product analytics data.
Conclusion
Data governance is a critical, multifaceted discipline that underpins the success of product analytics initiatives. By focusing on data quality, organizations ensure that insights are accurate and actionable. Through stringent security measures, they protect both their customers and their competitive advantage. Compliance frameworks help steer data usage along legal and ethical boundaries, maintaining user trust and avoiding costly penalties.
Whether you are a startup aiming to build responsible data practices from the ground up or an established enterprise seeking to modernize your approach, robust data governance is an investment that pays dividends. It aligns cross-functional teams, promotes reliable data-driven decisions, and sets a strong foundation for innovation. As new technologies and regulations continue to reshape the data landscape, an adaptable governance strategy will be essential for navigating the complexities of product analytics.
Ultimately, implementing effective data governance is about more than just adhering to best practices—it is about fostering a culture of responsibility, collaboration, and continuous improvement. By proactively managing quality, security, and compliance, organizations can unlock the true potential of their product analytics data and develop products that resonate with users while respecting their privacy and rights.
What next?
Ready to strengthen your data governance framework? Explore our additional resources on data quality management, cybersecurity best practices, and compliance guidelines to elevate your product analytics.
We also invite you to browse our library of articles and guides to deepen your understanding of modern data strategies. From practical tips on building secure data pipelines to in-depth analyses of emerging compliance requirements, our resources will help you stay at the forefront of data governance and product analytics innovation.