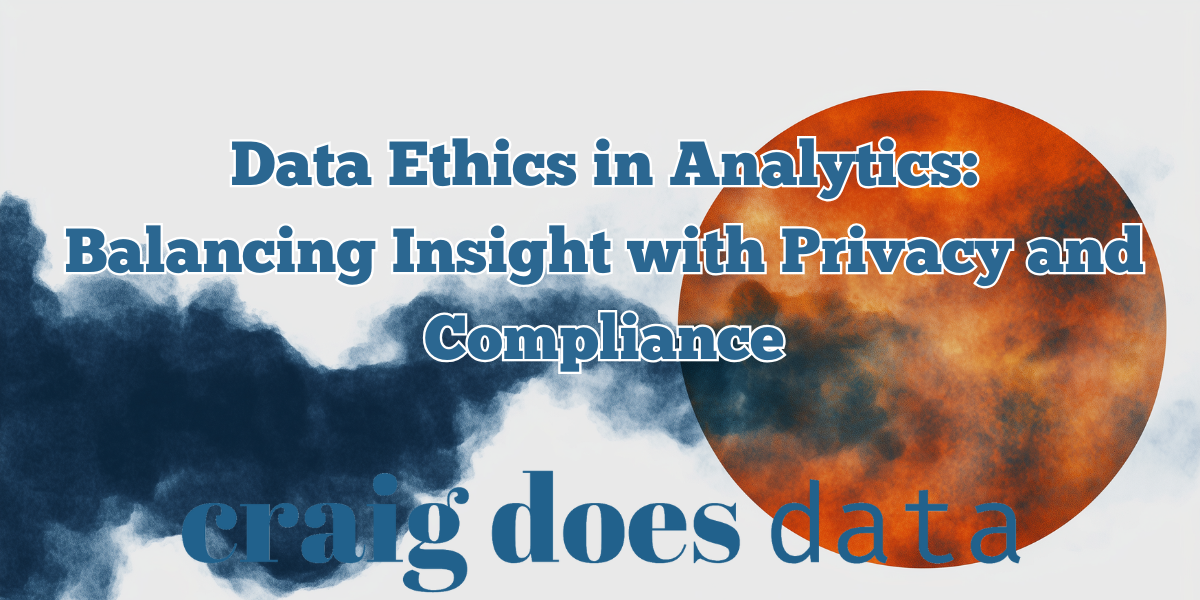
Data Ethics in Analytics: Balancing Insight with Privacy and Compliance
In the modern digital landscape, data powers everything from personalized shopping experiences to predictive health models. Organizations big and small rely on analytics to derive insights, shape strategies, and drive innovation. However, this data revolution has also raised significant questions around privacy, security, and fairness. As data becomes an ever more powerful resource, the need for ethical considerations in its collection, storage, and analysis grows increasingly urgent. Data Ethics in Analytics is no longer just a buzzword; it is a fundamental principle that can determine the trustworthiness of companies and the satisfaction of consumers.
Striking the balance between gaining meaningful insights and respecting individual privacy and compliance obligations is both an ethical necessity and a business imperative. With consumer trust at stake, organizations that fail to adhere to ethical standards risk reputational damage, legal repercussions, and a breakdown in customer relationships. The stakes have never been higher, and navigating this terrain requires an in-depth understanding of data ethics, privacy regulations, and best practices.
This article explores the concept of Data Ethics in Analytics, examines the critical role of data privacy, explains the importance of compliance, and offers strategies to help businesses harmonize insight generation with ethical obligations. As society becomes more data-driven, understanding how to responsibly handle and analyze data is vital for every professional involved in the analytics lifecycle.
1. Understanding Data Ethics in Analytics
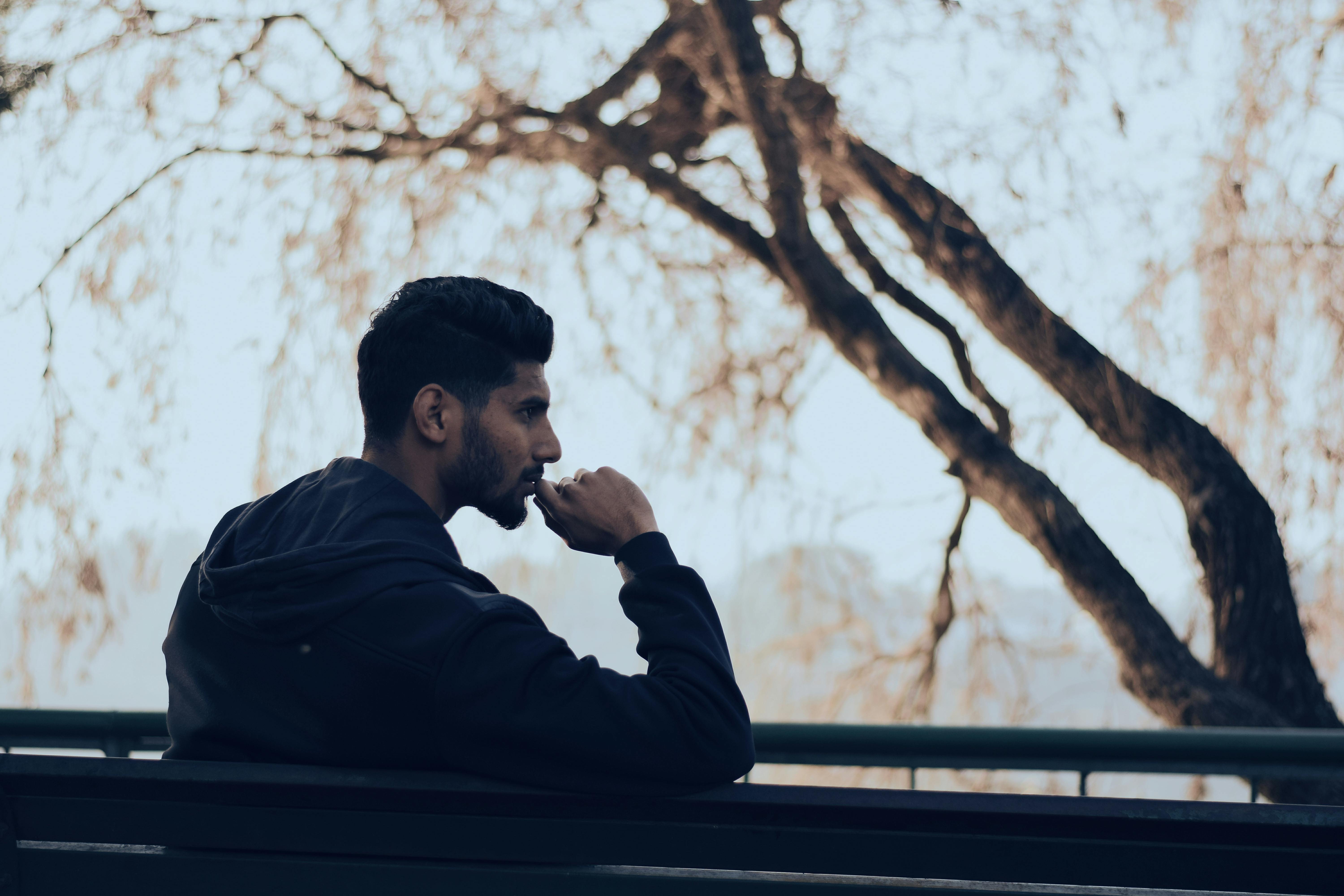
Data ethics refers to a system of moral principles that govern how data is collected, shared, and used. In the context of analytics, data ethics ensures that organizations leverage information in a way that respects individual rights, promotes fairness, and mitigates harm. While the concept of ethics has been part of human societies for millennia, its application in the data-driven environment is relatively recent. As the volume and velocity of data increase, the need for robust ethical frameworks becomes more critical.
Ethical considerations in analytics go beyond just following the law. They are about assessing the broader impact of data practices on stakeholders, including customers, employees, and society at large. An ethical approach to data acknowledges the inherent power imbalance between data collectors (organizations) and data subjects (individuals). By treating data with care and respect, analytics professionals can help prevent abuses, such as discriminatory profiling or unwarranted surveillance.
There are various frameworks that guide data ethics, often centered on principles such as transparency, accountability, and fairness. One widely referenced framework is the FATE model (Fairness, Accountability, Transparency, and Ethics) promoted by many researchers in machine learning and artificial intelligence. While originally developed for AI, these concepts are equally applicable to general data analytics. Fairness speaks to nondiscriminatory practices and equitable treatment of all data subjects; accountability ensures that individuals or teams own responsibility for data outcomes; transparency involves openness about data practices and processes; and ethics ensures alignment with societal values and moral principles.
Consider a large retailer using customer data for targeted marketing campaigns. Suppose the algorithms they employ inadvertently discriminate against specific ethnic groups by showing them fewer or less beneficial promotions. While this may not violate any explicit regulation if there's no protected attribute directly considered in the algorithm, the outcome might still be ethically problematic. It undermines the principle of fairness and can damage trust if uncovered.
Real-world examples of ethical dilemmas in analytics abound. Think of social media platforms that prioritize content based on engagement metrics, sometimes amplifying misinformation or harmful content for the sake of user retention. Or consider credit scoring models that use questionable proxy variables that correlate with race or other sensitive attributes, resulting in unfair lending decisions. In these scenarios, legal compliance may not always be enough to address the deeper ethical issues at play.
Ultimately, understanding data ethics in analytics demands that organizations go beyond the letter of the law. It is about ensuring that advanced technologies and predictive models serve the public good, respect individual autonomy, and maintain societal trust in the power of data. Data ethics must be woven into organizational culture, informing processes, policies, and the daily decisions made by data professionals.
2. The Role of Data Privacy
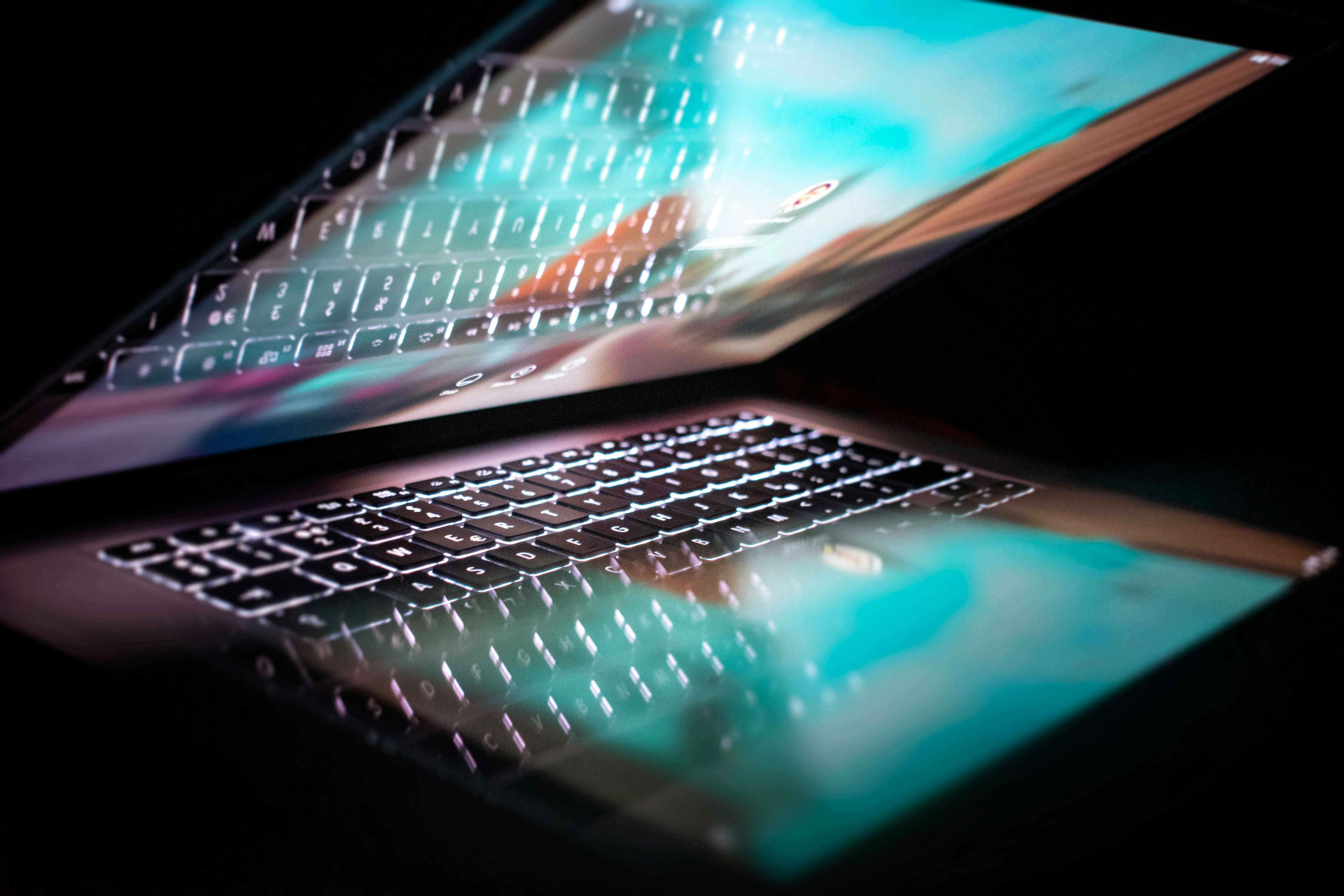
Data privacy is a foundational aspect of ethical data practices. It concerns the rights of individuals to have control over how their personal information is collected, used, and shared. In an era marked by high-profile data breaches and widespread data collection, privacy has become a key element of consumer trust and a substantial driver of regulatory action worldwide. Failing to protect privacy not only causes legal troubles but also inflicts significant damage to a company’s brand reputation.
Privacy affects analytics in multiple ways. First, it limits the kinds of data that organizations can gather and store. Second, it imposes constraints on how that data can be utilized and shared, often requiring additional safeguards such as de-identification or anonymization. Finally, privacy considerations can directly impact the algorithms and models employed, necessitating privacy-preserving techniques like differential privacy or homomorphic encryption for particularly sensitive use cases.
Privacy concerns don’t just arise from external threats such as hackers; they also emerge from internal processes. For instance, if data scientists have access to raw datasets containing personal information, the risk of inadvertent data exposure or misuse increases. Organizations must implement strong internal controls, limiting who can access sensitive data, and under what circumstances. This is where role-based access controls and data minimization principles become crucial.
On the regulatory front, laws such as the General Data Protection Regulation (GDPR) in the European Union and the California Consumer Privacy Act (CCPA) in the United States have significantly shaped data privacy practices. The GDPR, for example, mandates that organizations must have a legal basis for collecting and processing personal data, must honor individuals’ requests to access or delete their data, and must notify authorities of major data breaches in a timely fashion. Meanwhile, the CCPA grants California residents specific rights to know what personal data is being collected, why it’s collected, and how it’s used or shared.
Case studies of privacy breaches demonstrate just how damaging poor privacy practices can be to analytics efforts. For instance, the Cambridge Analytica scandal revealed how data collected under the guise of academic research was used to influence political advertising. Beyond the immediate regulatory penalties, the breach severely eroded trust in the social media platform involved. Another example is the Yahoo data breach, which compromised the personal information of billions of users, underscoring the catastrophic scale of potential privacy failures. Unfortunately, these kinds of breaches continue to happen in multiple industries. These incidents remind us that privacy protections are not optional add-ons, but indispensable components of any data strategy.
Maintaining strong data privacy is not just about avoiding regulatory fines or lawsuits; it is also about preserving public trust. When consumers feel confident that their personal data is handled responsibly and securely, they are more likely to share information, resulting in richer datasets and deeper analytics insights. This trust-based relationship is key for organizations aiming to maximize the value of data while adhering to ethical standards.
3. Compliance in Data Analytics
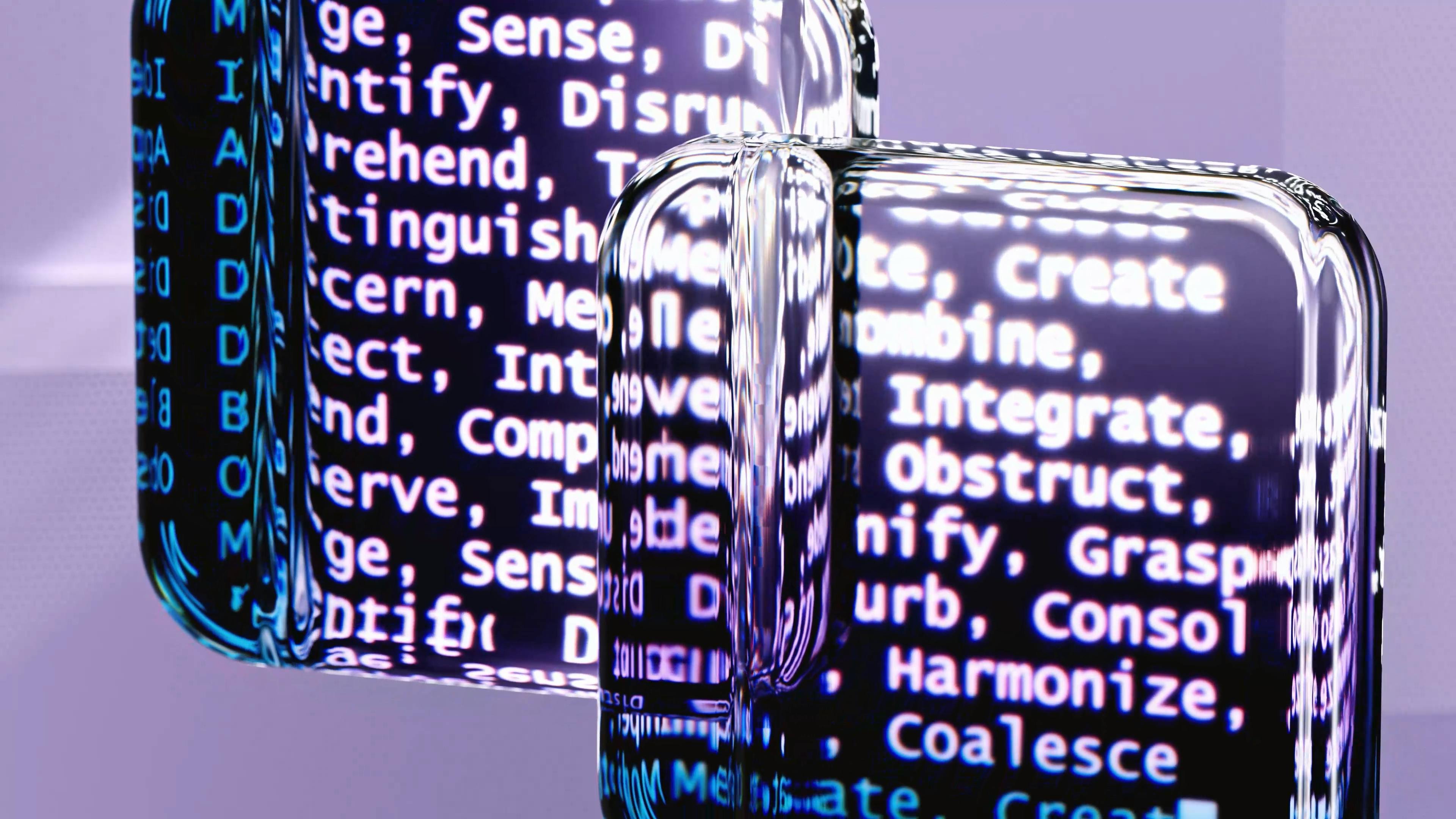
Compliance in data analytics refers to the adherence to laws, regulations, standards, and contractual obligations that govern how data is collected, processed, stored, and shared. While data privacy focuses primarily on the individual’s rights, compliance ensures that organizations meet all legal and regulatory requirements in their data handling processes. This includes measures to protect the confidentiality, integrity, and availability of data, as well as mechanisms to document and demonstrate these protections to external authorities.
Several key regulations significantly impact data analytics. We have already mentioned the GDPR (General Data Protection Regulation), which has a broad reach and strict penalties for non-compliance. Another regulation is the Health Insurance Portability and Accountability Act (HIPAA), which focuses on safeguarding medical information in the United States. HIPAA mandates strict controls around Protected Health Information (PHI), which in turn influences the types of data analytics possible in healthcare settings. Additionally, there is the California Consumer Privacy Act (CCPA), which grants Californian residents the right to understand and control how businesses handle their personal data. On a global scale, many other regions and countries are enacting privacy and data protection laws modeled after the GDPR, continuously raising the compliance bar.
Compliance might sound daunting, especially given the evolving regulatory environment. However, it is also a valuable asset. By building compliance into their data analytics workflows, organizations can foster trust among customers, partners, and regulators. A strong compliance posture signals that a company takes its responsibilities seriously, paving the way for more transparent interactions and safer data practices. Trust is a crucial enabler of data-driven innovation because it encourages stakeholders to share valuable data that can fuel analytics insights.
Many companies excel in compliance by integrating robust frameworks into their business processes. Microsoft, for example, invests heavily in compliance certifications for its cloud services, ensuring that customers can meet industry-specific requirements such as HIPAA, GDPR, or the International Organization for Standardization (ISO) standards. Salesforce also maintains dedicated compliance programs to help its clients navigate multi-jurisdictional regulations. These companies recognize that when customers can be confident in the compliance of the platforms they use, it removes significant barriers to innovation and collaboration.
Ensuring compliance in data analytics typically involves implementing a combination of policies, procedures, and technologies. Common practices include:
- Data Classification: Labeling data based on sensitivity and regulatory requirements to guide handling procedures.
- Access Controls: Limiting data access to authorized individuals and enforcing strong authentication measures.
- Encryption: Protecting data both at rest and in transit using robust cryptographic protocols.
- Audit Trails: Keeping detailed logs of data activities to demonstrate compliance and trace any unauthorized actions.
- Documentation: Creating and maintaining records of data processes, demonstrating adherence to legal and regulatory mandates.
By adopting these measures, analytics teams can reduce risk, minimize liability, and create an environment where responsible data use is second nature. Effective compliance ensures that data is used in a manner that respects the law, safeguards individual privacy, and fosters public confidence in the organization’s analytics initiatives.
4. Balancing Insight with Privacy and Compliance
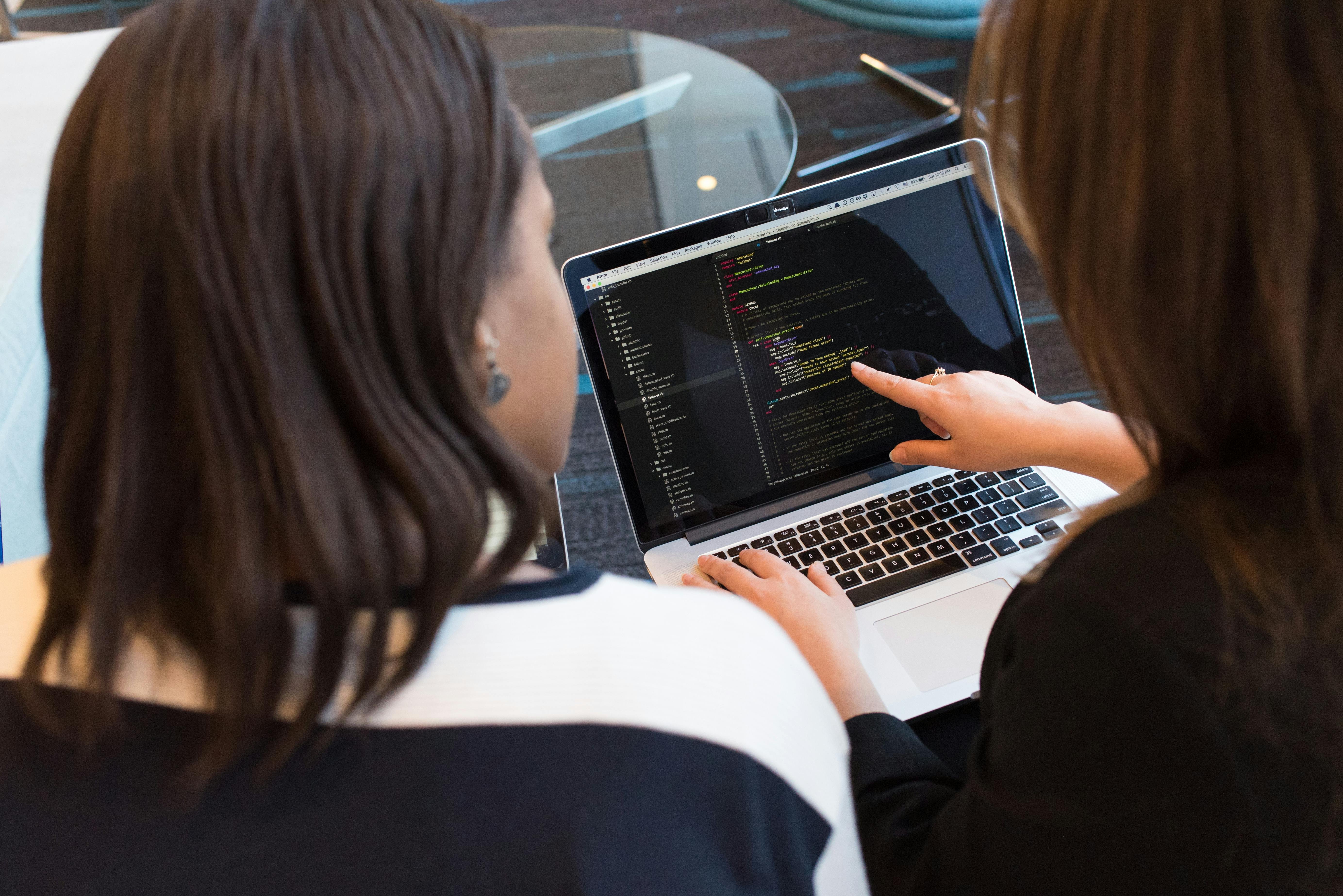
While data analytics promises unprecedented insights, it also brings ethical, privacy, and compliance challenges. The real question is how to achieve a balance where valuable analytics can thrive without compromising individual rights or violating regulations. Achieving this balance is not about limiting analytics altogether; rather, it is about adopting a deliberate, strategic approach to data use.
Data governance plays a critical role in this balancing act. Data governance establishes the overarching policies, standards, and procedures that guide data-related activities within an organization. By defining clear rules on data access, storage, retention, and usage, governance frameworks help align organizational actions with ethical and legal expectations. A mature data governance program includes designated data stewards, cross-functional committees, and continuous training to ensure that everyone in the organization understands their responsibilities.
Organizations looking to harmonize data insights with privacy and compliance often rely on specialized tools and technologies. Solutions for data anonymization can strip out personally identifiable information (PII) before analytics are performed, reducing the likelihood of privacy violations. Data discovery and classification tools can automatically identify sensitive data, aiding compliance. Meanwhile, privacy-preserving machine learning techniques, such as federated learning, allow models to be trained on distributed datasets without pooling all the raw data in one place.
However, adopting these approaches brings unique challenges. For example, anonymized data may still be vulnerable to re-identification attacks if an adversary can cross-reference multiple datasets. Additionally, ensuring compliance with regulations that vary across different regions can be resource-intensive. Successful organizations often adopt a risk-based approach to prioritize high-risk areas for stricter controls, while allowing more flexibility in lower-risk scenarios.
Striking the right balance also requires a strong ethical culture within the organization. No set of policies or technologies can compensate for a disregard of ethical principles. Every member of the analytics team must internalize the idea that powerful data insights come with equally powerful responsibilities. This collective mindset, supported by robust governance structures, is the foundation upon which ethically sound and compliant data analytics can flourish.
5. Best Practices for Ethical Data Analytics
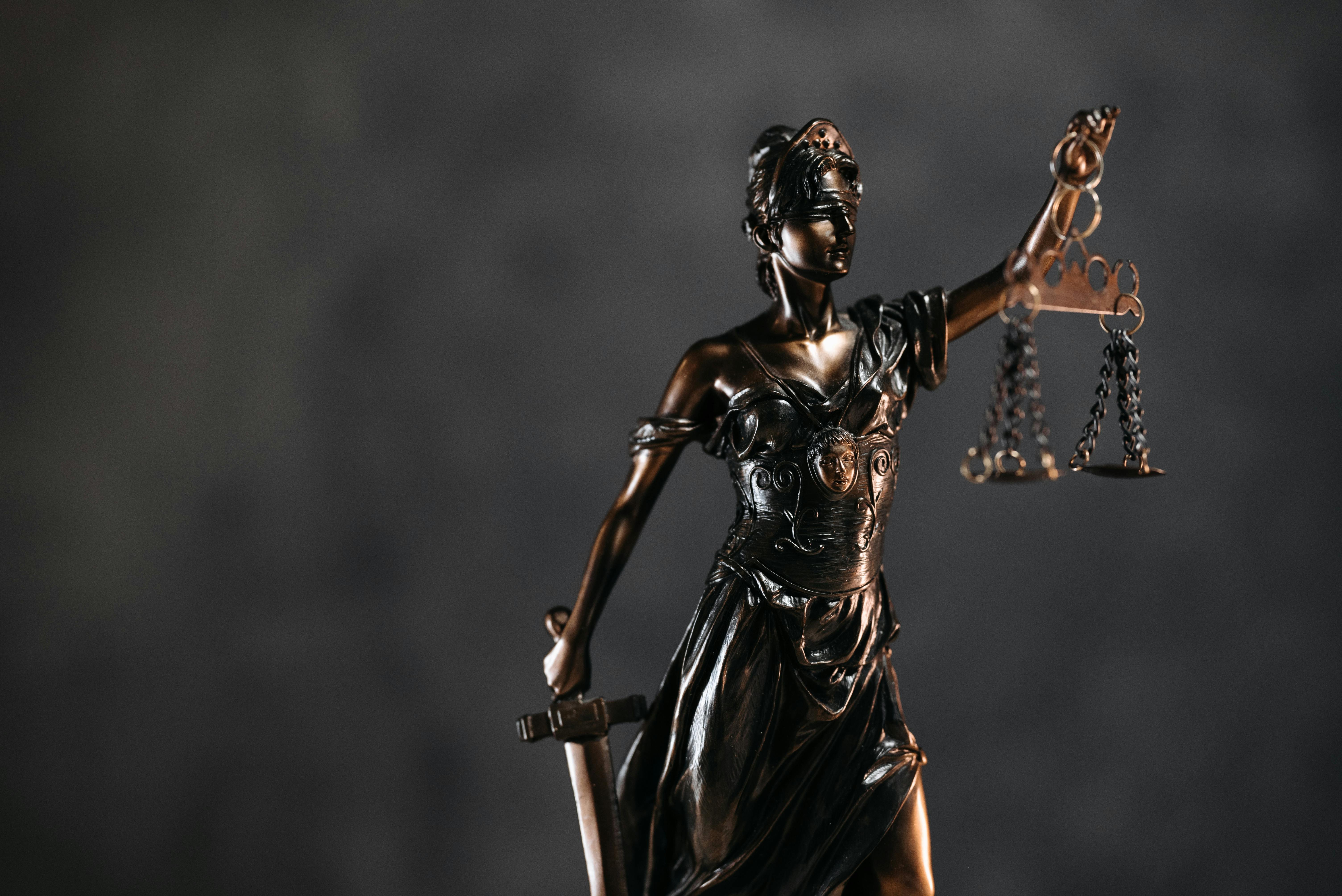
Implementing ethical data analytics is a continual process that evolves with technological advancements and emerging regulations. Organizations that prioritize ethical practices establish robust, adaptable frameworks. Below are some best practices that can guide ethical analytics initiatives:
- Ethical Data Collection: Only collect data that is absolutely necessary for the analysis at hand. Employ a clear consent mechanism, informing individuals about what data is collected and why. This practice not only respects user autonomy but also reduces the volume of sensitive data that needs protection.
- Transparency and Consent: Be open about how data is used. Create user-friendly privacy policies and notifications that clearly describe data practices. Where feasible, give individuals a choice to opt out of certain data uses. Transparency fosters trust and can alleviate public concerns about hidden or unauthorized data usage.
- Responsible Data Sharing: When sharing data externally, ensure that any shared dataset has been stripped of personal identifiers and that any potential risk of re-identification is minimized. Contracts or agreements should clearly outline acceptable uses and data protection obligations.
- Algorithmic Fairness: Regularly audit algorithms for bias by examining outcomes across different demographic groups. Bias can creep in through historical data, model assumptions, or design choices. By identifying biases early, organizations can take corrective steps and maintain equitable analytics.
- Ethical Review Boards: Consider setting up an internal review board that evaluates high-stakes analytics projects. Modeled after Institutional Review Boards (IRBs) in academic research, these committees assess potential harms, benefits, and alignment with ethical standards.
- Continuous Training and Awareness: Conduct regular workshops and training sessions to keep data analytics teams up to date on ethical considerations and privacy regulations. A well-informed workforce is your best defense against ethical lapses.
Organizations like Google have internal frameworks for assessing AI projects, which include privacy, security, and ethical criteria. Similarly, companies like IBM have published ethical principles guiding the design and deployment of artificial intelligence systems. These examples show that best practices are most effective when they are embedded into corporate culture rather than treated as add-ons or afterthoughts.
Implementing ethical analytics frameworks might mean adopting new documentation processes, updating training programs, or reorganizing governance structures to incorporate ethics oversight. However, these efforts pay dividends in terms of trust, reputational benefits, and risk mitigation. A robust and transparent approach to data ethics can become a unique selling point in a marketplace increasingly concerned with privacy and security.
6. The Future of Data Ethics in Analytics
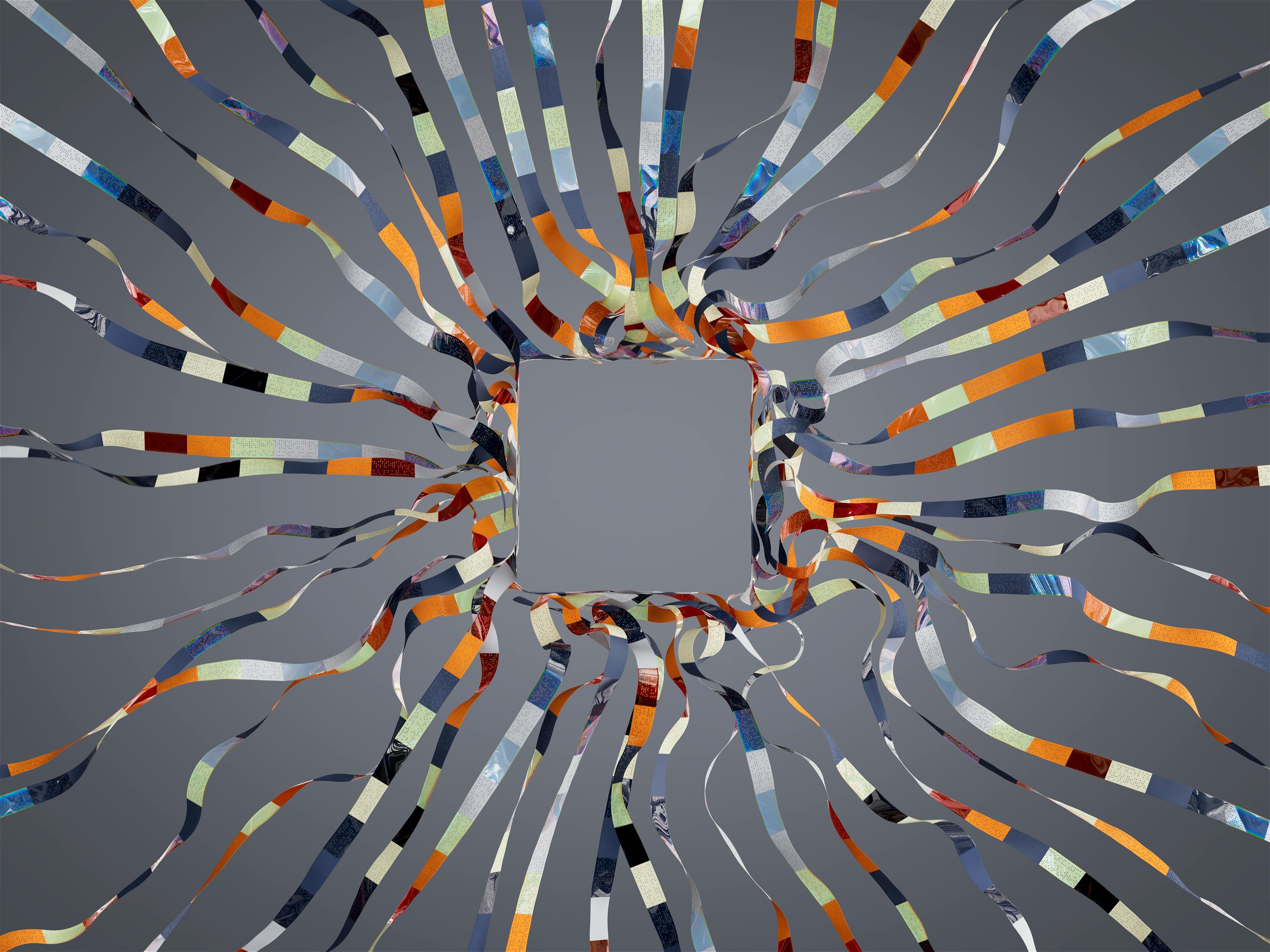
The rapid evolution of analytics technologies, especially in the realm of AI and machine learning, continues to shape new frontiers in Data Ethics in Analytics. As algorithms become more sophisticated, the potential for both positive impact and negative consequences grows exponentially. Indeed, AI-driven analytics can revolutionize healthcare, finance, and public services, but it also introduces concerns around data biases, unintended consequences, and the opaque nature of black-box models.
Emerging trends in data ethics include explainable AI (XAI), which aims to make machine learning models more transparent. Instead of offering a “black box” prediction, XAI techniques provide insight into how data was processed to reach a conclusion, potentially reducing the risk of hidden biases or unfair outcomes. This aligns with broader demands from regulators and the public for accountability in AI-driven decisions, whether those decisions impact hiring, lending, or law enforcement.
Privacy and compliance regulations are also likely to become more stringent, impacting the ways organizations handle global data flows. We can expect to see more jurisdictions enact GDPR-like laws, leading to a fragmented but heightened regulatory environment. Companies that operate internationally will need to invest in solutions that provide a consistent approach to data compliance across multiple regions, all while accommodating local variations in legal requirements.
Another emerging consideration is the ethical use of synthetic data. Synthetic data generation techniques can produce artificial datasets that mimic real-world data’s statistical properties, reducing risks of re-identification and privacy breaches. While this is promising, it also poses questions about whether synthetic data accurately represents reality, and how biases might persist or even magnify within these generated datasets.
Organizations preparing for the future of data ethics should adopt a proactive stance:
- Stay Informed: Keep abreast of new regulations, ethical guidelines, and industry best practices, adjusting policies accordingly.
- Invest in Technology: Explore privacy-enhancing technologies and explainable AI tools to stay ahead of emerging challenges.
- Build a Culture of Responsibility: Foster an environment where every team member, from leadership to entry-level data scientists, understands the criticality of ethical considerations.
- Engage Stakeholders: Collaborate with consumers, policymakers, and advocacy groups to shape transparent and inclusive data practices that can stand up to public scrutiny.
In many ways, the future of data ethics in analytics is a race between the sophistication of new technologies and the ability of organizations and regulators to keep up. Those who proactively establish robust ethical frameworks, adopt advanced privacy-preserving tools, and foster a culture of responsible innovation will be best positioned to thrive in this dynamic landscape.
Conclusion
As the world becomes increasingly interconnected and data-driven, the issues surrounding Data Ethics in Analytics grow more pressing. Balancing the pursuit of insights with the safeguarding of privacy and the observance of compliance regulations is both a strategic imperative and a moral responsibility. Organizations that fail to address these questions risk not only regulatory penalties but also eroding the trust of their customers, employees, and the broader society.
On the other hand, organizations that commit to ethical data use reap significant benefits. They strengthen customer loyalty, reduce legal risk, and foster an environment where employees feel empowered to innovate responsibly. Data ethics, when implemented correctly, is not a barrier to growth but rather a catalyst for sustainable, principled advancement in analytics.
Now more than ever, stakeholders—from executives to frontline data practitioners—must collaborate to create and enforce rigorous ethical guidelines. This involves developing transparent data governance structures, embracing privacy-preserving techniques, and ensuring compliance with a complex and evolving array of regulations. By prioritizing Data Ethics in Analytics, companies can lead the way in building a future where insight and integrity coexist, driving transformative innovations that respect individual rights and societal well-being.
Ultimately, the question is not whether to adopt ethical data practices, but how quickly and effectively organizations can do so. The time for action is now, and the decisions made today will shape the data-driven world of tomorrow.
References & Further Reading
- General Data Protection Regulation (GDPR): Official EU Site
- California Consumer Privacy Act (CCPA): California Office of the Attorney General
- Health Insurance Portability and Accountability Act (HIPAA): U.S. Department of Health & Human Services
- IBM's Principles for Trust and Transparency: IBM Policy Lab
- Google AI Principles: AI at Google
- FATE (Fairness, Accountability, Transparency, Ethics) in AI: Microsoft Research Project FATE
What next?
If you found this article valuable and want to deepen your understanding of big data analytics, explore the additional resources available on our website. Share your own experiences, challenges, or questions with us via the contact page — we’d love to hear from you.