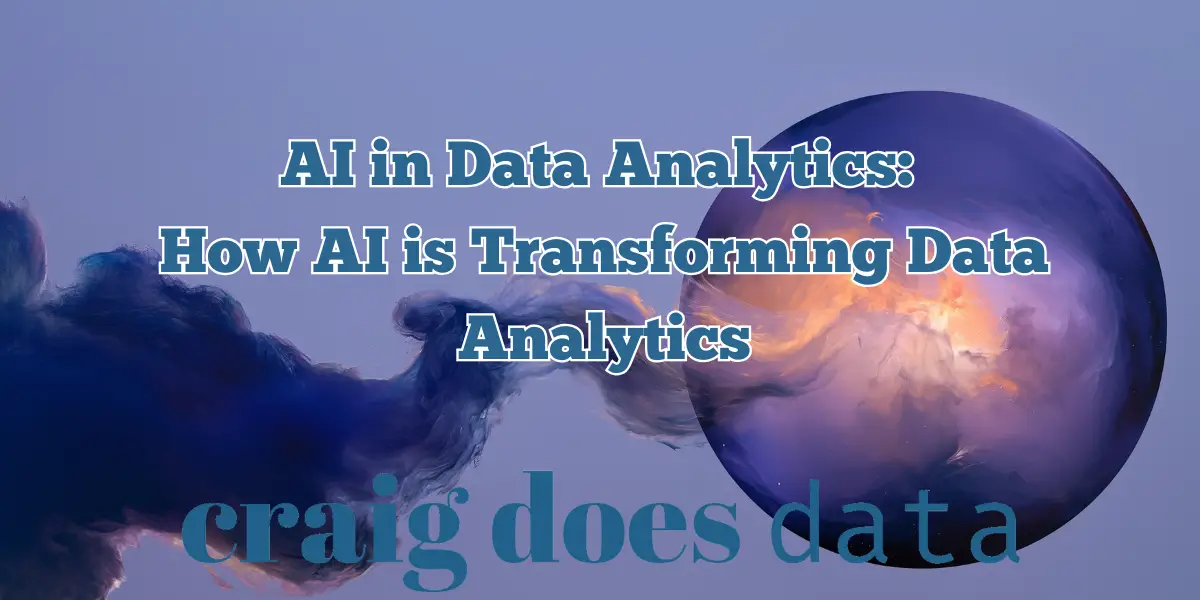
AI in Data Analytics: How AI is Transforming Data Analytics
In today's rapidly evolving business landscape, data is not just information—it's the lifeblood of informed decision-making, strategic innovation, and competitive advantage. Data analytics, the process of examining raw data to draw conclusions about that information, has become indispensable for businesses across all sectors. From understanding customer behavior to optimizing operational processes, the insights derived from data analytics are pivotal. However, traditional data analytics methods are increasingly challenged by the sheer volume, velocity, and variety of data generated in the digital age. This is where Artificial Intelligence (AI) steps in, revolutionizing the field and unlocking unprecedented potential.
AI is no longer a futuristic concept; it's a present-day reality reshaping data analytics in profound ways. By automating complex tasks, uncovering hidden patterns, and making predictions with remarkable accuracy, AI is augmenting human capabilities and transforming how businesses interact with their data. This guide will delve into the transformative role of AI in data analytics, exploring the key technologies, benefits, practical applications, and implementation strategies that are empowering organizations to harness the full power of their data. Whether you are a seasoned data analyst, a business leader, or simply curious about the future of data, this comprehensive exploration will provide you with valuable insights into how AI is not just enhancing, but fundamentally redefining data analytics.
In the following sections, we will embark on a journey to understand the synergy between AI and data analytics. We will begin by laying a foundation with the basics of data analytics and the challenges it faces, then explore the rise of AI and its core technologies that are driving this transformation. We will uncover the tangible benefits AI brings to businesses, illustrated with real-world case studies, and provide a practical roadmap for implementing AI in your own data analytics frameworks. Finally, we will address the important considerations and look towards the exciting future of AI in this dynamic field. Join us as we unpack the intricacies of AI in data analytics and discover how it's paving the way for a smarter, more data-driven world.
1. Understanding Data Analytics
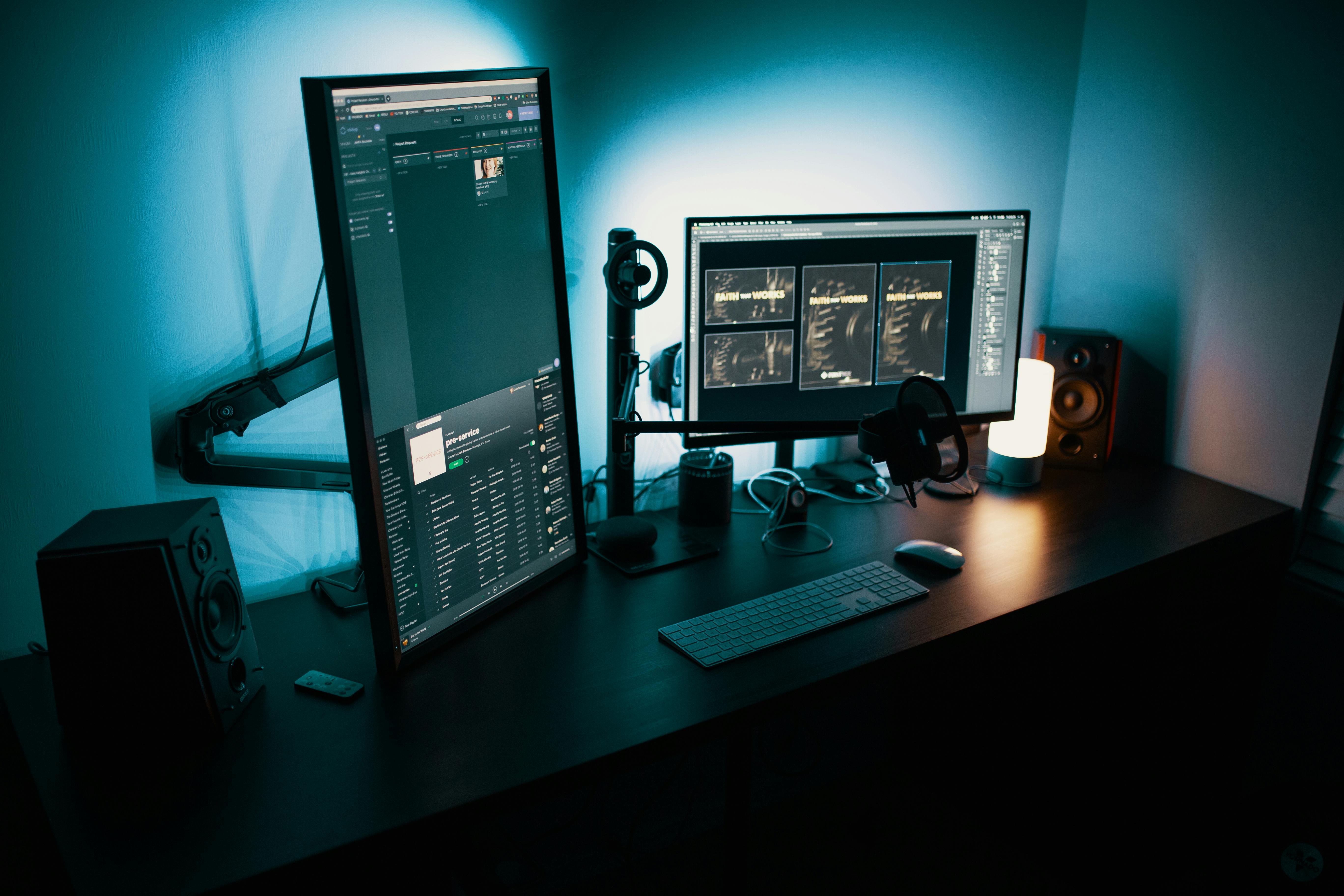
Data analytics is the science of analyzing raw data to make informed decisions. It involves a range of techniques and processes to extract insights, identify patterns, and derive meaningful conclusions from data. For businesses, data analytics is crucial because it provides the evidence-based understanding needed to optimize operations, improve customer experiences, identify new opportunities, and mitigate risks. In essence, it transforms data from a passive record of activities into an active tool for strategic and tactical advantage.
Traditional methods of data analysis have long been the backbone of business intelligence. These methods typically fall into four main categories:
- Descriptive Analytics: This is the most basic form of analytics, focused on summarizing past data to understand what has happened. Techniques include data aggregation, data mining, and basic statistics to describe historical trends and patterns. For example, generating reports on sales figures, website traffic, or customer demographics.
- Diagnostic Analytics: Going a step further, diagnostic analytics aims to understand why something happened. It involves looking at historical data to determine the causes of past events. Techniques often include data discovery, correlation analysis, and drill-down analysis to identify the root causes of observed patterns. For instance, investigating why sales declined in a particular quarter or understanding the reasons behind a spike in customer churn.
- Predictive Analytics: This type of analytics focuses on forecasting future outcomes based on historical data and statistical models. It uses techniques like regression analysis, time series analysis, and forecasting models to predict trends and future probabilities. Examples include predicting future sales volumes, forecasting customer demand, or estimating the likelihood of equipment failure.
- Prescriptive Analytics: The most sophisticated form of traditional analytics, prescriptive analytics not only predicts what will happen and why but also suggests actions to take to achieve desired outcomes. It uses optimization and simulation algorithms to recommend the best course of action. For example, recommending pricing strategies to maximize revenue, suggesting optimal inventory levels, or advising on the best marketing campaigns to run.
Despite their value, traditional data analytics methods face significant challenges in today's data-rich environment. These challenges include:
- Volume and Velocity of Data: The explosion of data from various sources – social media, IoT devices, online transactions, and more – has created massive datasets that are often too large and fast-moving for traditional methods to handle efficiently. Analyzing petabytes or exabytes of data in real-time is beyond the scope of many conventional tools and techniques.
- Complexity and Variety of Data: Data today comes in diverse formats – structured, semi-structured, and unstructured – including text, images, video, and audio. Traditional analytics tools are often designed to work primarily with structured data and struggle to effectively process and integrate the variety of data types now available.
- Time and Resource Constraints: Traditional analytics processes can be time-consuming and resource-intensive, often requiring manual data preparation, complex statistical modeling, and expert interpretation. This can lead to delays in insight generation and limit the ability to respond quickly to changing business conditions.
- Scalability Issues: As businesses grow and data volumes increase, traditional analytics infrastructure and methods may not scale effectively. Scaling up hardware, software, and human expertise to handle ever-larger datasets can be costly and complex.
- Human Bias and Error: Traditional analytics relies heavily on human expertise for data interpretation and model building. This introduces the potential for human bias and errors, which can affect the accuracy and reliability of insights. Subjectivity in data interpretation and model selection can lead to flawed conclusions.
These challenges highlight the limitations of traditional data analytics in the face of modern data complexities. The need for more sophisticated, automated, and scalable solutions has paved the way for the integration of Artificial Intelligence into data analytics, offering a powerful evolution to overcome these hurdles and unlock new frontiers in data-driven decision-making. The subsequent sections will explore how AI is rising to meet these challenges and transform the landscape of data analytics.
2. The Rise of AI in Data Analytics
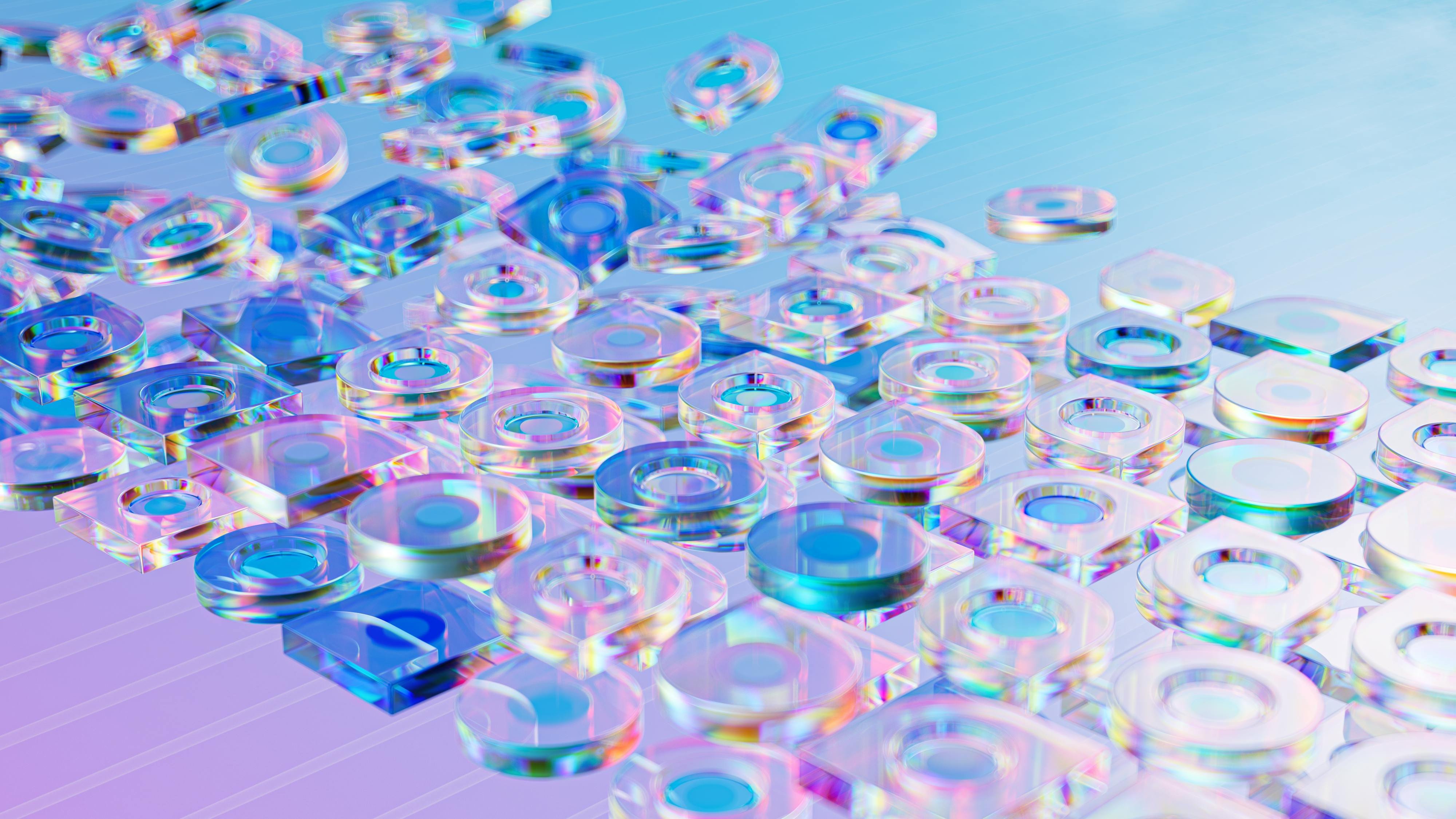
Artificial Intelligence (AI) is broadly defined as the capability of a machine to imitate intelligent human behavior. In the context of data analytics, AI refers to the use of computer systems to perform tasks that typically require human intelligence, such as learning, problem-solving, decision-making, and pattern recognition. AI is not about replacing human analysts but rather augmenting their abilities, enabling them to tackle more complex problems, process larger datasets, and derive deeper insights more efficiently. It's about making data analytics smarter, faster, and more impactful.
AI complements and enhances data analytics in several key ways. It automates repetitive and time-consuming tasks, freeing up human analysts to focus on higher-level strategic thinking and interpretation. AI algorithms can process vast amounts of data at speeds and scales that are simply impossible for humans, uncovering patterns and anomalies that might be missed by traditional methods. Moreover, AI can learn and adapt over time, continuously improving its performance as it is exposed to more data. This adaptability is crucial in dynamic business environments where data patterns are constantly evolving.
The evolution of AI in data analytics is a fascinating journey that spans decades, marked by significant milestones:
- Early Days of Analytics (Pre-AI): Initially, data analysis was largely manual, relying on spreadsheets and basic statistical tools. Analysts spent considerable time on data collection, cleaning, and manual reporting. Insights were often limited to descriptive statistics and simple visualizations.
- Emergence of Machine Learning (ML): The advent of machine learning marked a turning point. ML algorithms allowed computers to learn from data without explicit programming, enabling automated pattern detection and prediction. Early ML techniques like regression and classification started to be applied to data analytics, improving predictive capabilities.
- Deep Learning Revolution: Deep learning, a subset of machine learning using neural networks with multiple layers, has further accelerated AI's impact. Deep learning excels at complex tasks such as image and speech recognition, natural language processing, and handling unstructured data, opening new avenues for data analytics in areas previously difficult to address.
- AI-Powered Analytics Platforms: Today, we see the rise of sophisticated AI-powered analytics platforms that integrate various AI technologies into end-to-end solutions. These platforms offer automated data preparation, advanced analytics capabilities, and user-friendly interfaces, making AI-driven insights accessible to a broader range of business users, not just data scientists.
- Democratization of AI Analytics: The trend is towards democratizing AI analytics, making AI tools and techniques more accessible and user-friendly for non-technical users. This includes the development of AutoML (Automated Machine Learning) tools that simplify model building and deployment, and NLP-based interfaces that allow users to interact with data and analytics using natural language.
This historical context illustrates a clear progression towards more intelligent, automated, and accessible data analytics. AI is not just an incremental improvement; it represents a paradigm shift in how we approach data analysis. It's moving us from a world of primarily descriptive and diagnostic analytics to one where predictive and prescriptive insights are becoming increasingly automated, accurate, and real-time. The next section will delve into the key AI technologies that are at the heart of this transformation, showcasing the specific tools and techniques that are reshaping data analytics as we know it.
3. Key AI Technologies Transforming Data Analytics
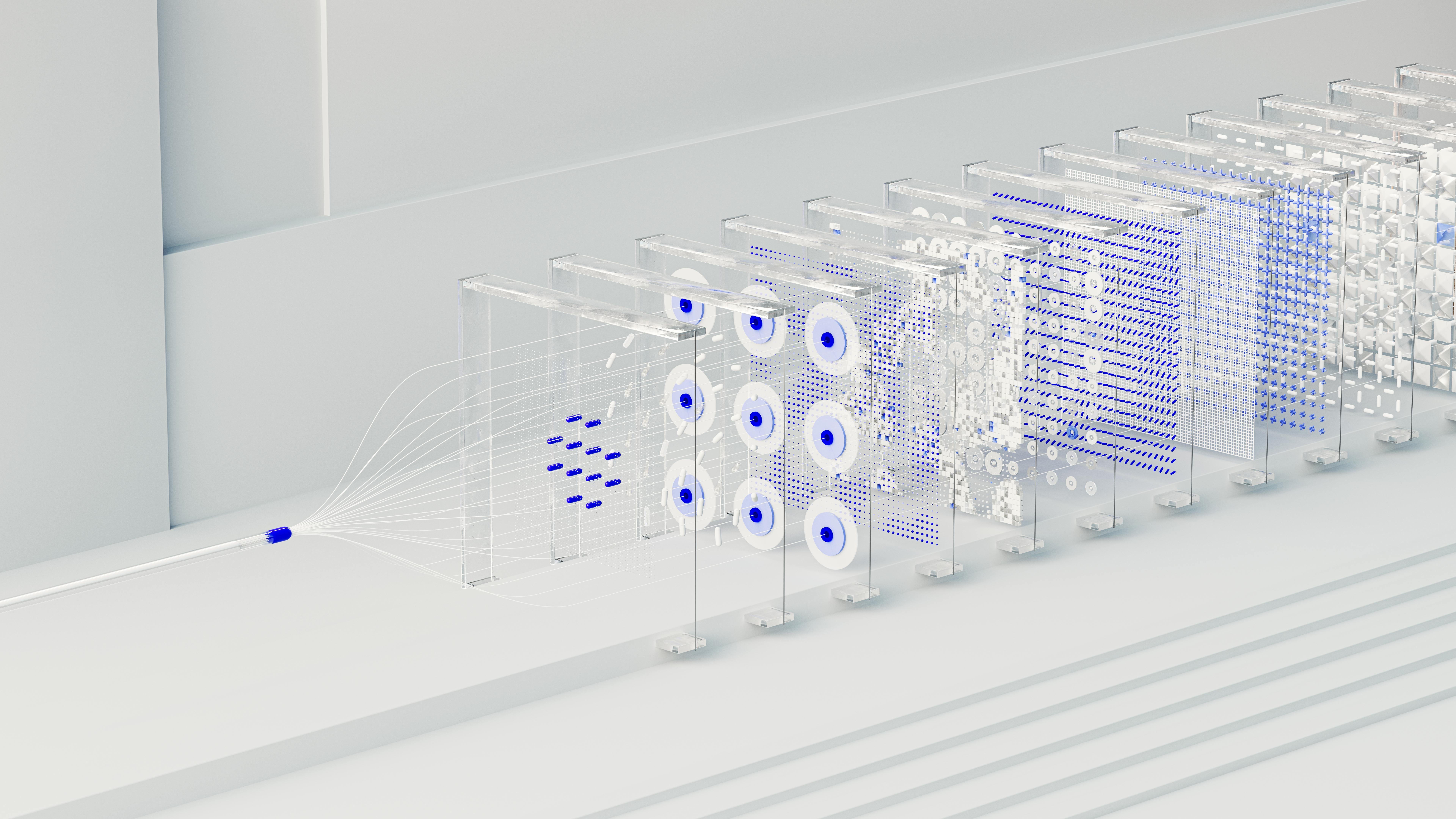
Several core AI technologies are driving the transformation of data analytics. These technologies are not mutually exclusive but often work synergistically to enhance analytical capabilities. Let's explore some of the most impactful AI technologies in data analytics:
-
Machine Learning (ML)
Machine learning is the cornerstone of AI in data analytics. It involves algorithms that allow computer systems to learn from data without being explicitly programmed. ML algorithms can identify patterns, make predictions, and improve their performance over time as they are exposed to more data. In data analytics, ML is used for a wide range of tasks:
- Automated Pattern Detection: ML algorithms can automatically identify complex patterns and relationships within large datasets that would be difficult or impossible for humans to detect manually. For example, in customer behavior analysis, ML can uncover hidden segments and purchasing patterns that inform targeted marketing strategies.
- Anomaly Detection: ML is highly effective in identifying anomalies or outliers in data, which is crucial for fraud detection, quality control, and risk management. For instance, in financial transactions, ML can flag unusual patterns that may indicate fraudulent activity.
- Classification and Regression: ML algorithms are used for classification tasks (categorizing data into predefined classes) and regression tasks (predicting continuous values). In marketing, classification can be used to categorize customers based on their likelihood to churn, while regression can predict customer lifetime value.
- Predictive Modeling: ML powers predictive analytics by building models that forecast future outcomes based on historical data. This includes predicting sales, demand, stock prices, equipment failures, and various other business-critical metrics.
There are various types of machine learning, including supervised learning (where the algorithm is trained on labeled data), unsupervised learning (where the algorithm finds patterns in unlabeled data), and reinforcement learning (where the algorithm learns through trial and error and feedback). Each type has its applications in data analytics, offering a versatile toolkit for different analytical challenges.
-
Natural Language Processing (NLP)
Natural Language Processing (NLP) focuses on enabling computers to understand, interpret, and generate human language. In data analytics, NLP is invaluable for extracting insights from text-based data sources, which are abundant in today's digital world. Key applications of NLP in data analytics include:
- Text Analysis and Sentiment Analysis: NLP techniques can analyze large volumes of text data from customer reviews, social media posts, surveys, and documents to understand customer sentiment, identify key topics, and extract valuable feedback. Sentiment analysis can gauge whether customer opinions are positive, negative, or neutral, providing insights into brand perception and product satisfaction.
- Chatbots and Conversational Analytics: NLP powers chatbots that can interact with users in natural language to answer data-related questions, provide reports, and perform basic data analysis tasks. This makes data access and analytics more user-friendly and accessible to non-technical users.
- Voice Interfaces for Analytics: NLP enables voice-activated analytics, allowing users to query data and generate reports using voice commands. This further democratizes data access and enhances the user experience, especially for quick data checks and on-the-go analysis.
- Document Analysis and Information Extraction: NLP can automatically process and analyze documents such as contracts, reports, and emails to extract key information, identify trends, and automate document-related workflows.
NLP bridges the gap between human language and machine understanding, making unstructured text data a rich source of analytical insights that were previously difficult to tap into.
-
Predictive Analytics (AI-Driven Forecasting)
While predictive analytics existed before AI, AI technologies, particularly machine learning, have significantly enhanced its capabilities and accuracy. AI-driven predictive analytics goes beyond traditional statistical forecasting by leveraging complex algorithms and vast datasets to make more accurate and nuanced predictions about future events. Key areas where AI is enhancing predictive analytics are:
- Advanced Forecasting Models: AI algorithms can build more sophisticated forecasting models that capture non-linear relationships, seasonality, and complex interactions in data, leading to more accurate demand forecasts, sales projections, and financial forecasts.
- Dynamic and Real-time Predictions: AI can enable dynamic predictive models that adapt to changing conditions in real-time. By continuously learning from new data streams, AI models can provide up-to-date predictions that are more responsive to market fluctuations and emerging trends.
- Personalized Predictions: AI allows for personalized predictions at the individual customer level. For example, in marketing, AI can predict the likelihood of each customer making a purchase or churning, enabling highly targeted and personalized interventions.
- Risk Assessment and Early Warning Systems: AI-driven predictive analytics is crucial for risk assessment in various domains, such as finance, supply chain, and cybersecurity. AI can predict potential risks, identify early warning signs of problems, and enable proactive risk mitigation strategies.
AI has elevated predictive analytics from basic forecasting to a powerful tool for anticipating future trends, making proactive decisions, and gaining a competitive edge in dynamic markets.
-
Computer Vision
Computer Vision is an AI field that enables computers to "see" and interpret images and videos. While perhaps less directly associated with traditional data analytics, computer vision is increasingly becoming relevant as visual data becomes more prevalent and valuable for businesses. Applications of computer vision in data analytics include:
- Image and Video Analysis: Computer vision can analyze images and videos to extract meaningful information. In the retail industry, it can be used to analyze customer traffic patterns in stores, monitor shelf inventory levels, and assess product placement effectiveness using video feeds.
- Quality Control and Defect Detection: In manufacturing, computer vision systems can automatically inspect products on assembly lines to detect defects and ensure quality standards. This reduces manual inspection efforts and improves product quality.
- Facial Recognition and Emotion Analysis: Computer vision can be used for facial recognition to identify customers or employees, and for emotion analysis to gauge customer reactions to products or services based on facial expressions captured in videos or images.
- Medical Image Analysis: In healthcare, computer vision is revolutionizing medical image analysis, assisting in the detection of diseases from X-rays, MRIs, and CT scans with increased accuracy and speed.
Computer vision expands the scope of data analytics to include visual data, opening up new possibilities for insights in industries ranging from retail and manufacturing to healthcare and security.
These key AI technologies – Machine Learning, Natural Language Processing, AI-driven Predictive Analytics, and Computer Vision – are at the forefront of transforming data analytics. They empower businesses to process more data, derive deeper insights, automate complex tasks, and make more intelligent decisions. The next section will explore the tangible benefits that these AI-powered capabilities bring to businesses across various sectors.
4. Benefits of AI in Data Analytics for Businesses
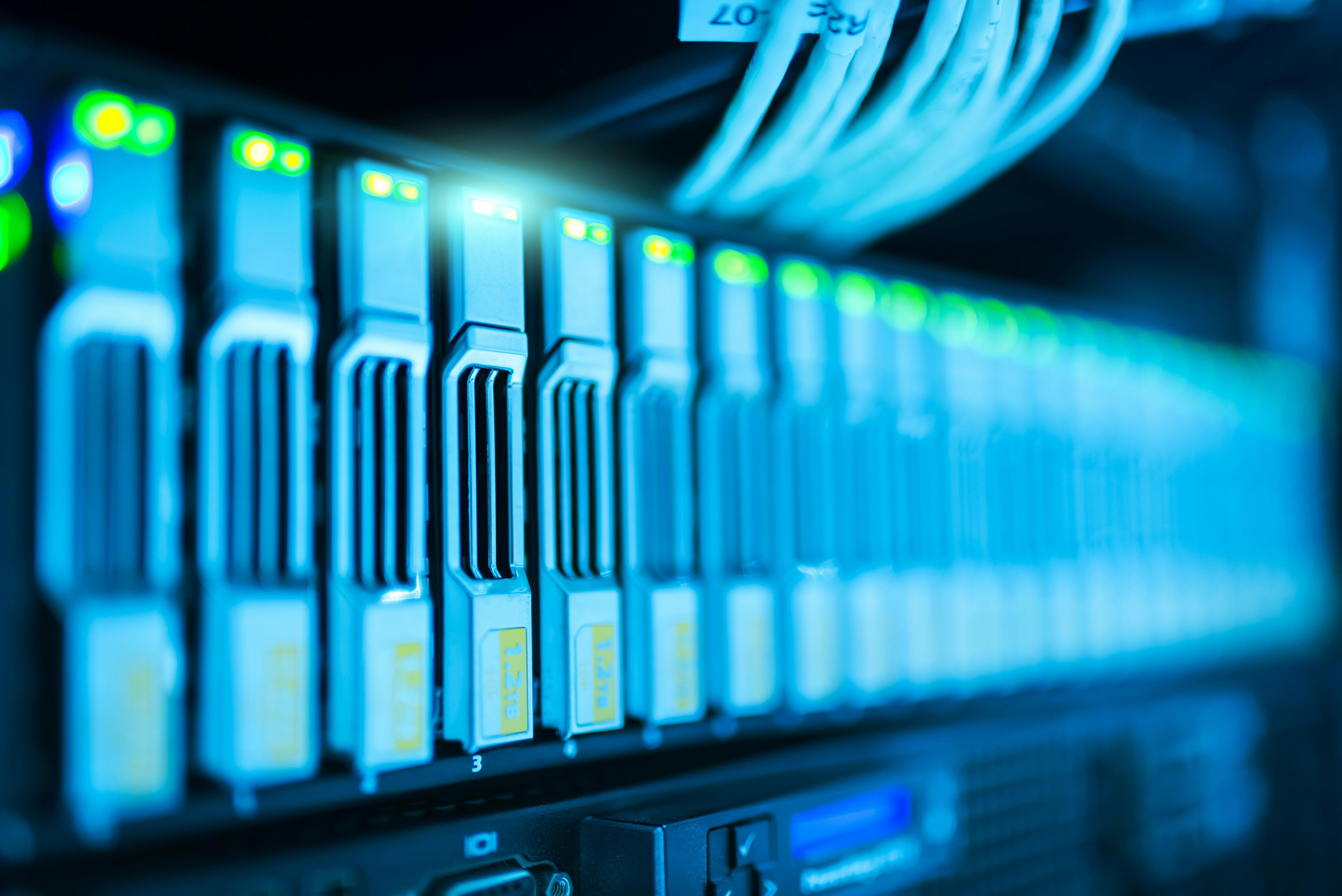
The integration of AI into data analytics brings a multitude of benefits to businesses, enhancing their operational efficiency, strategic decision-making, and overall competitiveness. These benefits are not just incremental improvements but represent a significant leap forward in how organizations can leverage their data assets. Let's explore some of the key advantages:
-
Improved Accuracy and Efficiency in Data Analysis
AI algorithms excel at processing vast amounts of data with speed and precision, significantly improving both the accuracy and efficiency of data analysis. By automating repetitive tasks and complex calculations, AI reduces the risk of human error and accelerates the entire analytics process.
- Automation of Data Preparation: AI can automate data cleaning, preprocessing, and feature engineering, tasks that are traditionally time-consuming and labor-intensive. This frees up data analysts to focus on higher-value activities like insight interpretation and strategic recommendations. For best practices for data cleaning and preparation, explore our resources.
- Faster Insight Generation: AI algorithms can analyze datasets much faster than traditional methods, enabling quicker generation of insights. This speed is crucial in fast-paced business environments where timely information is critical for decision-making.
- Enhanced Accuracy: AI models can identify subtle patterns and anomalies in data that might be missed by human analysts or traditional statistical methods, leading to more accurate insights and predictions.
- Scalability and Handling Big Data: AI is inherently scalable and can handle massive datasets (big data) with ease. This allows businesses to analyze larger and more complex datasets, unlocking insights that were previously inaccessible due to data volume limitations.
For instance, in financial analysis, AI can process millions of transactions in minutes to detect fraudulent activities with higher accuracy than manual review processes, saving time and reducing losses.
-
Real-time Analytics and Decision-Making
AI enables real-time analytics by processing streaming data as it is generated, providing up-to-the-minute insights that facilitate timely decision-making. This is particularly valuable in dynamic environments where immediate responses are crucial.
- Streaming Data Processing: AI algorithms can analyze data streams in real-time, such as website clickstreams, sensor data, and social media feeds, to detect immediate trends and anomalies.
- Dynamic Dashboards and Alerts: AI-powered analytics platforms can create real-time dashboards that visualize key metrics and provide instant alerts when critical thresholds are breached or anomalies are detected.
- Faster Response to Market Changes: Real-time insights enable businesses to react quickly to changing market conditions, customer behaviors, and operational issues. For example, in e-commerce, real-time analytics can track website traffic and sales trends to adjust pricing or marketing campaigns on the fly.
- Proactive Decision-Making: Real-time predictive analytics allows businesses to anticipate future events and make proactive decisions. For example, in supply chain management, real-time predictions of demand fluctuations can enable proactive inventory adjustments to avoid stockouts or overstocking.
In industries like logistics and transportation, real-time analytics powered by AI can optimize routing, predict delays, and improve delivery times based on live traffic and weather data.
-
Enhanced Personalization and Customer Insights
AI significantly enhances personalization and customer insights by analyzing vast amounts of customer data to understand individual preferences, behaviors, and needs at a granular level. This enables businesses to deliver more tailored experiences and build stronger customer relationships.
- Customer Segmentation: AI algorithms can segment customers into more nuanced and behavior-based groups than traditional demographic segmentation. This allows for more targeted marketing campaigns and personalized product recommendations. For a deeper dive, see our guide on customer segmentation with clustering.
- Personalized Product Recommendations: AI-powered recommendation engines analyze customer purchase history, browsing behavior, and preferences to suggest products that are most likely to appeal to individual customers, increasing sales and customer satisfaction.
- Personalized Marketing and Content: AI can personalize marketing messages, email campaigns, and website content based on individual customer profiles, leading to higher engagement rates and conversion rates.
- Improved Customer Service: AI-powered chatbots and virtual assistants can provide personalized customer service, answering queries, resolving issues, and guiding customers through processes in a way that feels tailored to their individual needs.
For example, streaming services use AI to recommend movies and shows based on individual viewing history and preferences, significantly enhancing user engagement and retention.
-
Cost Savings and Operational Efficiency
By automating tasks, optimizing processes, and improving resource allocation, AI in data analytics leads to significant cost savings and operational efficiencies across various business functions.
- Automation of Repetitive Tasks: AI automates many routine data analytics tasks, reducing the need for manual labor and freeing up human resources for more strategic initiatives.
- Process Optimization: AI algorithms can analyze operational data to identify inefficiencies and optimize processes. For example, in manufacturing, AI can optimize production schedules, reduce waste, and improve energy efficiency.
- Predictive Maintenance: In industries with heavy machinery, AI-driven predictive maintenance can forecast equipment failures and schedule maintenance proactively, reducing downtime and maintenance costs.
- Resource Allocation Optimization: AI can analyze data to optimize resource allocation, such as staffing levels, inventory management, and marketing budgets, ensuring resources are used most effectively and efficiently.
In logistics, AI can optimize delivery routes, reduce fuel consumption, and improve vehicle utilization, leading to significant cost savings in transportation operations.
These benefits demonstrate the transformative impact of AI on data analytics. By improving accuracy, enabling real-time insights, enhancing personalization, and driving operational efficiencies, AI empowers businesses to operate smarter, make better decisions, and achieve a competitive edge in today's data-driven world. The next section will explore practical applications of AI in data analytics through real-world case studies across different industries.
5. Practical Applications of AI in Data Analytics
To illustrate the transformative power of AI in data analytics, let's examine practical applications through case studies in three key industries: Retail, Finance, and Healthcare. These examples will showcase how AI is being used to solve real-world business challenges and drive significant improvements.
-
Example 1: Retail - Inventory Management and Personalized Marketing
In the retail industry, AI is revolutionizing inventory management and marketing strategies, enabling retailers to optimize operations and enhance customer engagement.
- Inventory Management:
- Challenge: Traditional inventory management often relies on historical sales data and simple forecasting methods, leading to either stockouts (lost sales) or overstocking (increased holding costs and potential waste).
- AI Solution: AI-driven demand forecasting uses machine learning algorithms to analyze a wide range of data, including historical sales, seasonality, promotions, weather patterns, social media trends, and economic indicators, to predict future demand with greater accuracy.
- Impact: Retailers using AI for inventory management can significantly reduce stockouts, minimize overstocking, optimize storage costs, and improve supply chain efficiency. For example, a major supermarket chain implemented an AI-based demand forecasting system, resulting in a 20% reduction in inventory holding costs and a 15% decrease in stockouts.
- Personalized Marketing:
- Challenge: Generic marketing campaigns often have low engagement and conversion rates. Customers expect personalized experiences and offers tailored to their individual preferences.
- AI Solution: AI-powered personalized marketing uses machine learning and NLP to analyze customer data (purchase history, browsing behavior, demographics, preferences) to create highly targeted marketing campaigns and personalized product recommendations. NLP is used to analyze customer feedback and sentiment to refine marketing messages.
- Impact: Retailers leveraging AI for personalized marketing see significant improvements in customer engagement, conversion rates, and customer loyalty.
- Inventory Management:
-
Example 2: Finance - Fraud Detection and Risk Management
In the finance industry, AI is critical for enhancing fraud detection and risk management, protecting financial institutions and customers from financial crimes and losses.
- Fraud Detection:
- Challenge: Traditional rule-based fraud detection systems are often rigid and can be easily circumvented by sophisticated fraudsters. They also generate high false positive rates, leading to unnecessary customer disruptions.
- AI Solution: AI-driven fraud detection uses machine learning algorithms to analyze transaction data in real-time, identifying anomalous patterns and behaviors that may indicate fraudulent activity. AI models learn from historical fraud cases and adapt to new fraud patterns as they emerge.
- Impact: Financial institutions using AI for fraud detection can significantly reduce fraud losses, improve detection accuracy, and lower false positive rates. A major credit card company implemented an AI-based fraud detection system, resulting in a 40% reduction in fraud losses and a 60% decrease in false positives compared to their previous rule-based system.
- Risk Management:
- Challenge: Traditional risk assessment methods are often static and fail to capture dynamic market conditions and complex risk factors.
- AI Solution: AI-powered risk management uses machine learning to analyze vast datasets from various sources (market data, economic indicators, customer data) to assess and predict financial risks more accurately and dynamically. AI models can assess credit risk, market risk, and operational risk, providing real-time risk scores and early warning signals.
- Impact: Financial institutions using AI for risk management can potentially make more informed lending decisions, optimize capital allocation, and mitigate potential financial losses. A global bank used AI for credit risk assessment, resulting in a 25% improvement in credit risk prediction accuracy and a 15% reduction in loan defaults.
- Fraud Detection:
-
Example 3: Healthcare - Predictive Diagnostics and Patient Care Optimization
In the healthcare industry, AI is transforming diagnostics and patient care, leading to earlier disease detection, personalized treatment plans, and improved patient outcomes.
- Predictive Diagnostics:
- Challenge: Traditional diagnostic processes can be time-consuming and sometimes subjective, leading to delays in diagnosis and treatment.
- AI Solution: AI-powered predictive diagnostics uses computer vision and machine learning to analyze medical images (X-rays, MRIs, CT scans) and patient data (medical history, symptoms, genetic information) to detect diseases earlier and more accurately. AI algorithms can identify subtle anomalies in medical images that may be missed by human eyes.
- Impact: Healthcare providers using AI for predictive diagnostics can improve diagnostic accuracy, accelerate diagnosis, and enable earlier treatment interventions, leading to better patient outcomes. A hospital implemented an AI-based system for detecting breast cancer from mammograms, resulting in a 10% improvement in detection accuracy and a 30% reduction in false negatives.
- Patient Care Optimization:
- Challenge: Delivering personalized and optimized patient care is complex, requiring consideration of numerous factors and individual patient needs.
- AI Solution: AI-driven patient care optimization uses machine learning to analyze patient data to personalize treatment plans, predict patient risks (e.g., readmission risk), and optimize resource allocation in hospitals (e.g., bed management, staffing levels). AI can also monitor patient data in real-time to detect early signs of deterioration and trigger timely interventions.
- Impact: Healthcare organizations using AI for patient care optimization can improve treatment effectiveness, reduce hospital readmissions, enhance patient safety, and optimize operational efficiency. A healthcare system used AI to predict patient readmission risk, resulting in a 20% reduction in readmission rates and improved patient satisfaction scores.
- Predictive Diagnostics:
These examples across Retail, Finance, and Healthcare highlight the diverse and impactful applications of AI in data analytics. AI is not just a theoretical concept but a practical tool that is driving tangible improvements in various industries, enabling businesses to solve complex problems, optimize operations, and deliver better outcomes. The next section will provide a practical guide on how businesses can implement AI in their own data analytics frameworks.
6. Implementing AI in Your Business's Data Analytics
Implementing AI in your business's data analytics framework is a strategic undertaking that requires careful planning, execution, and continuous refinement. It's not just about adopting AI tools but about integrating AI into your organizational culture and processes. Here's a step-by-step guide to help you navigate this journey:
-
Step 1: Identify Specific Business Use Cases
Start by identifying specific business problems or opportunities where AI-powered data analytics can deliver significant value. Focus on areas where data is abundant and insights can lead to measurable improvements. Examples include:
- Improving customer churn prediction
- Optimizing pricing strategies
- Enhancing fraud detection
- Personalizing marketing campaigns
- Streamlining supply chain operations
- Predicting equipment failures
Prioritize use cases that align with your business objectives and have a clear ROI. Starting with a few well-defined use cases allows for focused effort and demonstrable success, building momentum for broader AI adoption.
-
Step 2: Assess Your Data Readiness
AI algorithms are data-hungry. Assess the quality, quantity, and accessibility of your data. Key considerations include:
- Data Quality: Is your data accurate, complete, consistent, and reliable? AI models are only as good as the data they are trained on. Invest in data cleaning and quality improvement processes. For data cleaning and preparation best practices, see our guide.
- Data Quantity: Do you have enough data to train robust AI models? Complex AI models, especially deep learning models, require large datasets.
- Data Variety: Can you leverage different types of data (structured, unstructured, internal, external) relevant to your use cases?
- Data Accessibility: Is your data easily accessible and integrated? Data silos can hinder AI initiatives. Ensure data is accessible and can be integrated from various sources.
If your data readiness is low, prioritize data improvement initiatives before fully embarking on AI implementation.
-
Step 3: Choose the Right AI Tools and Platforms
Select AI tools and platforms that align with your business needs, technical capabilities, and budget. Options range from cloud-based AI services to specialized AI analytics platforms and open-source tools. Consider:
- Cloud AI Platforms: Cloud providers like AWS, Azure, and Google Cloud offer comprehensive AI services, including machine learning, NLP, computer vision, and AutoML tools. These platforms are scalable, flexible, and often provide user-friendly interfaces.
- Specialized AI Analytics Platforms: Several vendors offer platforms specifically designed for AI-powered data analytics, integrating various AI technologies into end-to-end solutions.
- Open-Source Tools: Open-source libraries like TensorFlow, PyTorch, scikit-learn, and NLTK provide powerful tools for building custom AI models. These require in-house expertise in data science and AI development.
- Ease of Integration: Ensure the chosen tools can integrate seamlessly with your existing data infrastructure, analytics tools, and business systems.
Start with tools that are user-friendly and require less specialized expertise if you are new to AI, and gradually explore more advanced options as your capabilities grow.
-
Step 4: Build an AI-Ready Data Infrastructure
An AI-ready data infrastructure is crucial for successful AI implementation. This includes:
- Scalable Data Storage: Implement scalable data storage solutions (e.g., data lakes, cloud storage) to handle large volumes of data required for AI.
- Efficient Data Pipelines: Establish robust data pipelines for data ingestion, processing, and transformation to ensure data flows smoothly to AI models.
- Data Governance and Security: Implement strong data governance policies and security measures to ensure data quality, compliance, and privacy, especially when dealing with sensitive data.
- Compute Infrastructure: Ensure you have adequate compute infrastructure (e.g., cloud computing, GPUs) to support the computational demands of AI model training and deployment.
Consider leveraging cloud-based data infrastructure for scalability and flexibility.
-
Step 5: Develop AI Talent and Skills
Successful AI implementation requires talent with data science, AI, and domain expertise. Consider:
- Hiring Data Scientists and AI Engineers: Recruit professionals with expertise in machine learning, NLP, computer vision, and data analytics.
- Training Existing Staff: Upskill your existing data analysts and IT staff in AI technologies and tools. Provide training on AI concepts, tools, and best practices.
- Partnerships and Consulting: Consider partnering with AI consulting firms or academic institutions to access specialized expertise and support, especially in the initial stages of AI adoption.
- Foster a Data-Driven Culture: Promote a culture of data literacy and encourage collaboration between business users and data science teams.
Building in-house AI expertise over time is crucial for long-term success with AI in data analytics.
-
Step 6: Start with Pilot Projects and Iterate
Begin with small-scale pilot projects to test AI solutions, validate their effectiveness, and learn from early experiences. Adopt an iterative approach:
- Pilot Projects: Select a few high-priority use cases for pilot projects. Focus on demonstrating quick wins and tangible value.
- Agile Development: Use agile methodologies for AI development, with short development cycles, frequent testing, and continuous feedback.
- Measure and Evaluate: Rigorously measure the performance and impact of AI solutions. Track key metrics and KPIs to assess ROI and identify areas for improvement.
- Iterate and Refine: Continuously iterate and refine AI models and solutions based on performance data and feedback. AI models require ongoing monitoring and retraining to maintain accuracy and relevance.
Pilot projects provide valuable learning opportunities and help build confidence in AI before scaling up initiatives.
-
Step 7: Address Ethical and Privacy Considerations
Implementing AI responsibly requires careful consideration of ethical and privacy implications:
- Data Privacy: Comply with data privacy regulations (e.g., GDPR, CCPA) and implement robust data anonymization and security measures to protect sensitive data.
- Algorithm Bias: Be aware of potential biases in AI algorithms and data, which can lead to unfair or discriminatory outcomes. Implement bias detection and mitigation techniques.
- Transparency and Explainability: Strive for transparency in AI models and decision-making processes. Use explainable AI (XAI) techniques to understand and explain AI model outputs, especially in critical applications.
- Ethical Guidelines: Develop and adhere to ethical guidelines for AI development and deployment, ensuring AI is used responsibly and for the benefit of society.
Ethical and privacy considerations should be integral to your AI implementation strategy from the outset.
By following these steps, businesses can strategically implement AI in their data analytics frameworks, unlocking the transformative potential of AI to drive innovation, efficiency, and competitive advantage. The next section will address some of the key challenges and considerations that businesses may encounter during AI implementation.
7. Challenges and Considerations
While the benefits of AI in data analytics are substantial, businesses must also be aware of the challenges and considerations that come with AI implementation. Addressing these proactively is crucial for successful AI adoption. Let's explore some key challenges and considerations:
-
Data Privacy and Ethical Considerations in AI
AI systems often rely on large volumes of data, which may include sensitive personal information. Data privacy and ethical considerations are paramount:
- Compliance with Data Privacy Regulations: Businesses must comply with data privacy regulations like GDPR, CCPA, and others, which impose strict rules on the collection, processing, and storage of personal data. AI systems must be designed to adhere to these regulations.
- Data Security: Protecting data from unauthorized access, breaches, and cyber threats is crucial. Robust data security measures, including encryption, access controls, and security monitoring, must be implemented.
- Ethical Use of AI: Ensure AI is used ethically and responsibly. Avoid using AI in ways that could discriminate against individuals or groups, infringe on privacy rights, or have unintended negative consequences. Develop ethical AI guidelines and governance frameworks.
- Transparency and Consent: Be transparent with users about how their data is being used by AI systems. Obtain informed consent when necessary, and provide users with control over their data.
Addressing data privacy and ethical concerns proactively builds trust and ensures responsible AI adoption.
-
The Need for Skilled Personnel and Training
Implementing and managing AI systems requires skilled personnel with expertise in data science, AI, and related fields. The shortage of AI talent is a significant challenge:
- Recruiting AI Talent: Hiring experienced data scientists, AI engineers, and machine learning specialists can be challenging and competitive. Develop effective recruitment strategies and competitive compensation packages.
- Upskilling Existing Staff: Invest in training and upskilling your existing data analysts and IT staff in AI technologies. Provide comprehensive training programs and learning resources.
- Building In-House Expertise: Focus on building in-house AI expertise over time, rather than relying solely on external consultants. This ensures long-term sustainability and knowledge retention.
- Data Literacy for Business Users: Promote data literacy across the organization, so business users can understand and effectively utilize AI-driven insights. Provide training and resources to enhance data literacy.
Investing in talent development and training is essential for building a capable AI-driven organization.
-
Potential Biases in AI Algorithms and How to Address Them
AI algorithms can inadvertently learn biases from training data, leading to unfair or discriminatory outcomes. Addressing bias is crucial for responsible AI:
- Data Bias: Training data may contain biases reflecting societal or historical inequalities. Identify and mitigate data biases through data preprocessing, data augmentation, and diverse data collection strategies.
- Algorithm Bias: AI algorithms themselves can introduce biases. Use bias detection and mitigation techniques during model development and training. Regularly audit AI models for bias.
- Fairness Metrics: Define and use fairness metrics to evaluate AI model outputs for potential biases across different groups.
- Explainable AI (XAI): Use XAI techniques to understand how AI models make decisions and identify potential sources of bias in model logic.
Proactive bias detection and mitigation are essential for ensuring fairness and equity in AI applications.
-
Integration Complexity and Legacy Systems
Integrating AI into existing data analytics frameworks and legacy systems can be complex and challenging:
- System Compatibility: Ensure AI tools and platforms are compatible with your existing IT infrastructure, data systems, and applications.
- Data Integration Challenges: Integrating data from disparate sources and data silos can be complex. Implement robust data integration strategies and tools.
- Legacy System Modernization: Legacy systems may not be easily adaptable to AI. Consider modernizing legacy systems or developing integration layers to bridge the gap.
- Change Management: Implementing AI often requires significant changes in organizational processes, workflows, and roles. Effective change management strategies are crucial for smooth AI adoption.
Careful planning and a phased approach to integration can help mitigate these complexities.
Addressing these challenges and considerations thoughtfully will pave the way for more successful, responsible, and impactful AI implementation in data analytics. The next section will look towards the future of AI in data analytics, exploring emerging trends and the evolving role of AI.
8. The Future of AI in Data Analytics
The future of AI in data analytics is dynamic and promising, with continuous advancements pushing the boundaries of what's possible. Emerging trends and evolving roles of AI point towards even more transformative capabilities. Let's explore some key aspects of the future landscape:
-
Emerging Trends and Technologies to Watch
Several emerging trends and technologies are set to shape the future of AI in data analytics:
- Automated Machine Learning (AutoML): AutoML is making AI more accessible to non-experts by automating many steps in the machine learning pipeline, such as data preprocessing, feature selection, model selection, and hyperparameter tuning. AutoML tools will democratize AI and empower business users to build and deploy AI models more easily.
- Explainable AI (XAI): As AI becomes more pervasive, the need for transparency and explainability is growing. XAI techniques aim to make AI model decisions more understandable to humans, enhancing trust and accountability. XAI will be crucial for applications in regulated industries and critical decision-making scenarios.
- AI-Driven Data Governance: AI is being applied to automate and enhance data governance processes, including data quality monitoring, data lineage tracking, data cataloging, and compliance management. AI-driven data governance will improve data quality, reduce governance costs, and ensure data compliance more effectively.
- Edge AI Analytics: Edge AI involves processing data closer to the source of data generation, such as IoT devices, edge servers, and mobile devices. Edge AI analytics reduces latency, bandwidth usage, and privacy risks by processing data locally, enabling real-time insights and actions at the edge.
- Generative AI for Data Analytics: Generative AI models, like Generative Adversarial Networks (GANs) and transformers, are being explored for data augmentation, synthetic data generation, and creating more realistic simulations for analytics. Generative AI can enhance data quality and expand the scope of data analytics.
- Quantum Computing for AI: Quantum computing, while still in early stages, has the potential to revolutionize AI by solving complex optimization problems and accelerating machine learning algorithms significantly. Quantum AI could unlock new levels of performance and capabilities in data analytics in the future.
Staying abreast of these emerging trends will be crucial for businesses to leverage the full potential of AI in data analytics.
-
The Evolving Role of AI in Business Decision-Making
AI's role in business decision-making is evolving from automation and augmentation to becoming a strategic partner:
- From Automation to Augmentation: Initially, AI was primarily seen as a tool for automating repetitive tasks and augmenting human capabilities. AI will increasingly become a strategic partner, providing deeper insights, predictive intelligence, and prescriptive recommendations to guide strategic decisions.
- AI as a Decision Support System: AI will act as a sophisticated decision support system, providing business leaders with comprehensive data-driven insights, scenario analysis, and risk assessments to inform strategic choices.
- AI-Driven Strategic Planning: AI will play a more prominent role in strategic planning, helping businesses identify new market opportunities, assess competitive landscapes, and formulate long-term strategies based on predictive intelligence and data simulations.
- Human-AI Collaboration: The future of decision-making will be characterized by closer human-AI collaboration, where humans bring domain expertise, creativity, and ethical judgment, while AI provides data processing power, analytical capabilities, and predictive insights.
AI is poised to become an indispensable strategic asset, transforming how businesses make decisions at all levels.
-
How Businesses Can Prepare for Future Advancements in AI
To prepare for future advancements in AI, businesses should take proactive steps:
- Continuous Learning and Experimentation: Foster a culture of continuous learning and experimentation with new AI technologies and techniques. Encourage employees to stay updated with AI trends and experiment with emerging tools.
- Building Adaptable Data Infrastructure: Invest in adaptable and scalable data infrastructure that can accommodate future AI advancements. Cloud-based infrastructure and data lakes offer flexibility and scalability.
- Developing AI Ethics and Governance Frameworks: Establish robust AI ethics and governance frameworks to guide responsible AI development and deployment. Integrate ethical considerations into AI strategy and operations.
- Talent Development and Lifelong Learning: Invest in talent development and promote lifelong learning in AI skills. Encourage employees to pursue continuous learning and skill enhancement in AI and data analytics.
- Strategic Partnerships and Ecosystem Building: Build strategic partnerships with AI technology providers, research institutions, and industry peers to stay at the forefront of AI innovation and collaborate on AI initiatives.
By taking these preparatory steps, businesses can position themselves to effectively leverage future advancements in AI and maintain a competitive edge in the evolving landscape of data analytics.
The future of AI in data analytics is bright, filled with opportunities for innovation, efficiency, and transformative impact. By embracing emerging trends, adapting to evolving roles, and preparing proactively, businesses can harness the full power of AI to drive data-driven success.
Conclusion
Artificial Intelligence is no longer a futuristic concept—it is the present and future of data analytics. As we have explored in this guide, AI is fundamentally transforming data analytics, moving it beyond traditional methods to unlock unprecedented levels of accuracy, efficiency, and insight. From automating complex tasks to enabling real-time decision-making and personalized experiences, AI is empowering businesses to harness the full potential of their data assets.
Key AI technologies like Machine Learning, Natural Language Processing, AI-driven Predictive Analytics, and Computer Vision are driving this transformation, each bringing unique capabilities to the analytical landscape. Machine learning automates pattern detection and prediction, NLP unlocks insights from text data, AI-driven predictive analytics enhances forecasting accuracy, and computer vision extends analytics to visual data. These technologies, working synergistically, are enabling businesses to solve complex problems, optimize operations, and create new opportunities.
The benefits of AI in data analytics are tangible and impactful, ranging from improved accuracy and efficiency to enhanced personalization, real-time insights, and significant cost savings. Case studies across Retail, Finance, and Healthcare demonstrate the practical applications and real-world value that AI brings to diverse industries. Implementing AI in your business requires a strategic approach, focusing on identifying use cases, assessing data readiness, choosing the right tools, building an AI-ready infrastructure, developing talent, and adopting an iterative approach.
While the journey of AI adoption comes with challenges and considerations—including data privacy, ethical concerns, talent gaps, and integration complexities—these can be addressed proactively with careful planning, responsible practices, and a commitment to continuous learning. The future of AI in data analytics is marked by exciting emerging trends like AutoML, XAI, Edge AI, and AI-driven data governance, promising even more powerful and accessible AI capabilities. As AI evolves from automation to augmentation and strategic partnership, businesses that embrace AI and prepare for future advancements will gain a significant competitive advantage in the data-driven era.
In conclusion, AI is not just enhancing data analytics—it is redefining it. By embracing AI, businesses can unlock deeper insights, make smarter decisions, operate more efficiently, and create more personalized experiences for their customers. The time to embrace AI in data analytics is now. By taking strategic steps, addressing challenges responsibly, and staying informed about future trends, your organization can harness the transformative power of AI to drive data-driven success and innovation.
What next?
Ready to embark on your AI in data analytics journey? Explore the resources available on our website to equip your teams with the knowledge and tools they need. Share this guide with your colleagues and leadership to spark conversations and foster a culture of AI innovation within your organization.
Remember, the path to AI-driven data analytics is a journey of continuous learning and adaptation. Start by identifying a pilot project, assess your data readiness, and take the first steps towards implementing AI in your business. Every step you take will move your organization closer to unlocking the transformative potential of AI and achieving data-driven excellence.
Let's collaborate to harness the power of AI for your data analytics needs. Embrace these insights, champion AI innovation, and watch as AI-powered data analytics propels your business towards greater efficiency, innovation, and strategic advantage.
References and Further Reading
Below is a curated list of resources for those eager to deepen their understanding of AI in data analytics, machine learning, and related topics:
- Goodfellow, I., Bengio, Y., & Courville, A. (2016). Deep Learning. MIT Press.
- Russell, S. J., & Norvig, P. (2020). Artificial Intelligence: A Modern Approach (4th ed.). Pearson.
- Provost, F., & Fawcett, T. (2013). Data Science for Business: What You Need to Know about Data Mining and Data-Analytic Thinking. O'Reilly Media.