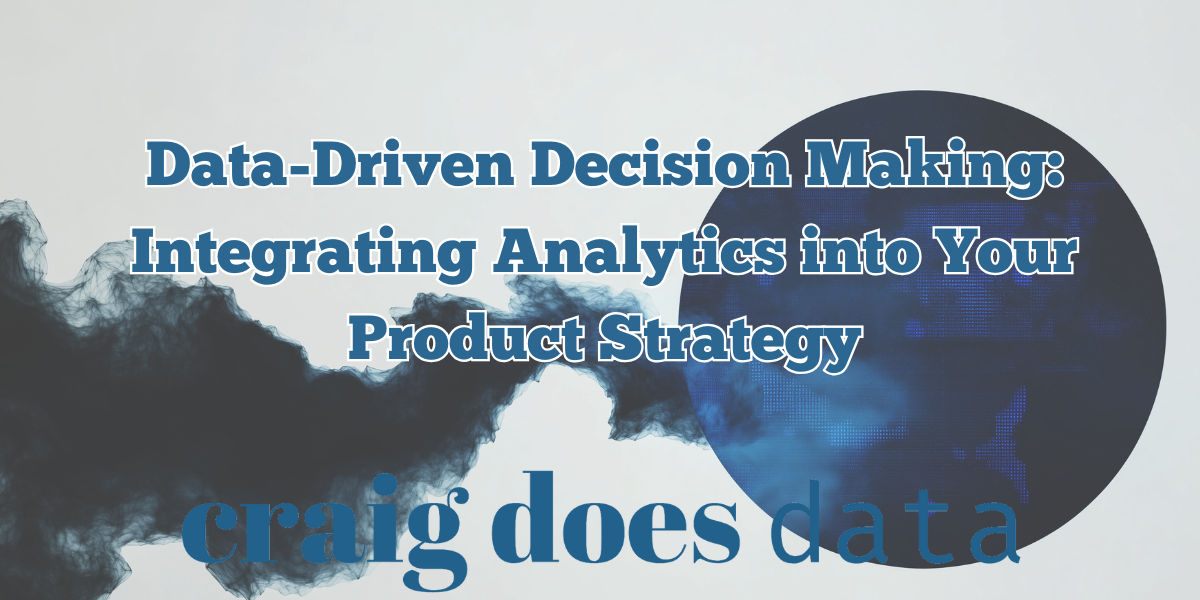
Data-Driven Decision Making: Integrating Analytics into Your Product Strategy
Introduction
Data-driven decision making has become a cornerstone of modern business practice. As markets grow increasingly competitive and customers become more discerning, relying on gut feelings alone is no longer enough. By systematically gathering, analyzing, and interpreting data, organizations can uncover patterns, test hypotheses, and ultimately deliver products that resonate with their target markets.
Integrating analytics into product strategy allows businesses to refine their offerings, target the right audiences, and measure success in a meaningful way. Through this deliberate approach, product teams can iterate quickly, reduce the likelihood of costly missteps, and create products that truly address user needs. Data analytics has also proven instrumental in forging closer connections between product development, marketing, sales, and customer support, aligning all parts of an organization around a central, data-informed vision.
In this article, we will walk you through the fundamental concepts and step-by-step processes of weaving data-driven decision making into your product strategy. From understanding the core principles of data analytics to exploring the different types of analytics and building a data-focused culture, you will gain insight into how to optimize product development and champion data throughout your organization. Whether you are new to the concept or seeking to refine an existing strategy, these sections will arm you with practical knowledge and tools to move forward with confidence.
1. Understanding Data-Driven Decision Making
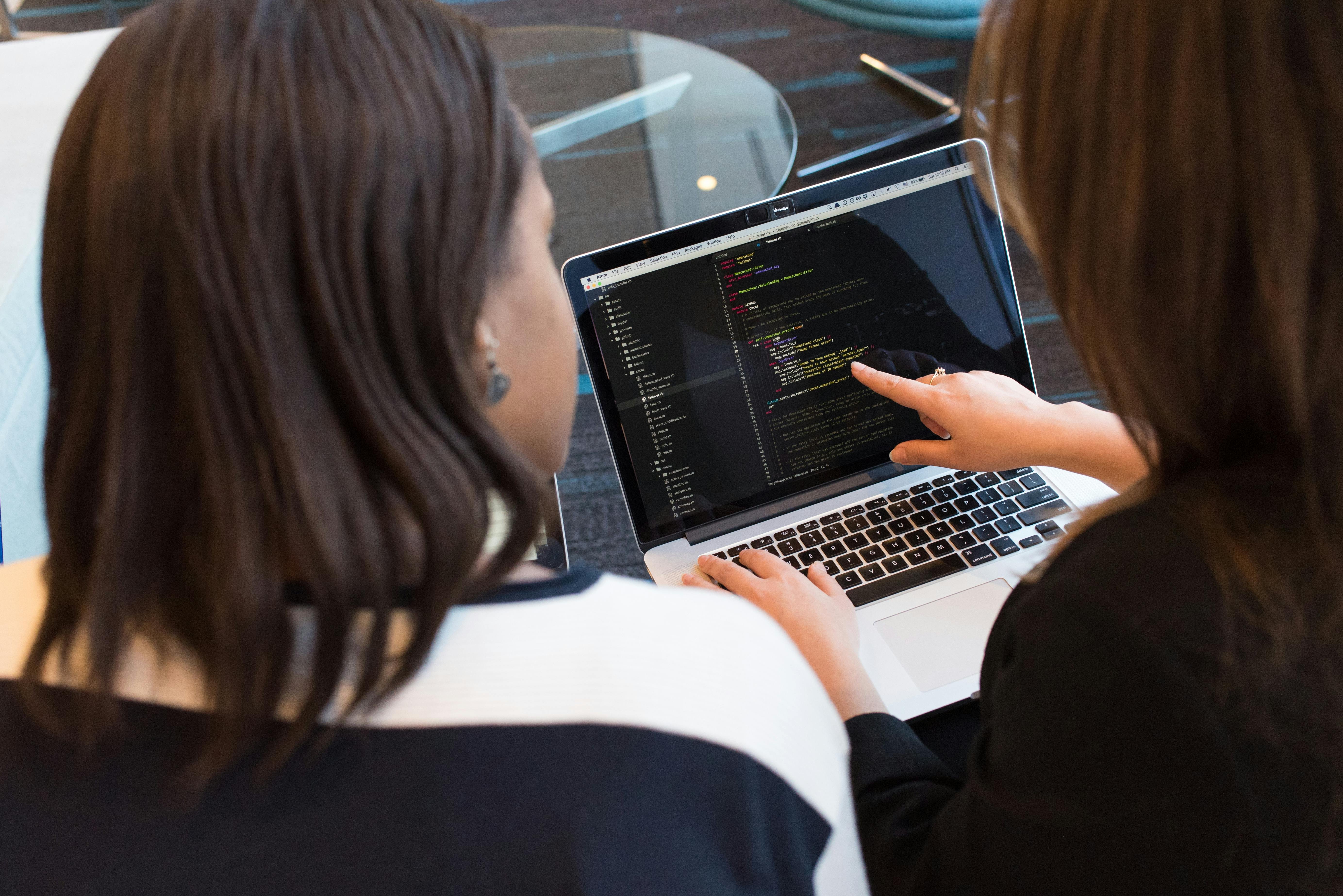
Data-driven decision making is the practice of basing strategic and operational choices on empirical evidence collected from various data sources. Rather than depending solely on intuition, past experiences, or hierarchical directives, data-driven organizations rely on quantifiable metrics and insights to inform their actions. At its core, this approach involves identifying the right questions, gathering relevant information, analyzing trends or patterns, and using these insights to shape decisions that drive organizational growth and innovation.
A modern business environment thrives on rapid change and digital transformation. In this climate, the importance of data analytics cannot be overstated. As customers interact with products and services across multiple touchpoints—from online platforms to brick-and-mortar storefronts—businesses are faced with an enormous influx of information. Websites track user clicks, time spent on pages, and conversion metrics; mobile apps record daily active users and in-app behaviors; and social media channels offer sentiment analysis, engagement metrics, and demographic data. The ability to synthesize these diverse inputs and derive actionable insights is what sets data-driven companies apart from their competitors.
A key component of a data-driven methodology is cyclical iteration: collect, analyze, act, and measure again. Initially, data is gathered from credible and relevant sources. It is then cleansed, organized, and analyzed using a variety of techniques—from exploratory data analysis and statistical modeling to sophisticated machine learning algorithms. The next phase is translating these analyses into action, whether that involves repositioning a product, tweaking a marketing campaign, or iterating on a user interface design. Finally, the outcomes of these actions are measured to see if they achieve the desired effect, and the entire cycle repeats with new or refined data.
Many high-performing companies illustrate the power of data-driven strategies. Consider Amazon, which constantly refines its recommendation engine based on user behaviors, purchases, and browsing patterns. This personalization not only boosts sales but also enhances the customer experience, fostering loyalty. Netflix’s content curation and original programming decisions are guided extensively by user data, leading to shows and movies that match audience preferences and significantly reduce the risk of costly flops. Across industries, from retail to finance, similar success stories highlight how data-based insights can minimize uncertainty, cut unnecessary expenses, and spark innovation.
In essence, adopting a data-driven mindset means placing validated data at the center of strategic thinking. It involves building an internal ecosystem where every stakeholder understands the significance of metrics, recognizes how to interpret them, and takes collective responsibility for harnessing this knowledge to move the business forward. The subsequent sections will delve deeper into how analytics can be seamlessly integrated into your product strategy and what concrete steps you can take to cultivate a truly data-oriented organization.
2. The Role of Analytics in Product Strategy
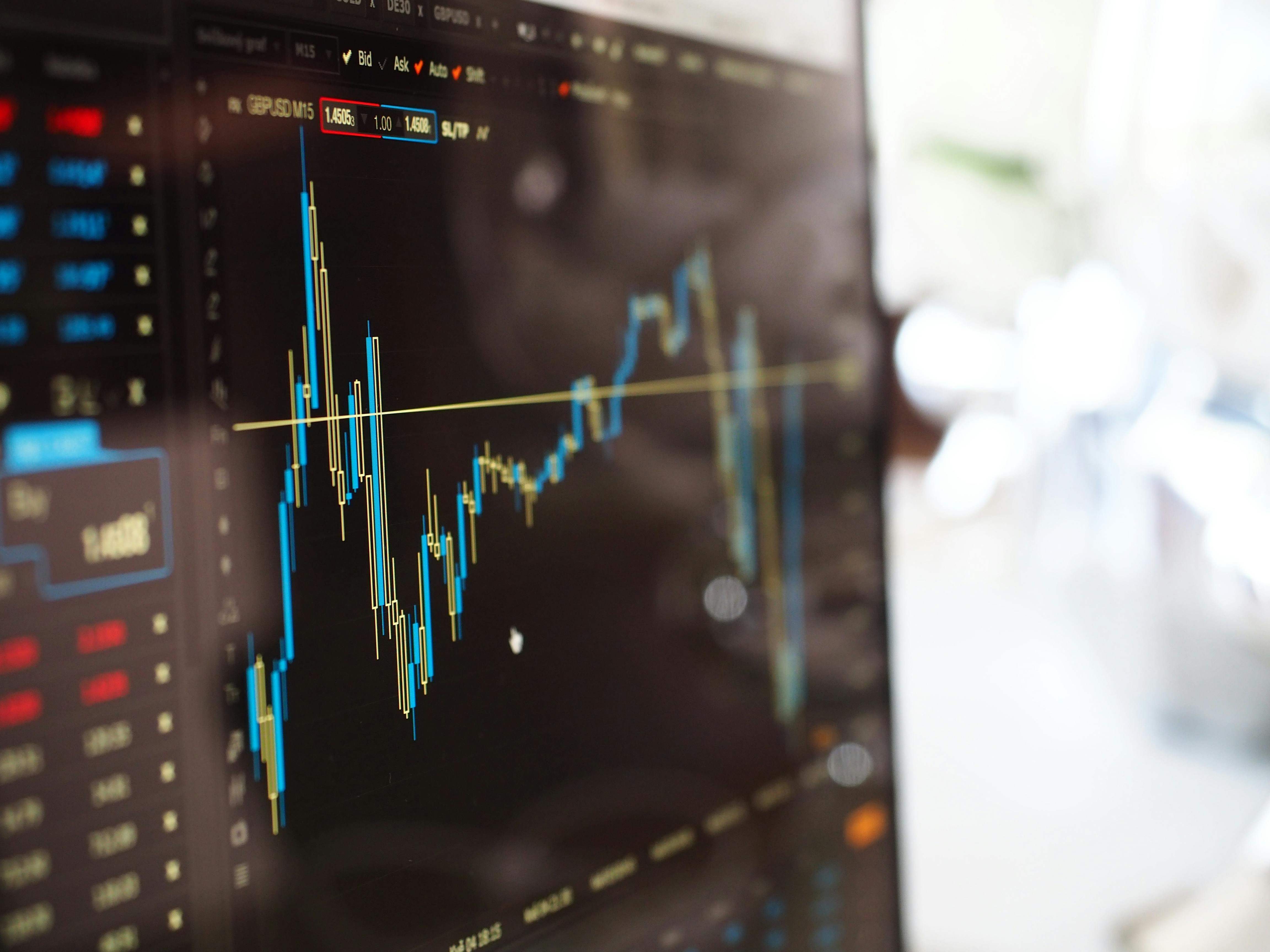
Analytics plays a pivotal role in shaping a product’s journey from its initial ideation to its eventual maturation and possibly even retirement. By embedding analytics into the product strategy, teams can gain clarity on critical questions: What do users truly want? How are they engaging with the product? Which features resonate, and which ones fall flat? Ultimately, the answers to these questions help allocate resources effectively, refine marketing messages, and tailor offerings for different segments of the market.
Understanding the various types of analytics—descriptive, diagnostic, predictive, and prescriptive—can unlock the full spectrum of insights. Descriptive analytics involves looking at past data to understand trends and patterns. For instance, analyzing web traffic data or user session durations can reveal which features of your product attract the most attention. Diagnostic analytics takes this a step further by asking “why?” If there was a spike in user churn last month, a diagnostic approach looks for the underlying causes, possibly tying them to a problematic update or a competitor’s launch.
Predictive analytics adds a forward-looking perspective, applying statistical models or machine learning algorithms to forecast future trends. This might include anticipating user growth in a certain region or predicting which new feature might deliver the highest return on investment. Finally, prescriptive analytics provides recommendations for action. Using simulations and optimization algorithms, it not only forecasts what might happen but also suggests the best course of action to achieve a desired outcome.
Consider how analytics helps in understanding market trends. By examining user demographics, engagement metrics, and purchasing behaviors, product teams can identify new opportunities. For example, if data reveals a significant portion of user growth coming from mobile platforms, the strategy might pivot to focus on improving mobile user experiences or developing features that cater to a smartphone-centric audience. Similarly, analytics can uncover evolving customer preferences by monitoring feedback channels, social media sentiment, and support tickets. If a surge in support requests centers around difficulties with a payment feature, it becomes clear that usability improvements are needed.
Post-launch, analytics remains crucial for ongoing optimization. Product performance metrics—like daily active users, retention rates, and net promoter scores—signal whether the product is on track or needs recalibration. Leveraging both quantitative data (e.g., conversion rates, usage stats) and qualitative inputs (e.g., user interviews, open-ended survey responses) empowers teams to refine marketing tactics, redesign user journeys, and deepen customer loyalty.
In sum, analytics is a guiding compass in formulating and continuously enhancing product strategy. It identifies market needs, pinpoints growth opportunities, and clarifies the rationale behind user behaviors. When employed effectively, analytics not only steers the product roadmap but also helps build a competitive advantage. In the following sections, we will explore how to create an organizational culture that embraces data, the best ways to collect relevant data, and the tools and techniques to transform raw numbers into meaningful, actionable insights.
3. Building a Data-Driven Culture
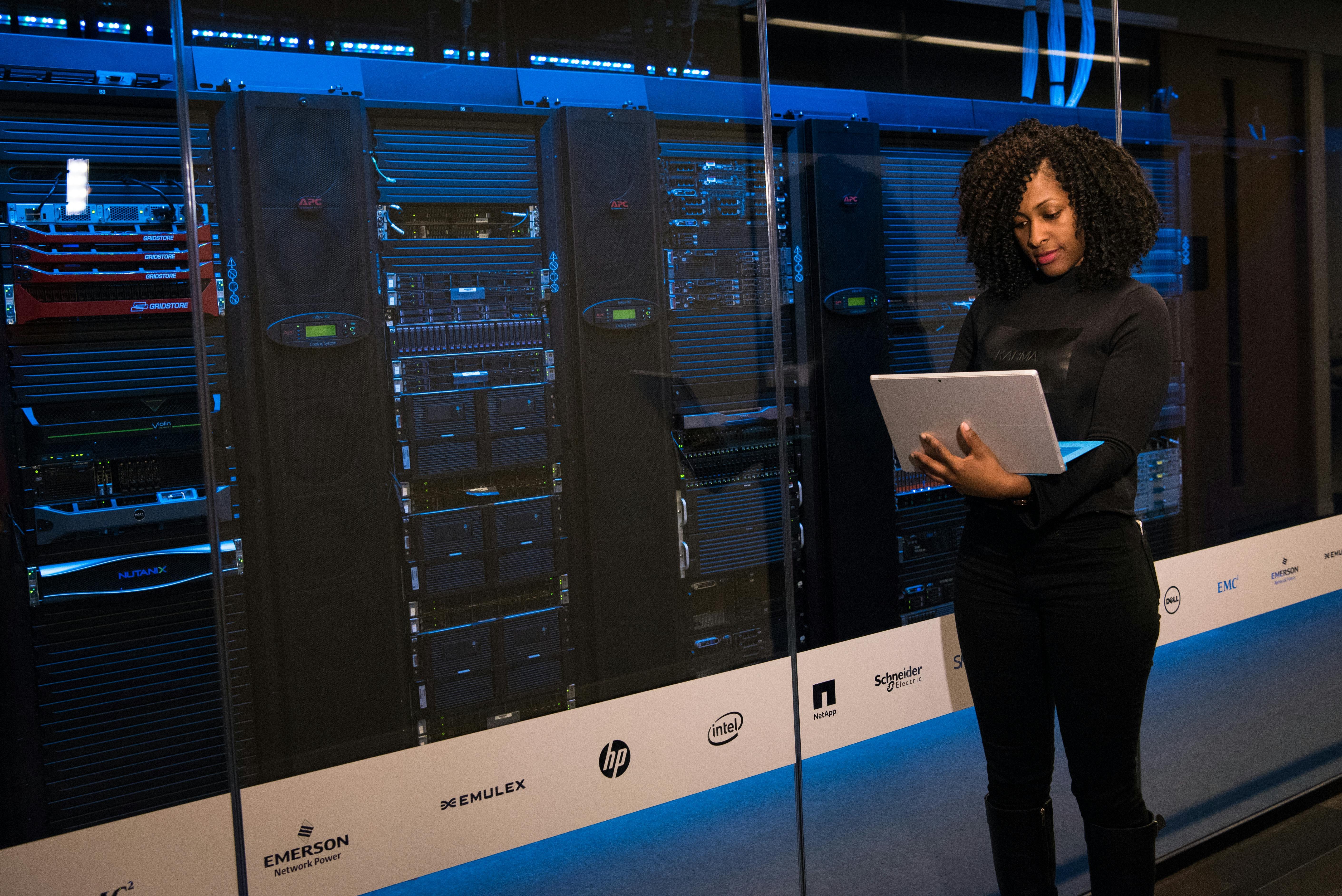
Adopting data-driven decision making is not simply a matter of installing new software or hiring data scientists; it requires a fundamental cultural shift. A data-driven culture is one in which every team member, from top leadership to entry-level employees, values data as a source of truth and leverages it for insights and decisions. This cultural ethos encourages curiosity, critical thinking, and continuous learning across all departments.
Leadership is key to cultivating a data-first mindset. When executives and managers demonstrate their reliance on data—citing metrics during meetings, using dashboards to track performance, and celebrating data-based successes—it sets a tone that resonates throughout the organization. For instance, hosting regular “data review” sessions where teams present insights encourages transparency and recognizes the importance of quantitative evidence in shaping strategy. When employees observe these practices, it fosters trust in data and a willingness to invest the time necessary to understand metrics.
Data literacy is another cornerstone of a data-driven culture. It's essential to provide training and resources that empower employees to understand and work with data effectively. This training can range from teaching basic spreadsheet skills to offering courses in data visualization and statistics, depending on the nature of the roles involved. Cross-functional workshops can also break down silos by teaching non-technical teams how to interpret data analyses presented by data scientists. Encouraging open communication between data experts and domain experts ensures that insights are accurately contextualized and actionable.
It’s also important to create a supportive environment where experimentation is encouraged. In a data-driven culture, failure can be reframed as a learning opportunity, provided it’s approached methodically. This might involve running A/B tests, refining hypotheses, and analyzing outcomes to either pivot quickly or double down on effective tactics. Teams that know they can experiment without fear of blame are more likely to surface innovative ideas.
Building this culture, however, is an ongoing effort that requires consistency and patience. Organizations must continuously reinforce data-driven ideals by integrating metrics into performance reviews, celebrating data-centric achievements, and maintaining accessible systems for sharing insights. Over time, these practices help solidify a collective mindset that values rigorous analysis and fosters a shared sense of responsibility for the company’s success.
4. Collecting the Right Data
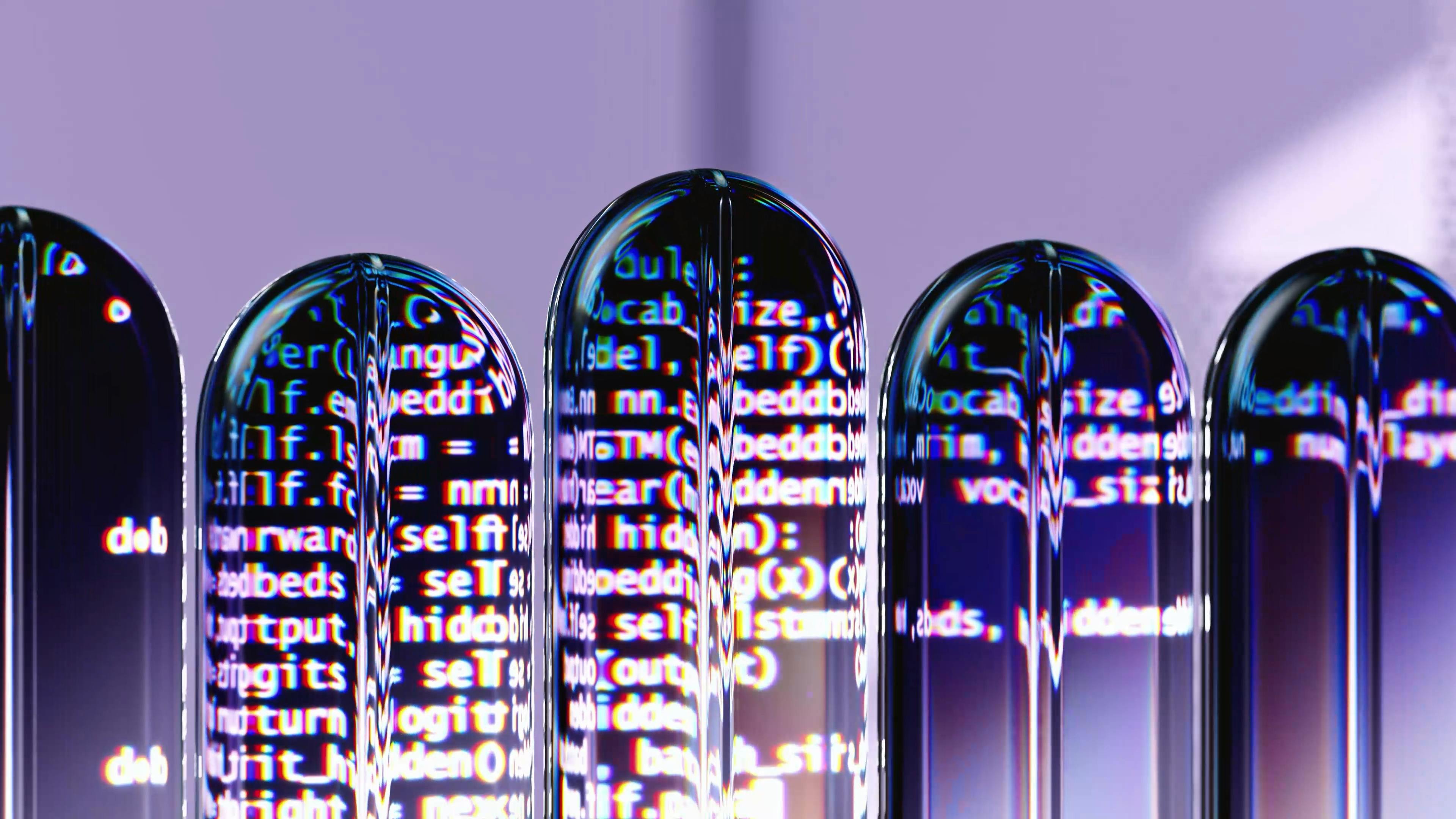
Collecting data effectively is the bedrock of any data-driven organization. Without the right data, even the most sophisticated analytics tools and methodologies can yield misleading or incomplete insights. The first step is identifying the specific questions you aim to answer. Are you trying to understand user behavior, track conversion rates, measure feature adoption, or gauge brand sentiment? Defining clear objectives guides the selection of relevant metrics and helps avoid the common pitfall of collecting excessive, unfocused data.
Data sources vary widely, and each can bring unique perspectives to your analysis. Customer feedback, gathered through channels such as surveys, support tickets, or social media comments, can illuminate pain points or desired features. Sales data provides insight into purchase behaviors, revenue patterns, and seasonal trends. Web and mobile analytics reveal how users navigate your product, where they drop off, and which elements draw the most engagement. Meanwhile, market research, including competitor analyses and industry reports, contextualizes your product within a larger ecosystem, helping you spot opportunities and threats.
While quantity is valuable, data quality is paramount. Accurate, clean data helps ensure that any subsequent insights are reliable. If datasets are riddled with inaccuracies, duplicates, or inconsistencies, the risk of making flawed decisions increases drastically. Establishing strict data governance policies can mitigate these risks. This includes defining data entry standards, regularly auditing data for errors, and implementing robust validation procedures. For example, an e-commerce platform might require specific formats for phone numbers and mailing addresses to avoid confusion and reduce the potential for data corruption.
Integrity also matters. Data ethics and privacy regulations—such as GDPR in Europe or CCPA in California—are increasingly critical factors. Collecting user data ethically fosters trust, a vital commodity for businesses. Always be transparent about what data is collected, how it will be used, and ensure that you have secured proper consent. Moreover, implementing data encryption and other security measures is essential to safeguarding sensitive information.
Gathering the right data, in the right ways, sets the foundation for meaningful analysis. When teams know exactly what questions they are trying to answer and have confidence in the integrity of the data, they can efficiently turn raw figures into actionable insights. In the following sections, we will examine how to utilize an array of analytical tools and technologies to uncover these insights and drive product-focused decisions.
5. Tools and Technologies for Data Analytics
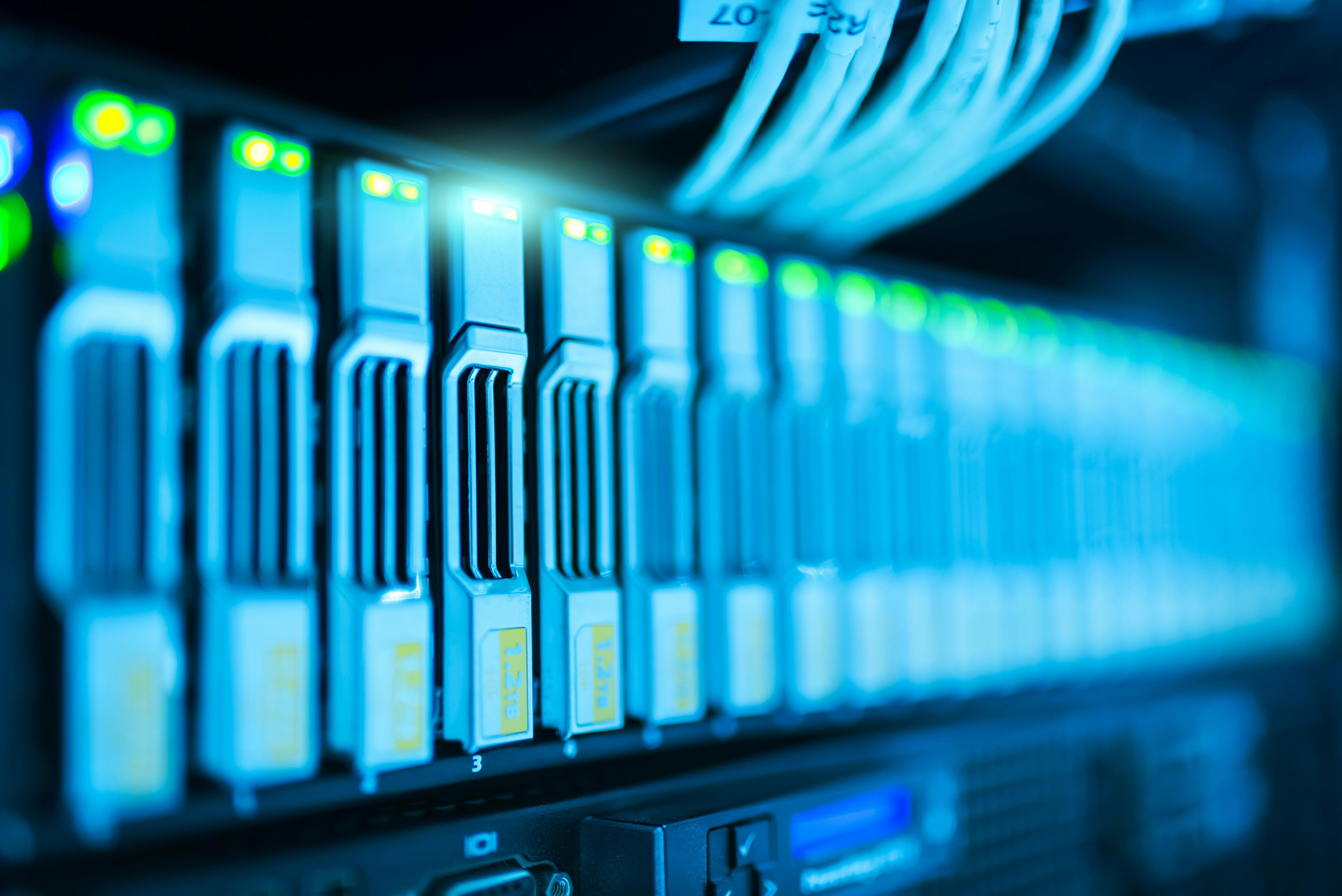
The landscape of data analytics tools and technologies has expanded exponentially in recent years. Whether you’re a startup exploring cost-effective solutions or a large enterprise seeking robust, scalable platforms, there are tools designed to fit every stage of your data journey. Selecting the right mix depends on your specific needs, budget, and the skill sets of your team.
A foundational tool for many organizations is a business intelligence (BI) platform. Solutions like Tableau, Power BI, and Looker provide user-friendly interfaces for data visualization and reporting, making it easier for non-technical stakeholders to interact with complex datasets. These platforms excel at creating interactive dashboards where users can filter and drill down into data, identify trends, and share insights across teams.
For those who require deep statistical analysis or custom algorithms, programming languages like Python and R are indispensable. Python’s rich ecosystem of libraries—such as pandas for data manipulation, NumPy for numerical computations, and scikit-learn for machine learning—makes it a versatile choice, and the most widely-used option in industry and business. R, on the other hand, is particularly favored in academic and research settings for its strong statistical analysis capabilities and breadth of packages for specialized tasks. Both languages integrate well with a range of data storage and visualization frameworks.
Web analytics tools like Google Analytics, Mixpanel, and Amplitude are designed to track user behavior on digital products. By implementing tracking codes or software development kits (SDKs), product teams can gather real-time information on user journeys, conversion funnels, and retention rates. Google Analytics is particularly popular for its user-friendly interface and seamless integration with other Google services. Mixpanel and Amplitude, meanwhile, offer more granular capabilities for product analytics, such as user segmentation and sophisticated cohort analysis.
Data pipelines and warehousing solutions form the backbone of large-scale analytics initiatives. Tools like Apache Kafka, AWS Glue, or Airflow can handle the extraction, transformation, and loading (ETL) of data from multiple sources into centralized data warehouses such as Amazon Redshift, Google BigQuery, or Snowflake. These platforms are designed to store massive volumes of structured and unstructured data while enabling fast query performance. For organizations heavily invested in the modern data stack, using dbt (data build tool) can standardize transformation logic and version control, ensuring data consistency and reproducibility.
When selecting analytics tools, it’s vital to consider scalability, ease of integration, and the learning curve for your team. A smaller organization might opt for user-friendly, out-of-the-box solutions that don’t require specialized expertise, while enterprises might lean toward highly configurable systems that can handle vast data volumes and complex workflows. Ultimately, the best approach is often a hybrid one, combining dashboards for quick insights with powerful scripting languages for advanced analysis. In the next section, we’ll discuss how to translate data from these tools into actionable insights that drive real change in your product strategy.
6. Analyzing Data for Insights
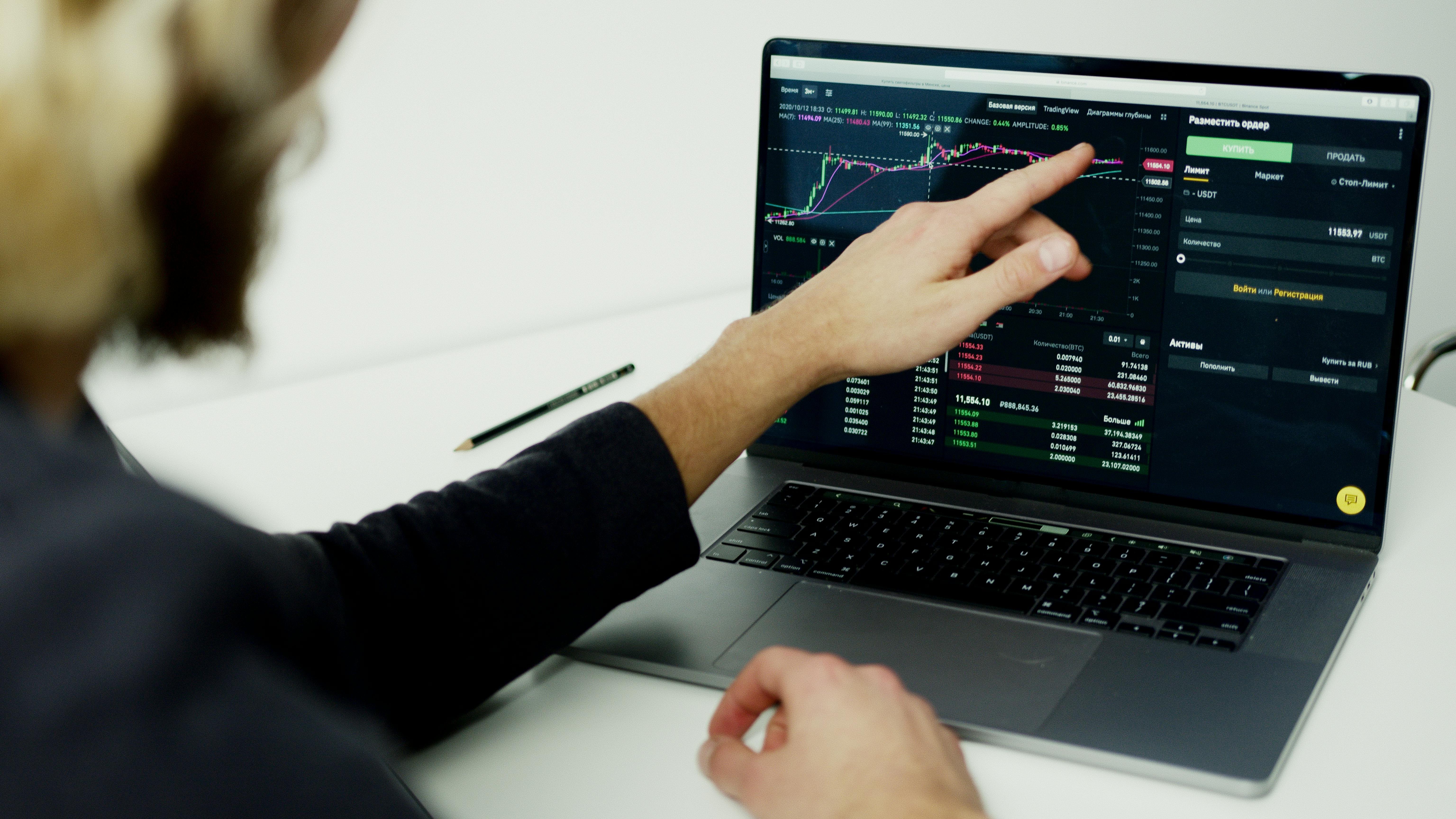
Having robust data analytics tools is only half the battle; the true value emerges when you skillfully interpret the data to inform strategic decisions. Analyzing data for insights involves sifting through tables, charts, and statistics to pinpoint the information that is most relevant to your objectives. Whether you are a product manager focused on feature adoption or a marketing executive tracking conversion pipelines, understanding how to glean meaningful narratives from data is crucial.
A structured analysis typically begins with exploratory data analysis (EDA). During EDA, analysts use descriptive statistics, data visualizations, and correlations to get a basic understanding of the data’s shape, outliers, and key trends. For instance, imagine you have a dataset of user sign-up information for a mobile application. Plotting the distribution of sign-up dates might reveal seasonal peaks, while calculating average usage times could highlight differences in behavior between weekday and weekend users. By systematically exploring these patterns, you can uncover insights that guide more nuanced investigations.
Data storytelling transforms these findings into compelling narratives. While a spreadsheet filled with figures and pivot tables can be useful, many stakeholders respond better to visual and contextual cues. This is where data visualization tools shine. Graphs, heatmaps, and dashboards can highlight anomalies, trends, and relationships in ways that are intuitive. Coupling visuals with clear annotations and succinct explanations ensures that the insights are accessible to audiences with varying levels of technical expertise.
Actionable insights connect analytical findings to concrete steps. For instance, let’s say your analysis reveals that users drop off after initiating the checkout process in your e-commerce store. An “actionable insight” might be: “Improve the checkout form’s user interface to reduce friction and cart abandonment.” With this insight, your product team can hypothesize solutions—perhaps simplifying the number of steps, adding progress indicators, or offering multiple payment options—and then test these changes in a controlled environment like an A/B test.
Analyzing data is also an iterative endeavor. As changes are implemented, the data shifts, revealing new opportunities and challenges. This “feedback loop” allows you to validate or refine hypotheses continually. For example, if the new checkout flow reduces cart abandonment by 20%, you might expand these improvements to additional product lines or platforms. If it has little effect, you re-examine the data, refine your hypothesis, and test again.
The skills required for effective data analysis are both technical and conceptual. Technical proficiency in analytics tools is necessary to handle large datasets, construct queries, or build predictive models. Conceptual skills in critical thinking and domain expertise are equally essential to frame the right questions and interpret results correctly. When these skills converge, businesses can move beyond surface-level metrics to unearth deep insights that substantially influence product direction and performance.
7. Implementing Data-Driven Strategies
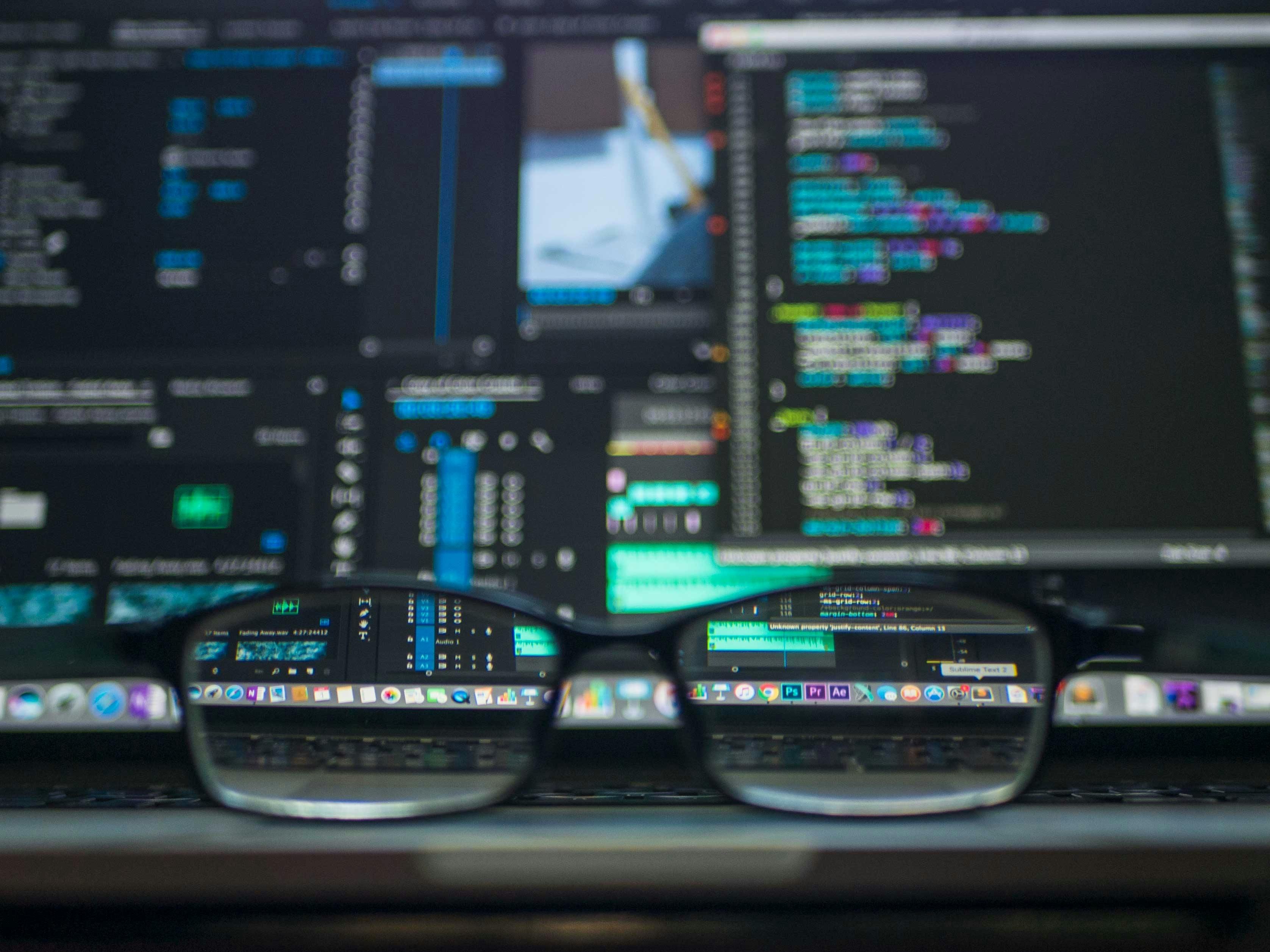
Data-driven decision making becomes truly transformative once insights are operationalized into real strategies. This involves translating analytical findings into product roadmaps, development sprints, marketing campaigns, and other tangible business activities. A common framework for implementation is to begin with a clear objective, align teams around that objective, deploy data-informed initiatives, and then measure outcomes to confirm success or reveal areas for improvement.
An agile methodology is particularly suited for embedding data-driven strategies into your workflow. By working in short development cycles—or “sprints”—teams can incorporate new insights rapidly, iterate on features, and measure impact. Suppose your data analysis indicates that adding social sign-in options will increase sign-up rates by simplifying the process. You could create a user story for this feature, prioritize it in the next sprint, and deliver a minimum viable version to a subset of users. After collecting feedback and usage data, you refine or expand the feature in subsequent sprints. This approach reduces risk by preventing large-scale rollouts of untested ideas.
Communication is critical in this phase. Product managers, data analysts, designers, and developers should collaborate closely to ensure that every decision is grounded in empirical evidence. Regular stand-up meetings, sprint reviews, and retrospectives provide opportunities to share insights, address questions, and make swift pivots if the data shows the need for course correction. Transparent documentation—like a centralized wiki or project management board—helps maintain alignment across distributed teams and serves as an audit trail for how decisions were reached.
Real-world case studies exemplify the power of data-driven execution. For instance, a software-as-a-service (SaaS) company might discover through analytics that most trial users drop off before fully exploring the product’s features. The team decides to rework the onboarding flow, emphasizing interactive tutorials and guided tours. After implementing these changes, they measure usage patterns and compare them to the original dataset, often witnessing significant lifts in trial-to-paid conversion rates. This validated success can inspire further data-driven initiatives, such as refining pricing strategies or introducing new subscription tiers for different customer segments.
Another example might involve a mobile gaming company that sees unusually high user retention for a specific demographic. By digging deeper, they discover that a certain character skin or game mechanic resonates strongly with these users. The product team then decides to double down on similar mechanics or aesthetics for future updates, aligning marketing campaigns and development resources to capitalize on the discovered preference. Over time, the consistent alignment of data insights with product decisions solidifies a culture of continuous improvement, driving both user satisfaction and revenue.
Implementing data-driven strategies is not a one-off endeavor, but an evolving practice that adapts to shifting market conditions and technological changes. As new insights surface and user expectations evolve, product teams must remain nimble, ready to pivot and optimize. By making analytics a central part of every product decision, organizations can reduce uncertainty, better meet customer needs, and maintain a competitive edge.
8. Overcoming Challenges in Data-Driven Decision Making
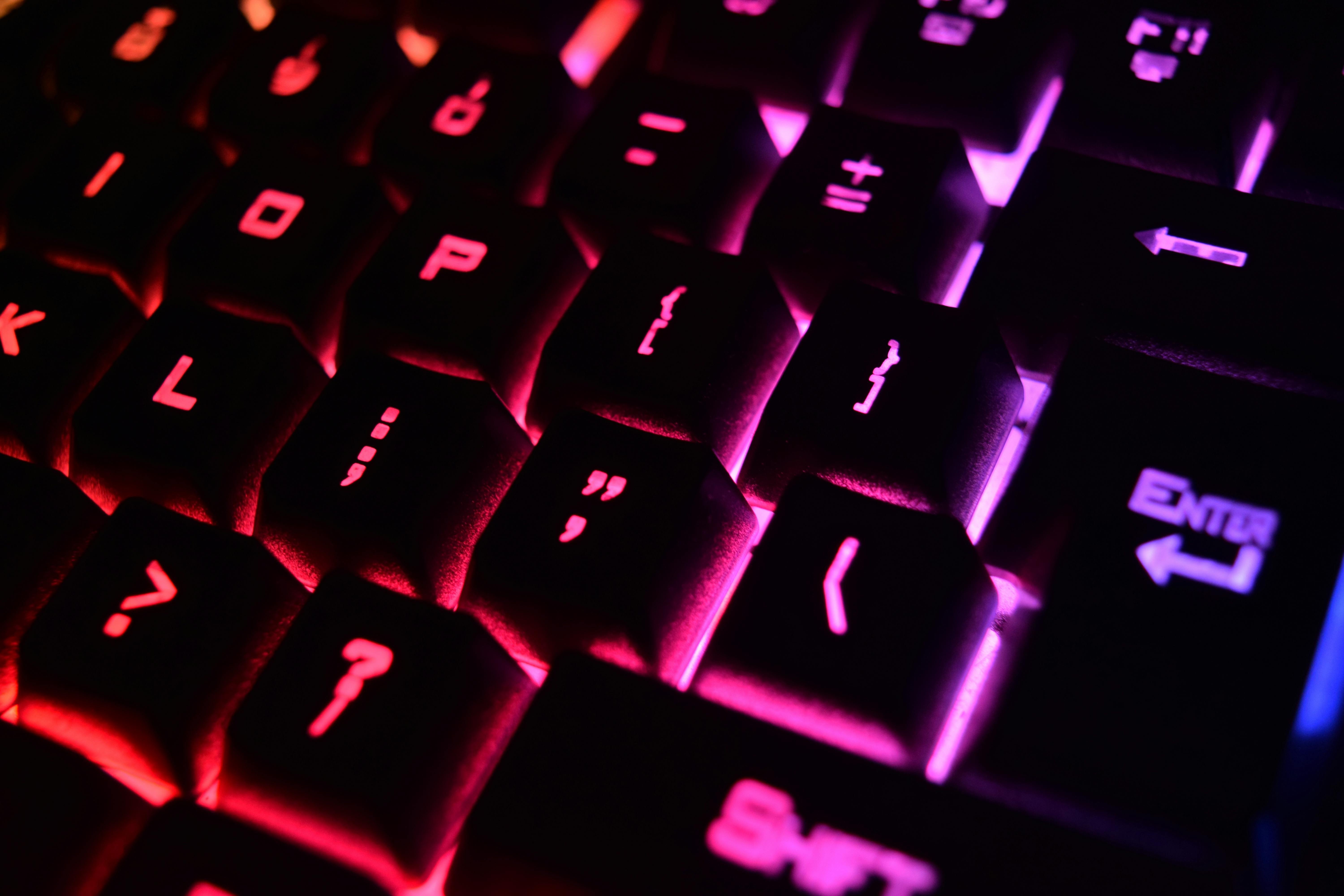
Despite its many advantages, transitioning to a data-driven approach comes with its fair share of challenges. Common obstacles include data privacy concerns, organizational silos, insufficient technical expertise, and resistance to cultural change. Recognizing these potential roadblocks early on and proactively devising strategies to address them can mean the difference between a smooth adoption and a stalled initiative.
Data privacy and compliance concerns have grown significantly in recent years. Organizations collecting user data must abide by regulations such as GDPR (General Data Protection Regulation), CCPA (California Consumer Privacy Act), and others specific to their local jurisdictions. Failing to comply can result in substantial fines and a damaged reputation. To overcome these challenges, companies should implement robust data governance frameworks and ensure that all data collection and handling processes are transparent and secure. By making privacy a foundational pillar of the data strategy, you not only safeguard your organization legally but also build trust with customers.
Another frequent issue is the existence of data silos, where different departments or units store their data in disconnected systems. These silos hamper holistic analysis, as no single source of truth exists. Overcoming this often requires a concerted effort to integrate data flows, possibly by establishing a centralized data warehouse or adopting a data lake approach that unifies structured and unstructured datasets. Encouraging cross-departmental collaboration and standardizing data formats can also break down these silos.
Resistance to change is equally challenging, particularly in organizations where decisions have historically been made based on experience, tradition, or the highest-paid person’s opinion (the “HiPPO” effect). Managers might worry that a data-driven approach undermines their authority, or employees might fear that analytics will expose performance issues. Addressing these concerns calls for clear communication of the benefits, training programs to increase data literacy, and assurance that data-driven insights complement, rather than replace, professional judgment.
Finally, maintaining momentum is crucial. Adopting a data-driven mindset is not an overnight transformation but a continuous journey. Initial excitement can wane if the organization doesn’t see quick wins or if teams revert to old habits when deadlines loom. One way to sustain progress is to celebrate small victories—such as a successful pilot project, a well-received dashboard, or an improvement in a key metric—and showcase the tangible impact of data-driven decisions. Regular training and resource allocation can keep the workforce skilled and motivated to engage with analytics tools.
By proactively identifying and addressing these challenges, you can smooth the path toward creating a truly data-focused organization. Whether it’s implementing privacy safeguards, tearing down data silos, or nurturing a culture that values evidence-based insights, each step helps entrench data-driven practices as a natural and enduring part of the company’s DNA.
Conclusion
Throughout this article, we have explored the fundamentals of data-driven decision making and its powerful influence on product strategy. We examined the essential roles of data collection, analysis, and organizational culture in creating an environment where insights can emerge naturally. From understanding the nuances of descriptive, diagnostic, predictive, and prescriptive analytics to implementing agile cycles and using data to guide product roadmaps, the journey to becoming truly data-driven is both transformative and rewarding.
By integrating analytics at every stage of product development—from ideation to iteration and beyond—companies can unlock efficiency, foster innovation, and gain a meaningful competitive advantage. Data not only validates hypotheses but also uncovers hidden opportunities, allowing you to tailor products to evolving customer needs and market conditions. The collaborative effort of leadership, data specialists, product teams, and all stakeholders ensures that evidence-based insights guide every significant move.
If you’re on the cusp of your data-driven journey, remember that consistent effort, open communication, and a willingness to adapt are crucial. Embracing the right tools, nurturing a culture of curiosity and accountability, and methodically addressing challenges can set you on a path to sustained success. As the world continues to change, so too will the data that informs your decisions. By staying agile and committed to continuous learning, you and your team can confidently navigate the future, guided by the clarity that only data can provide.
Now is the time to begin integrating analytics into your product strategy. Whether you’re a startup seeking your first data insights or a mature enterprise looking to refine an existing infrastructure, the principles in this guide can serve as a compass. The key is to start somewhere—take a small step, measure it, learn from it, and keep building. Over time, those incremental steps converge, shaping a robust, thriving data-driven organization.
Additional Resources
Below is a curated list of resources to help deepen your understanding of data-driven decision making and product strategy. These include books, online courses, and case studies from leading organizations that have successfully integrated analytics into their business models. By exploring these resources, you can expand your skill set, gain fresh perspectives, and stay current with emerging best practices in this rapidly evolving field:
-
Books:
- The Lean Startup by Eric Ries – A seminal work on using data to drive continuous product iteration.
- Data Science for Business by Foster Provost & Tom Fawcett – An excellent primer on extracting value from data.
- Lean Analytics by Alistair Croll & Benjamin Yoskovitz – A deep dive into metrics-driven decision making.
-
Online Courses & Tutorials:
- Coursera’s “Data-Driven Decision Making” specializations – Various modules on statistical methods, machine learning, and business analytics.
- Udemy’s “Data Visualization with Tableau” – Hands-on training to create interactive dashboards.
- Google’s “Analytics Academy” – In-depth tutorials on Google Analytics for web and app tracking.
As you dive into these materials, remember that the end goal is to integrate knowledge into actionable steps. Keep refining your approach to data collection, analysis, and organizational culture. Seek out mentors or professional guidance if needed, and continuously revisit your strategy to ensure it remains relevant in a dynamic market. With the right mindset and resources, you can sustain a data-driven framework that evolves alongside your products, your team, and your customers.
What next?
If you found this article valuable and want to deepen your understanding of big data analytics, explore the additional resources available on our website. Share your own experiences, challenges, or questions with us via the contact page — we’d love to hear from you.